Methods of Machine Learning-Based Chimeric Antigen Receptor Immunological Synapse Quality Quantification.
Methods in molecular biology (Clifton, N.J.)(2023)
摘要
Chimeric Antigen Receptor (CAR)-mediated immunotherapy shows promising results for refractory blood cancers. Currently, six CAR-T drugs have been approved by U.S. Food and Drug Administration (FDA). Theoretically, CAR-T cells must form an effective immunological synapse (IS, an interface between effective cells and their target cells) with their susceptible tumor cells to eliminate tumor cells. Previous studies show that CAR IS quality can be used as a predictive functional biomarker for CAR-T immunotherapies. However, quantification of CAR-T IS quality is clinically challenging. Machine learning (ML)-based CAR-T IS quality quantification has been proposed previously.Here, we show an easy-to-use, step-by-step approach to predicting the efficacy of CAR-modified cells using ML-based CAR IS quality quantification. This approach will guide the users on how to use ML-based CAR IS quality quantification in detail, which include: how to image CAR IS on the glass-supported planar lipid bilayer, how to define the CAR IS focal plane, how to segment the CAR IS images, and how to quantify the IS quality using ML-based algorithms.This approach will significantly enhance the accuracy and proficiency of CAR IS prediction in research.
更多查看译文
关键词
Artificial neural networks (ANN),Chimeric antigen receptor (CAR),Confocal microscopy,IS,Immune synapse,Immunological synapse,SLB,glass-supported lipid bilayer
AI 理解论文
溯源树
样例
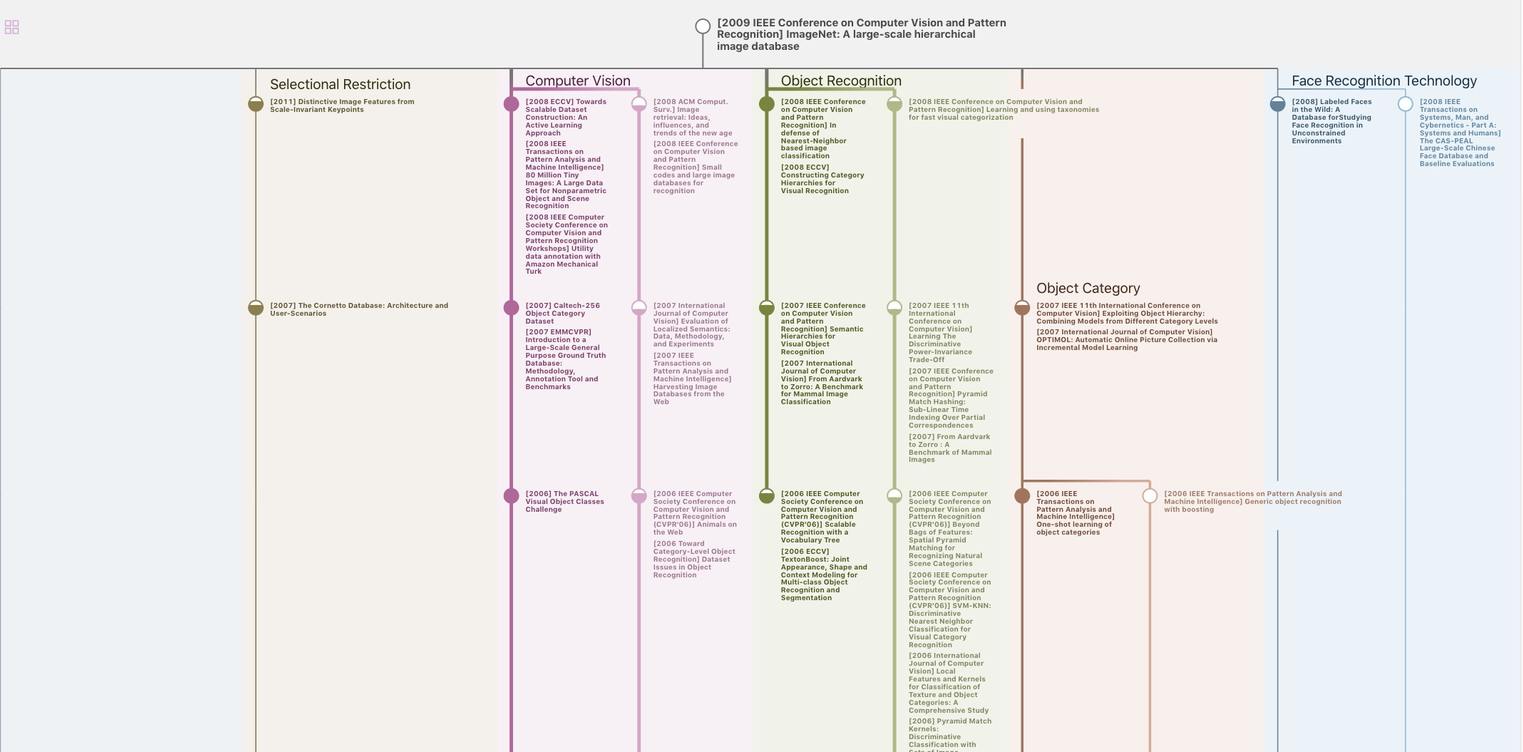
生成溯源树,研究论文发展脉络
Chat Paper
正在生成论文摘要