Lane Change Strategies for Autonomous Vehicles: A Deep Reinforcement Learning Approach Based on Transformer
IEEE Transactions on Intelligent Vehicles(2023)
Abstract
End-to-end approaches are one of the most promising solutions for autonomous vehicles (AVs) decision-making. However, the deployment of these technologies is usually constrained by the high computational burden. To alleviate this problem, we proposed a lightweight transformer-based end-to-end model with risk awareness ability for AV decision-making. Specifically, a lightweight network with depth-wise separable convolution and transformer modules was firstly proposed for image semantic extraction from time sequences of trajectory data. Then, we assessed driving risk by a probabilistic model with position uncertainty. This model was integrated into deep reinforcement learning (DRL) to find strategies with minimum expected risk. Finally, the proposed method was evaluated in three lane change scenarios to validate its superiority.
MoreTranslated text
Key words
Autonomous vehicles,Decision making,Computational modeling,Transformers,Computer architecture,Uncertainty,Reinforcement learning,decision-making,reinforcement learning,lane change,transformer
AI Read Science
Must-Reading Tree
Example
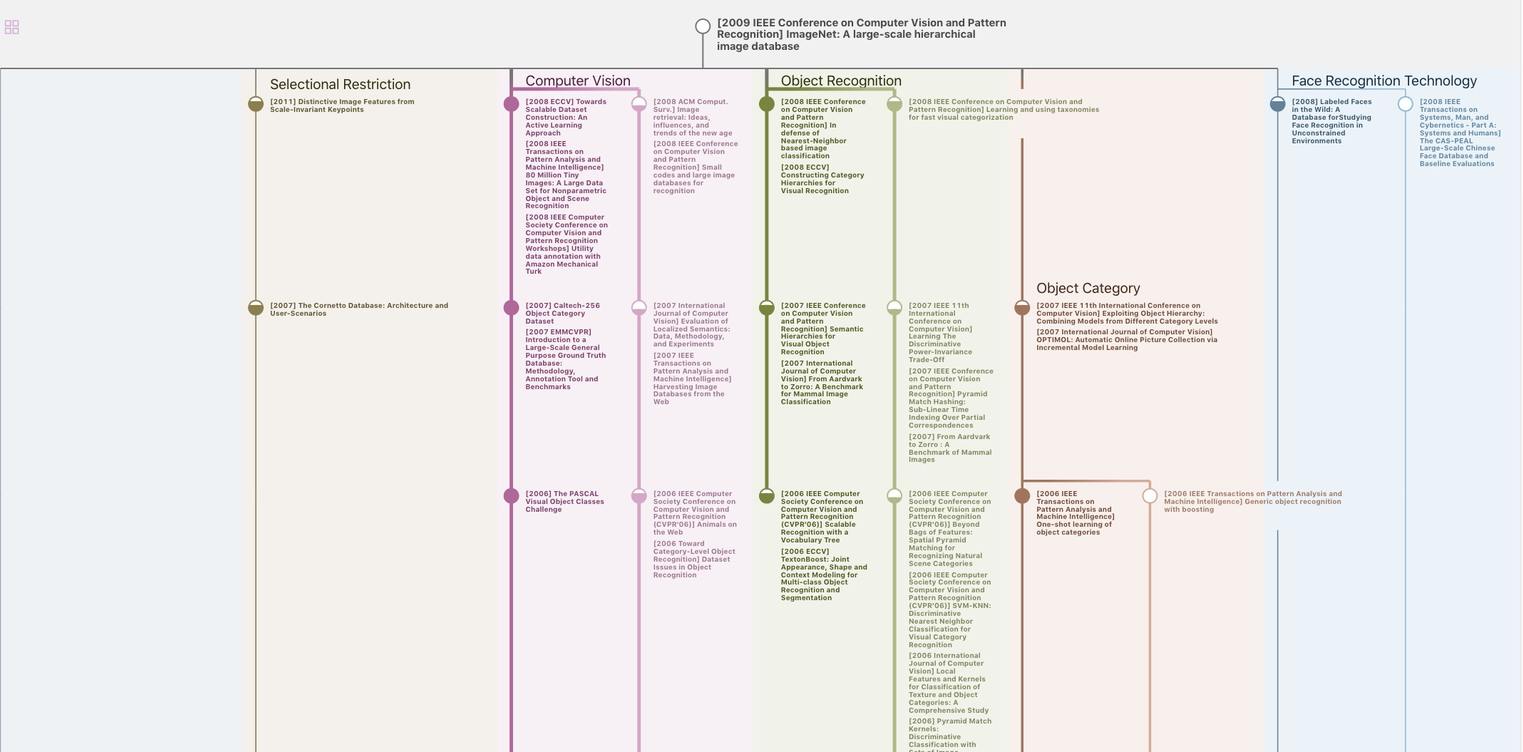
Generate MRT to find the research sequence of this paper
Chat Paper
Summary is being generated by the instructions you defined