Maximizing Submodular Functions for Recommendation in the Presence of Biases
WWW 2023(2023)
摘要
Subset selection tasks, arise in recommendation systems and search engines and ask to select a subset of items that maximize the value for the user. The values of subsets often display diminishing returns, and hence, submodular functions have been used to model them. If the inputs defining the submodular function are known, then existing algorithms can be used. In many applications, however, inputs have been observed to have social biases that reduce the utility of the output subset. Hence, interventions to improve the utility are desired. Prior works focus on maximizing linear functions—a special case of submodular functions—and show that fairness constraint-based interventions can not only ensure proportional representation but also achieve near-optimal utility in the presence of biases. We study the maximization of a family of submodular functions that capture functions arising in the aforementioned applications. Our first result is that, unlike linear functions, constraint-based interventions cannot guarantee any constant fraction of the optimal utility for this family of submodular functions. Our second result is an algorithm for submodular maximization. The algorithm provably outputs subsets that have near-optimal utility for this family under mild assumptions and that proportionally represent items from each group. In empirical evaluation, with both synthetic and real-world data, we observe that this algorithm improves the utility of the output subset for this family of submodular functions over baselines.
更多查看译文
关键词
maximizing submodular functions,recommendation
AI 理解论文
溯源树
样例
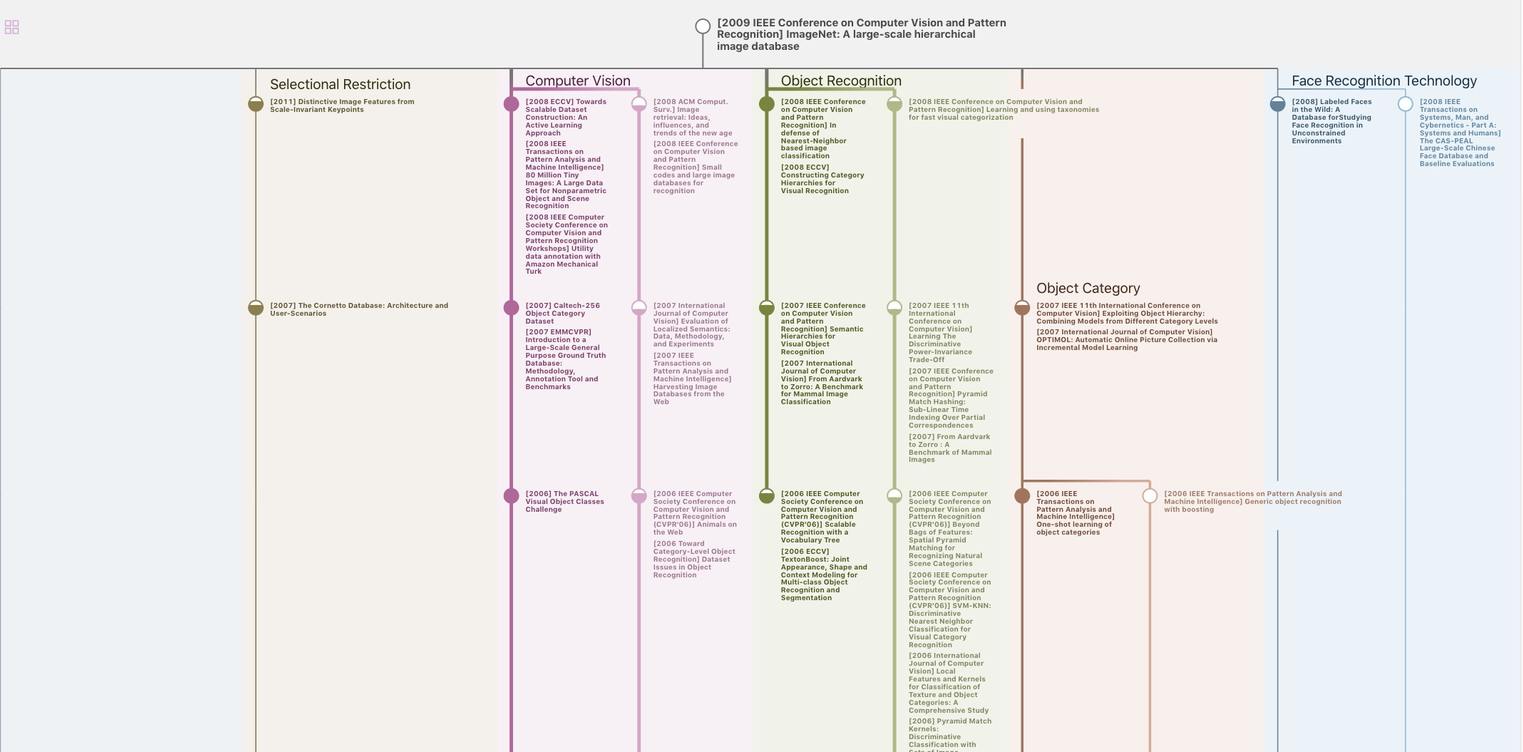
生成溯源树,研究论文发展脉络
Chat Paper
正在生成论文摘要