Attacks on Robust Distributed Learning Schemes via Sensitivity Curve Maximization
CoRR(2023)
摘要
Distributed learning paradigms, such as federated or decentralized learning, allow a collection of agents to solve global learning and optimization problems through limited local interactions. Most such strategies rely on a mixture of local adaptation and aggregation steps, either among peers or at a central fusion center. Classically, aggregation in distributed learning is based on averaging, which is statistically efficient, but susceptible to attacks by even a small number of malicious agents. This observation has motivated a number of recent works, which develop robust aggregation schemes by employing robust variations of the mean. We present a new attack based on sensitivity curve maximization (SCM), and demonstrate that it is able to disrupt existing robust aggregation schemes by injecting small, but effective perturbations.
更多查看译文
关键词
Decentralized learning,federated learning,robust aggregation,byzantine robustness,sensitivity curve
AI 理解论文
溯源树
样例
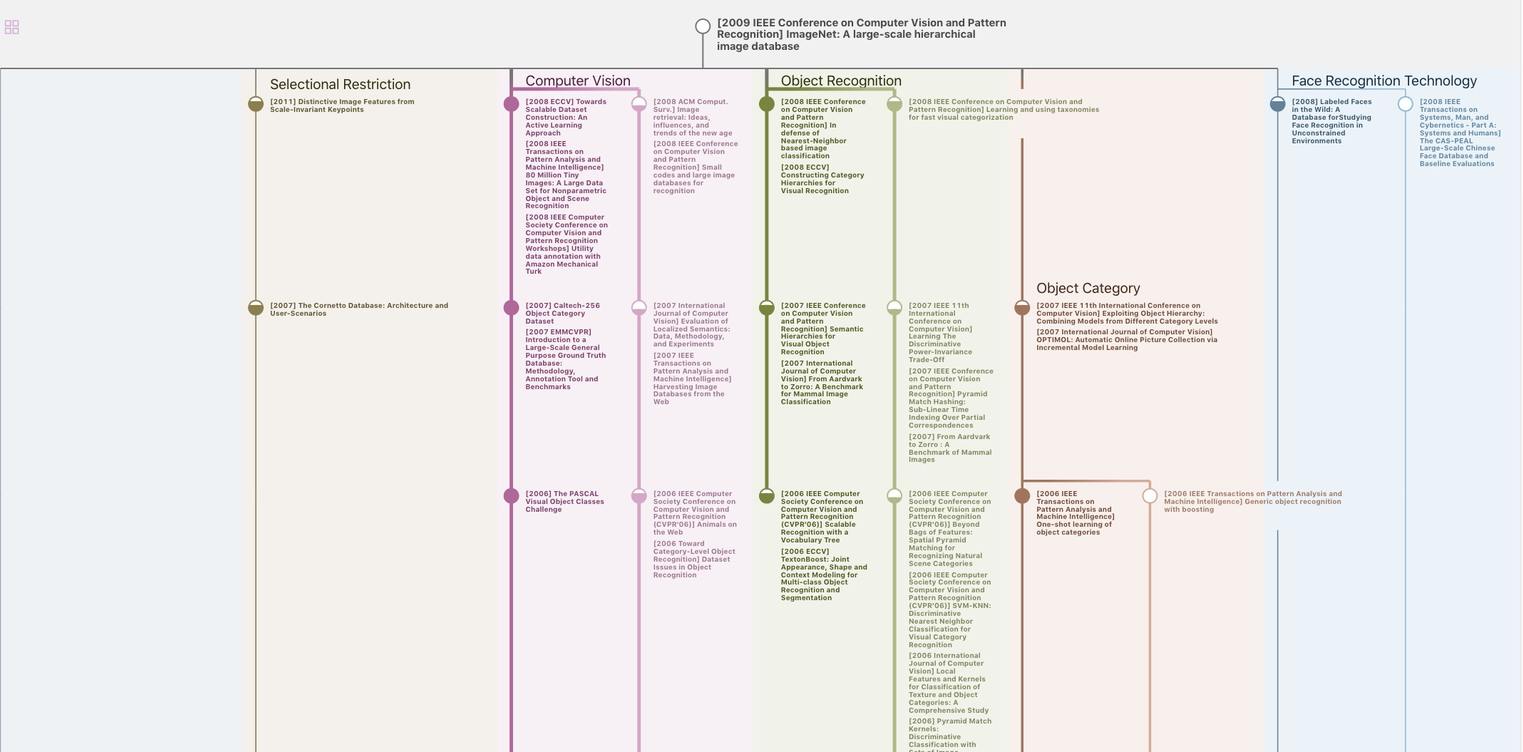
生成溯源树,研究论文发展脉络
Chat Paper
正在生成论文摘要