Lowering the Entry Bar to HPC-Scale Uncertainty Quantification
CoRR(2023)
Abstract
Treating uncertainties in models is essential in many fields of science and engineering. Uncertainty quantification (UQ) on complex and computationally costly numerical models necessitates a combination of efficient model solvers, advanced UQ methods and HPC-scale resources. The resulting technical complexities as well as lack of separation of concerns between UQ and model experts is holding back many interesting UQ applications. The aim of this paper is to close the gap between advanced UQ methods and advanced models by removing the hurdle of complex software stack integration, which in turn will offer a straightforward way to scale even prototype-grade UQ applications to high-performance resources. We achieve this goal by introducing a parallel software architecture based on UM-Bridge, a universal interface for linking UQ and models. We present three realistic applications from different areas of science and engineering, scaling from single machines to large clusters on the Google Cloud Platform.
MoreTranslated text
Key words
uncertainty,quantification,entry bar,hpc-scale
AI Read Science
Must-Reading Tree
Example
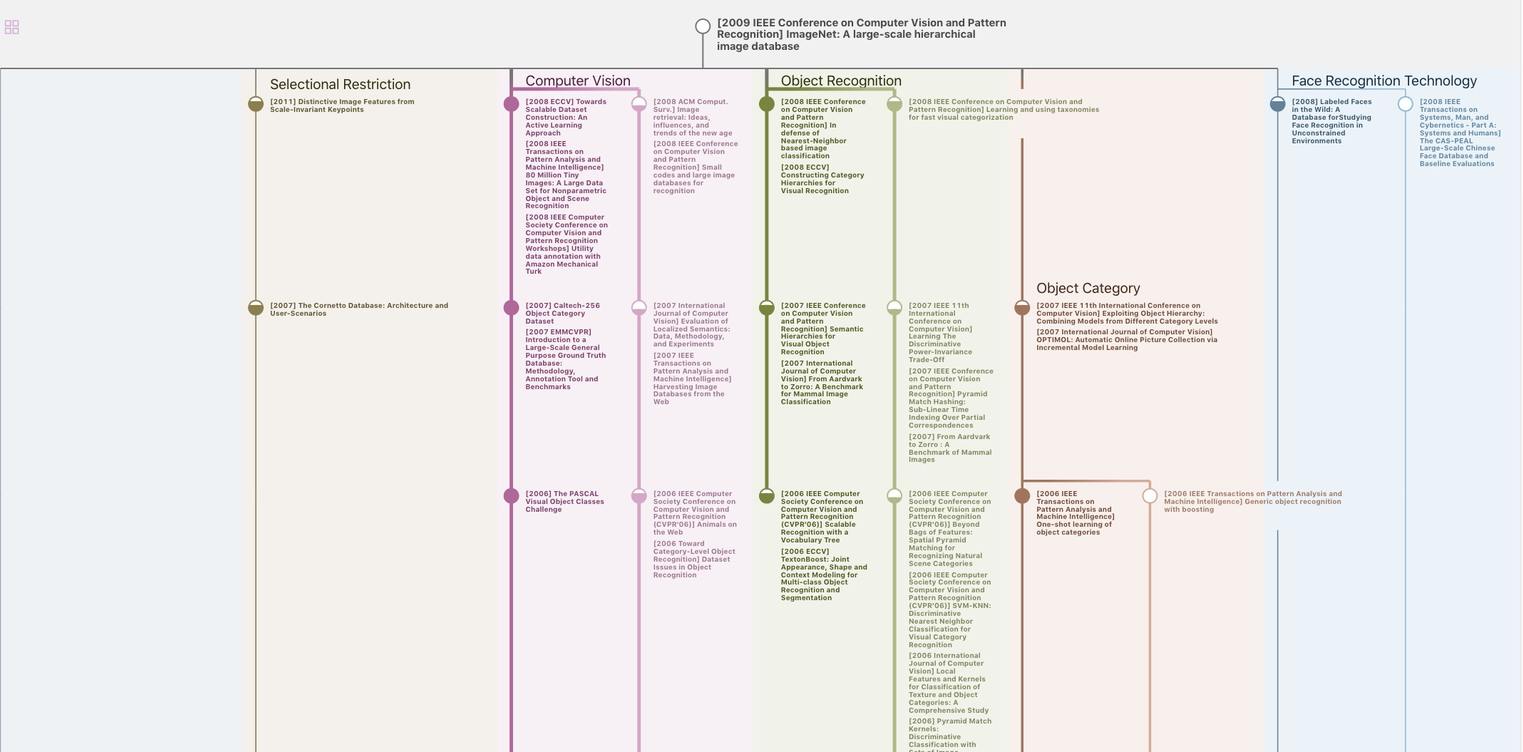
Generate MRT to find the research sequence of this paper
Chat Paper
Summary is being generated by the instructions you defined