Apply Graph Signal Processing on NILM: An Unsupervised Approach Featuring Power Sequences.
Sensors (Basel, Switzerland)(2023)
摘要
As a low-cost demand-side management application, non-intrusive load monitoring (NILM) offers feedback on appliance-level electricity usage without extra sensors. NILM is defined as disaggregating loads only from aggregate power measurements through analytical tools. Although low-rate NILM tasks have been conducted by unsupervised approaches based on graph signal processing (GSP) concepts, enhancing feature selection can still contribute to performance improvement. Therefore, a novel unsupervised GSP-based NILM approach with power sequence feature (STS-UGSP) is proposed in this paper. First, state transition sequences (STS) are extracted from power readings and featured in clustering and matching, instead of power changes and steady-state power sequences featured in other GSP-based NILM works. When generating graph in clustering, dynamic time warping distances between STSs are calculated for similarity quantification. After clustering, a forward-backward power STS matching algorithm is proposed for searching each STS pair of an operational cycle, utilizing both power and time information. Finally, load disaggregation results are obtained based on STS clustering and matching results. STS-UGSP is validated on three publicly accessible datasets from various regions, generally outperforming four benchmarks in two evaluation metrics. Besides, STS-UGSP estimates closer energy consumption of appliances to the ground truth than benchmarks.
更多查看译文
关键词
dynamic time warping,graph signal processing,non–intrusive load monitoring,state transition sequences
AI 理解论文
溯源树
样例
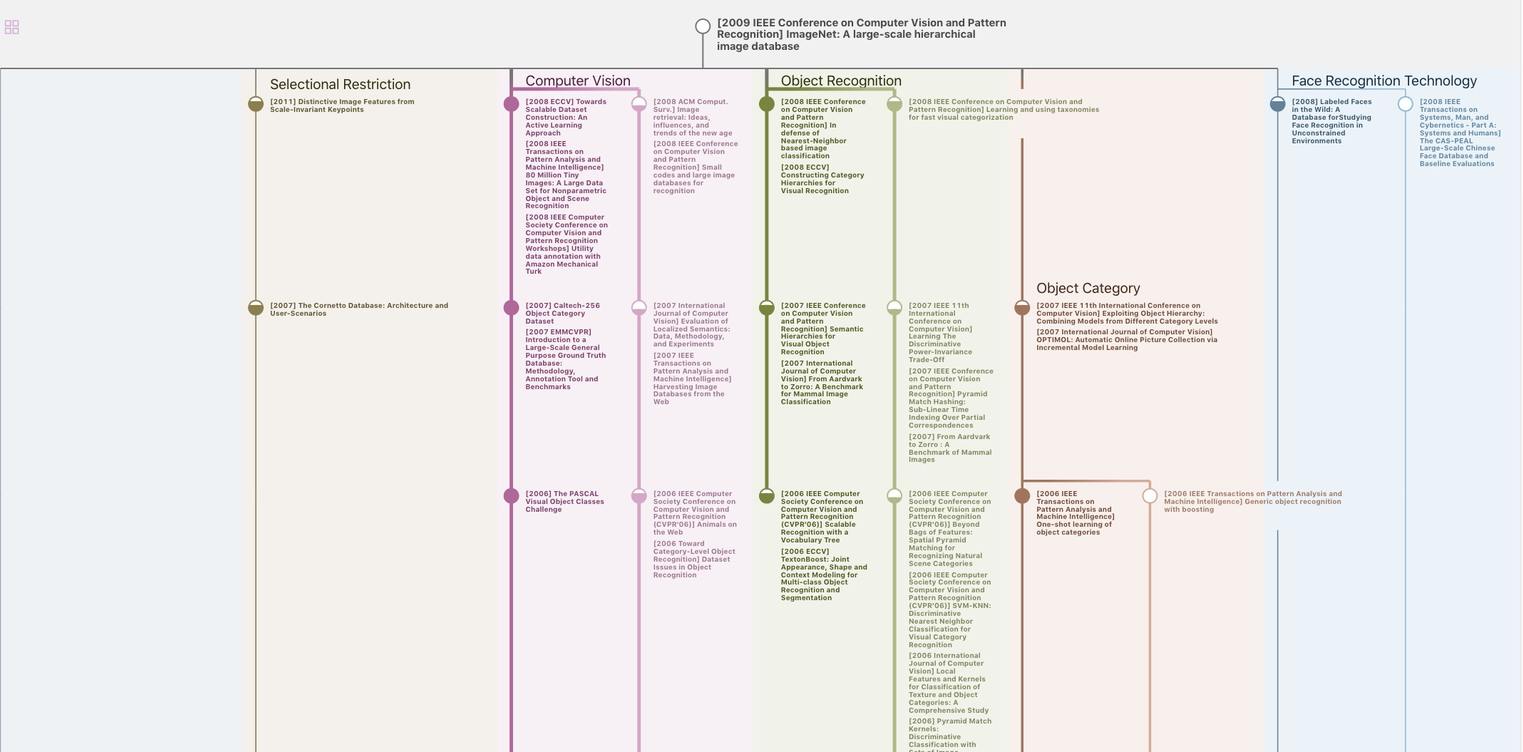
生成溯源树,研究论文发展脉络
Chat Paper
正在生成论文摘要