Perspectively Equivariant Keypoint Learning for Omnidirectional Images
IEEE transactions on image processing : a publication of the IEEE Signal Processing Society(2023)
摘要
Robust keypoint detection on omnidirectional images against large perspective variations, is a key problem in many computer vision tasks. In this paper, we propose a perspectively equivariant keypoint learning framework named OmniKL for addressing this problem. Specifically, the framework is composed of a perspective module and a spherical module, each one including a keypoint detector specific to the type of the input image and a shared descriptor providing uniform description for omnidirectional and perspective images. In these detectors, we propose a differentiable candidate position sorting operation for localizing keypoints, which directly sorts the scores of the candidate positions in a differentiable manner and returns the globally top-K keypoints on the image. This approach does not break the differentiability of the two modules, thus they are end-to-end trainable. Moreover, we design a novel training strategy combining the self-supervised and co-supervised methods to train the framework without any labeled data. Extensive experiments on synthetic and real-world 360° image datasets demonstrate the effectiveness of OmniKL in detecting perspectively equivariant keypoints on omnidirectional images. Our source code are available online at
https://github.com/vandeppce/sphkpt
.
更多查看译文
关键词
equivariant keypoint learning,images
AI 理解论文
溯源树
样例
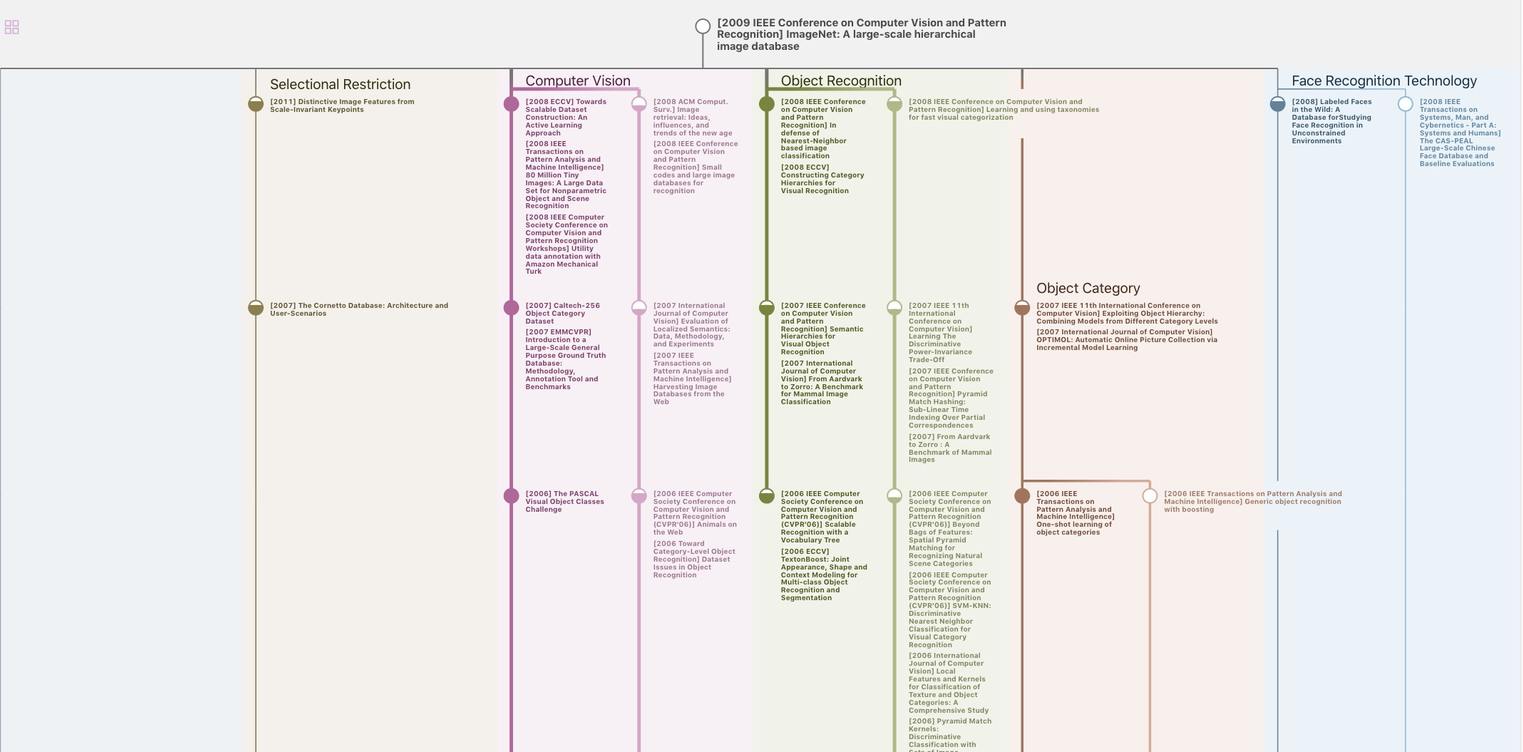
生成溯源树,研究论文发展脉络
Chat Paper
正在生成论文摘要