Motion-Conditioned Diffusion Model for Controllable Video Synthesis
CoRR(2023)
摘要
Recent advancements in diffusion models have greatly improved the quality and diversity of synthesized content. To harness the expressive power of diffusion models, researchers have explored various controllable mechanisms that allow users to intuitively guide the content synthesis process. Although the latest efforts have primarily focused on video synthesis, there has been a lack of effective methods for controlling and describing desired content and motion. In response to this gap, we introduce MCDiff, a conditional diffusion model that generates a video from a starting image frame and a set of strokes, which allow users to specify the intended content and dynamics for synthesis. To tackle the ambiguity of sparse motion inputs and achieve better synthesis quality, MCDiff first utilizes a flow completion model to predict the dense video motion based on the semantic understanding of the video frame and the sparse motion control. Then, the diffusion model synthesizes high-quality future frames to form the output video. We qualitatively and quantitatively show that MCDiff achieves the state-the-of-art visual quality in stroke-guided controllable video synthesis. Additional experiments on MPII Human Pose further exhibit the capability of our model on diverse content and motion synthesis.
更多查看译文
关键词
controllable video synthesis,diffusion model,motion-conditioned
AI 理解论文
溯源树
样例
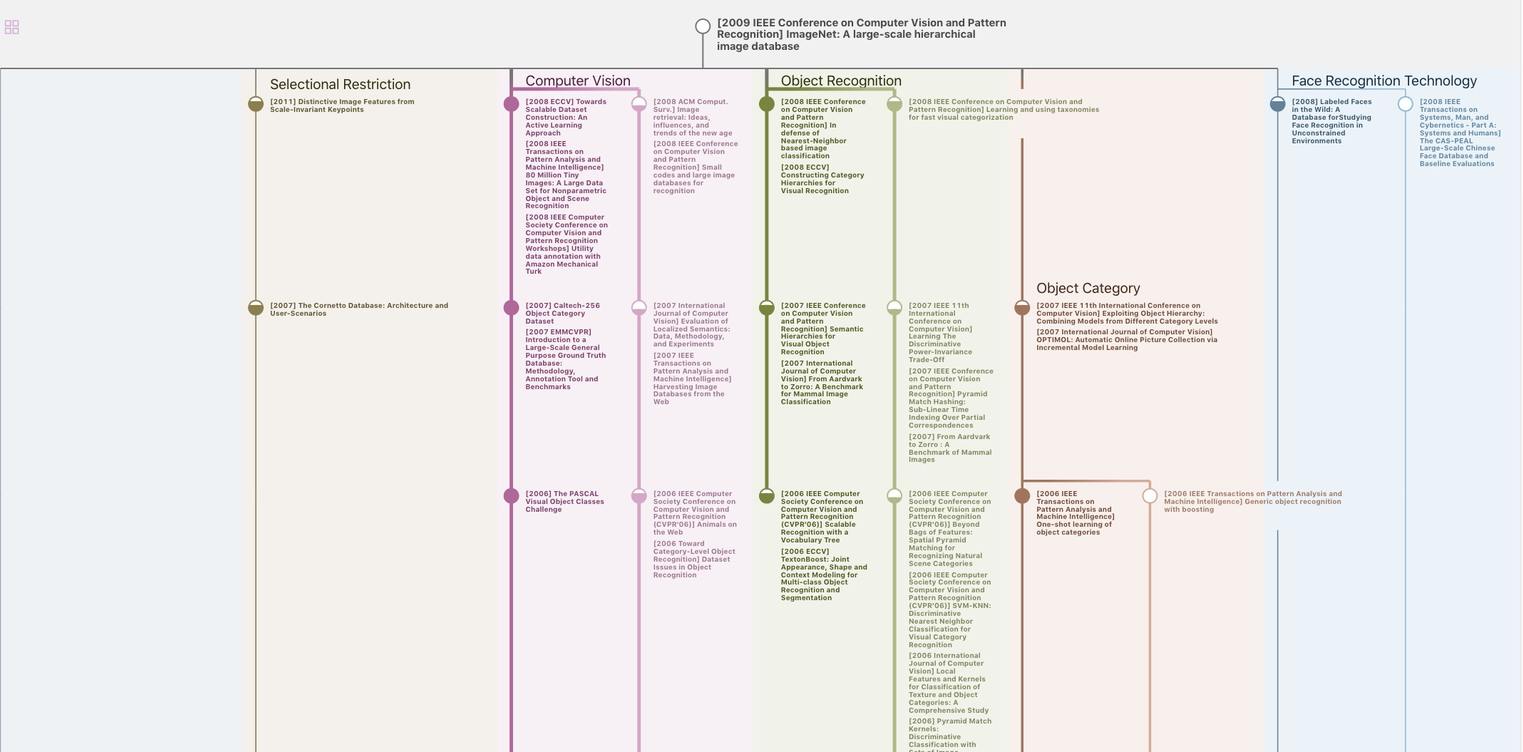
生成溯源树,研究论文发展脉络
Chat Paper
正在生成论文摘要