Unsupervised representation learning of spontaneous MEG data with Nonlinear ICA
NeuroImage(2023)
摘要
Resting-state magnetoencephalography (MEG) data show complex but structured spatiotemporal patterns. However, the neurophysiological basis of these signal patterns is not fully known and the underlying signal sources are mixed in MEG measurements. Here, we developed a method based on the nonlinear independent component analysis (ICA), a generative model trainable with unsupervised learning, to learn representations from resting-state MEG data. After being trained with a large dataset from the Cam-CAN repository, the model has learned to represent and generate patterns of spontaneous cortical activity using latent nonlinear components, which reflects principal cortical patterns with specific spectral modes. When applied to the downstream classification task of audio-visual MEG, the nonlinear ICA model achieves competitive performance with deep neural networks despite limited access to labels. We further validate the generalizability of the model across different datasets by applying it to an independent neurofeedback dataset for decoding the subject's attentional states, providing a real-time feature extraction and decoding mindfulness and thought-inducing tasks with an accuracy of around 70% at the individual level, which is much higher than obtained by linear ICA or other baseline methods. Our results demonstrate that nonlinear ICA is a valuable addition to existing tools, particularly suited for unsupervised representation learning of spontaneous MEG activity which can then be applied to specific goals or tasks when labelled data are scarce.
更多查看译文
关键词
Nonlinear independent component analysis (ICA),Unsupervised learning,Deep generative model,Resting-state network,Non-stationarity,Neurofeedback,Magnetoencephalography (MEG)
AI 理解论文
溯源树
样例
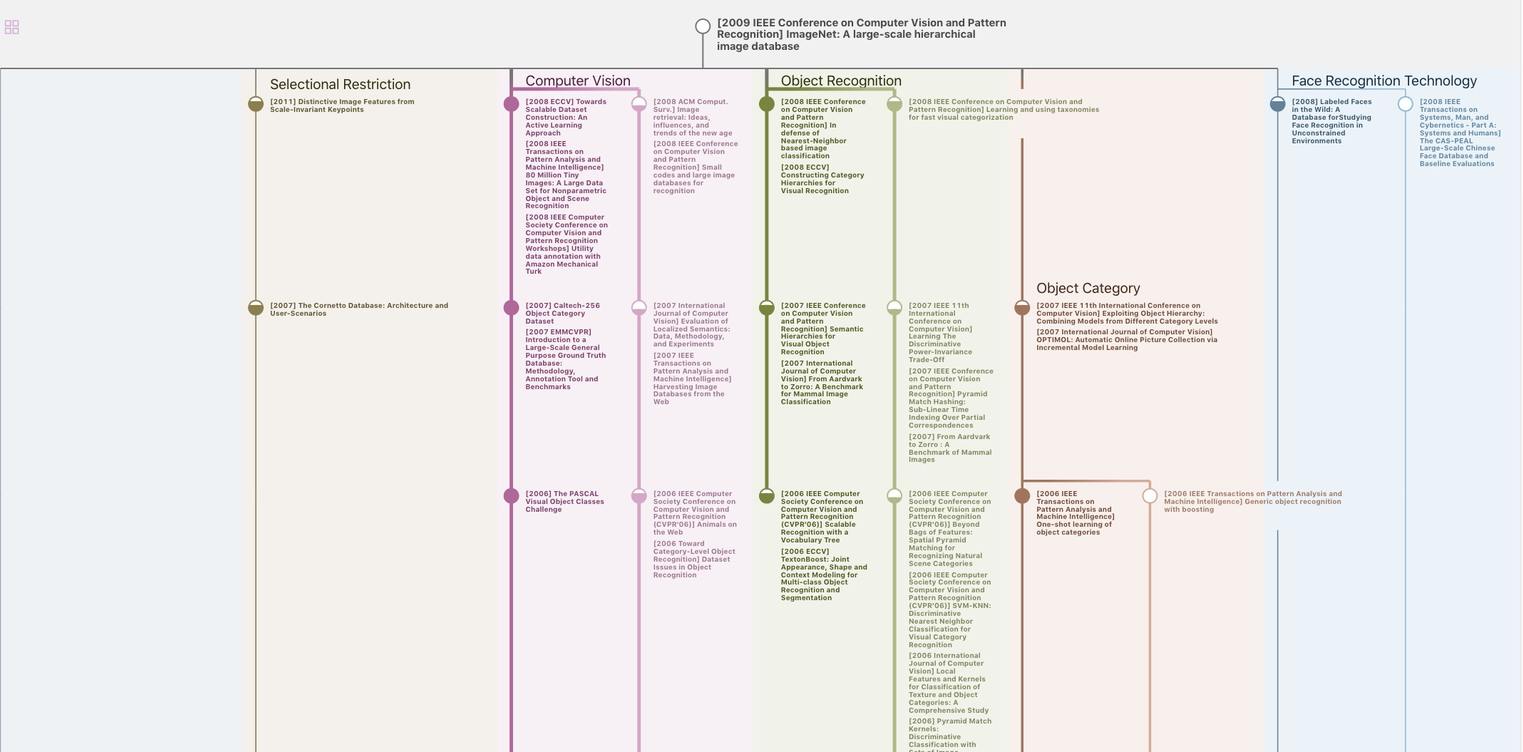
生成溯源树,研究论文发展脉络
Chat Paper
正在生成论文摘要