Implementation of AI image reconstruction in CT-how is it validated and what dose reductions can be achieved
BRITISH JOURNAL OF RADIOLOGY(2023)
摘要
CT reconstruction has undergone a substantial change over the last decade with the introduction of iterative reconstruction (IR) and now with deep learning reconstruction (DLR). In this review, DLR will be compared to IR and filtered back-projection (FBP) reconstructions. Comparisons will be made using image quality metrics such as noise power spectrum, contrast-dependent task-based transfer function, and non-prewhitening filter detectability index (dNPW'). Discussion on how DLR has impacted CT image quality, low-contrast detectability, and diagnostic confidence will be provided. DLR has shown the ability to improve in areas that IR is lacking, namely: noise magnitude reduction does not alter noise texture to the degree that IR did, and the noise texture found in DLR is more aligned with noise texture of an FBP reconstruction. Additionally, the dose reduction potential for DLR is shown to be greater than IR. For IR, the consensus was dose reduction should be limited to no more than 15-30% to preserve low-contrast detectability. For DLR, initial phantom and patient observer studies have shown acceptable dose reduction between 44 and 83% for both low- and high-contrast object detectability tasks. Ultimately, DLR is able to be used for CT reconstruction in place of IR, making it an easy "turnkey" upgrade for CT reconstruction. DLR for CT is actively being improved as more vendor options are being developed and current DLR options are being enhanced with second generation algorithms being released. DLR is still in its developmental early stages, but is shown to be a promising future for CT reconstruction.
更多查看译文
AI 理解论文
溯源树
样例
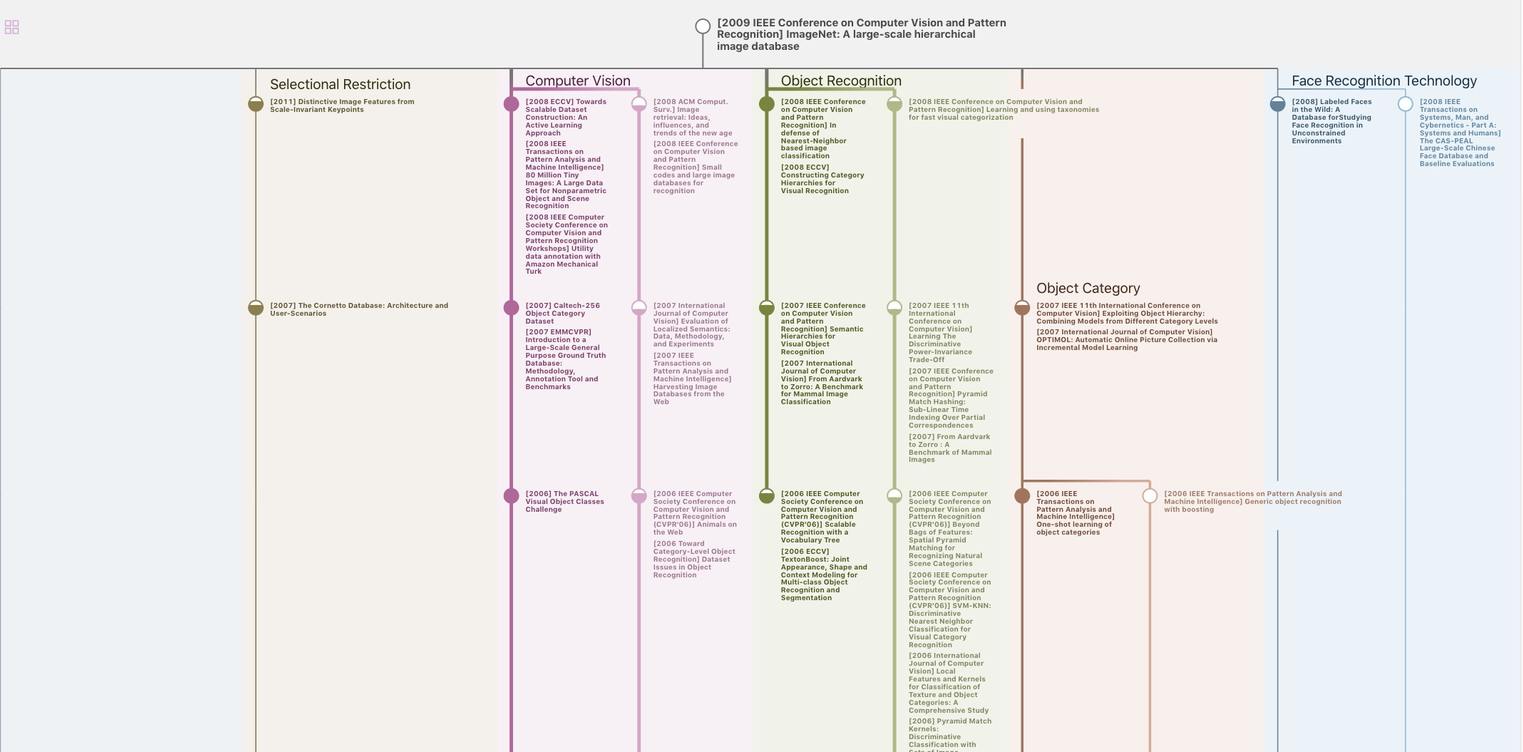
生成溯源树,研究论文发展脉络
Chat Paper
正在生成论文摘要