DIAMANT: Dual Image-Attention Map Encoders For Medical Image Segmentation
CoRR(2023)
摘要
Although purely transformer-based architectures showed promising performance in many computer vision tasks, many hybrid models consisting of CNN and transformer blocks are introduced to fit more specialized tasks. Nevertheless, despite the performance gain of both pure and hybrid transformer-based architectures compared to CNNs in medical imaging segmentation, their high training cost and complexity make it challenging to use them in real scenarios. In this work, we propose simple architectures based on purely convolutional layers, and show that by just taking advantage of the attention map visualizations obtained from a self-supervised pretrained vision transformer network (e.g., DINO) one can outperform complex transformer-based networks with much less computation costs. The proposed architecture is composed of two encoder branches with the original image as input in one branch and the attention map visualizations of the same image from multiple self-attention heads from a pre-trained DINO model (as multiple channels) in the other branch. The results of our experiments on two publicly available medical imaging datasets show that the proposed pipeline outperforms U-Net and the state-of-the-art medical image segmentation models.
更多查看译文
关键词
medical image-attention,segmentation
AI 理解论文
溯源树
样例
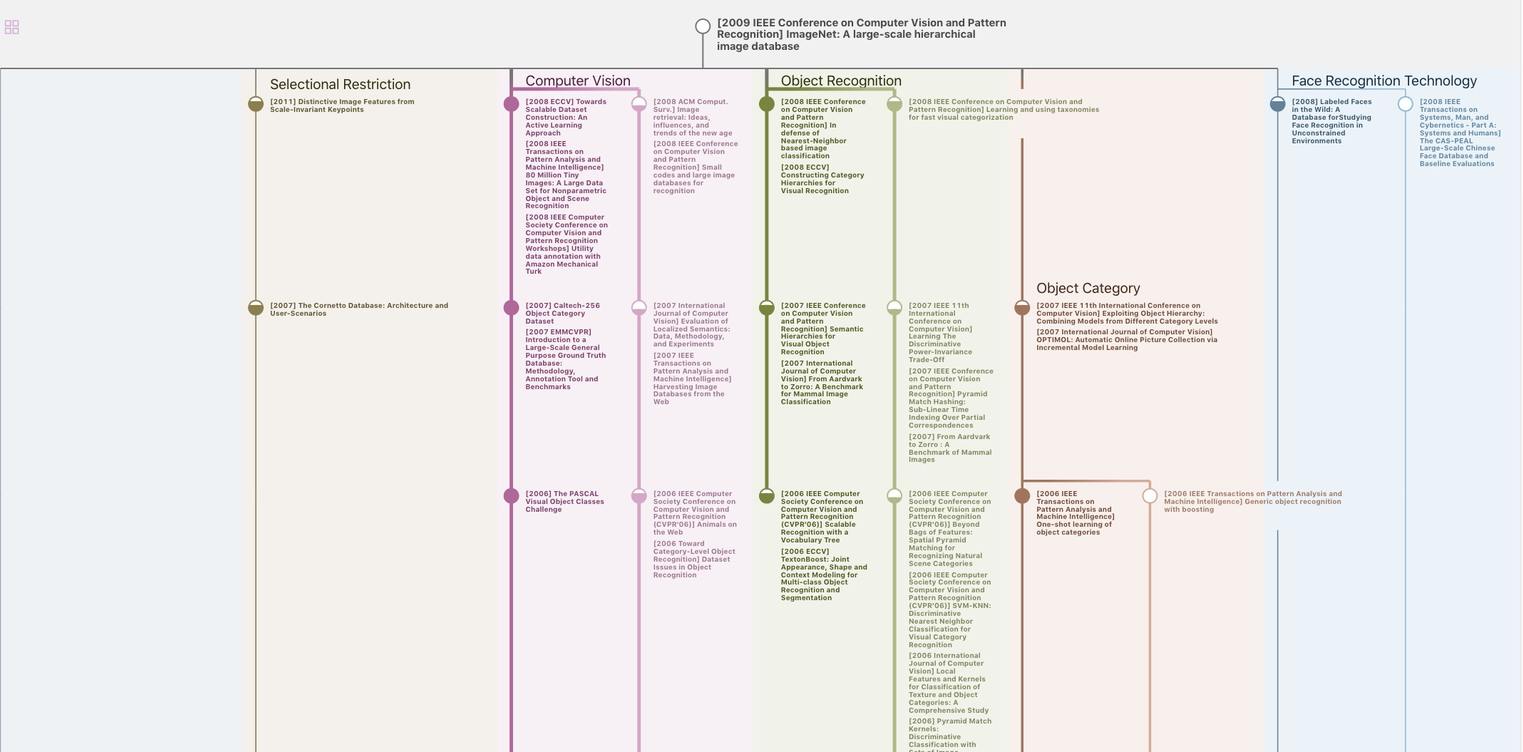
生成溯源树,研究论文发展脉络
Chat Paper
正在生成论文摘要