Neural Implicit Dense Semantic SLAM
CoRR(2023)
摘要
This paper presents an efficient online framework to solve the well-known semantic Visual Simultaneous Localization and Mapping (V-SLAM) problem for indoor scenes leveraging the advantages of neural implicit scene representation. Existing methods on similar lines, such as NICE-SLAM, has some critical practical limitations to put to use for such an important indoor scene understanding problem. To this end, we contend for the following proposition for modern semantic V-SLAM contrary to existing methods assuming RGB-D frames as input (i) For a rigid scene, robust and accurate camera motion could be computed with disentangled tracking and 3D mapping pipeline. (ii) Using neural fields, a dense and multifaceted scene representation of SDF, semantics, RGB, and depth is provided memory efficiently. (iii) Rather than using every frame, we demonstrate that the set of keyframes is sufficient to learn excellent scene representation, thereby improving the pipeline's train time. (iv) Multiple local mapping networks could be used to extend the pipeline for large-scale scenes. We show via extensive experiments on several popular benchmark datasets that our approach offers accurate tracking, mapping, and semantic labeling at test time even with noisy and highly sparse depth measurements. Later in the paper, we show that our pipeline can easily extend to RGB image input. Overall, the proposed pipeline offers a favorable solution to an important scene understanding task that can assist in diverse robot visual perception and related problems.
更多查看译文
AI 理解论文
溯源树
样例
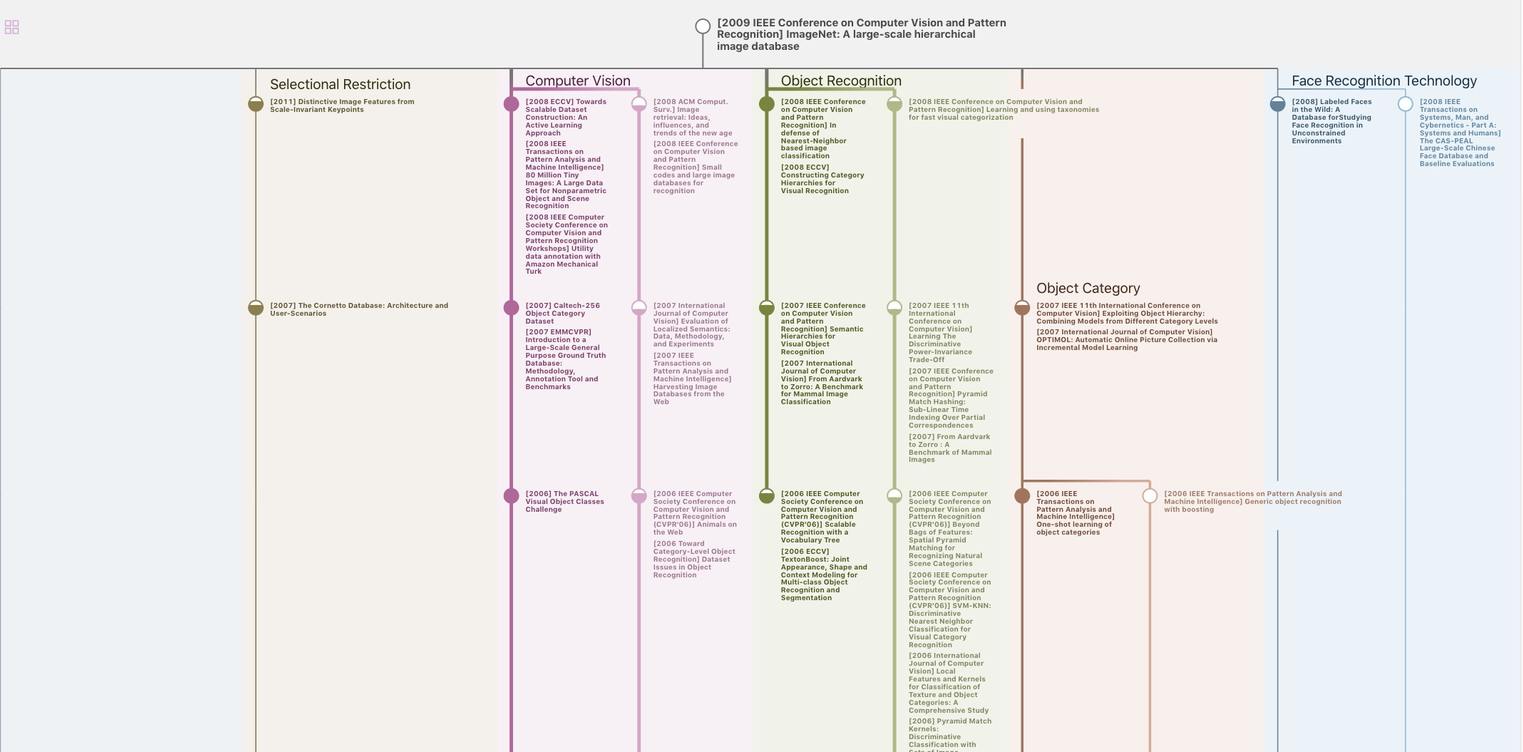
生成溯源树,研究论文发展脉络
Chat Paper
正在生成论文摘要