Domain Adaptive and Generalizable Network Architectures and Training Strategies for Semantic Image Segmentation
IEEE TRANSACTIONS ON PATTERN ANALYSIS AND MACHINE INTELLIGENCE(2024)
摘要
Unsupervised domain adaptation (UDA) and domain generalization (DG) enable machine learning models trained on a source domain to perform well on unlabeled or even unseen target domains. As previous UDA&DG semantic segmentation methods are mostly based on outdated networks, we benchmark more recent architectures, reveal the potential of Transformers, and design the DAFormer network tailored for UDA&DG. It is enabled by three training strategies to avoid overfitting to the source domain: While (1) Rare Class Sampling mitigates the bias toward common source domain classes, (2) a Thing-Class ImageNet Feature Distance and (3) a learning rate warmup promote feature transfer from ImageNet pretraining. As UDA&DG are usually GPU memory intensive, most previous methods downscale or crop images. However, low-resolution predictions often fail to preserve fine details while models trained with cropped images fall short in capturing long-range, domain-robust context information. Therefore, we propose HRDA, a multi-resolution framework for UDA&DG, that combines the strengths of small high-resolution crops to preserve fine segmentation details and large low-resolution crops to capture long-range context dependencies with a learned scale attention. DAFormer and HRDA significantly improve the state-of-the-art UDA&DG by more than 10 mIoU on 5 different benchmarks.
更多查看译文
关键词
Domain adaptation,domain generalization,semantic segmentation,transformers,high-resolution,multi-resolution
AI 理解论文
溯源树
样例
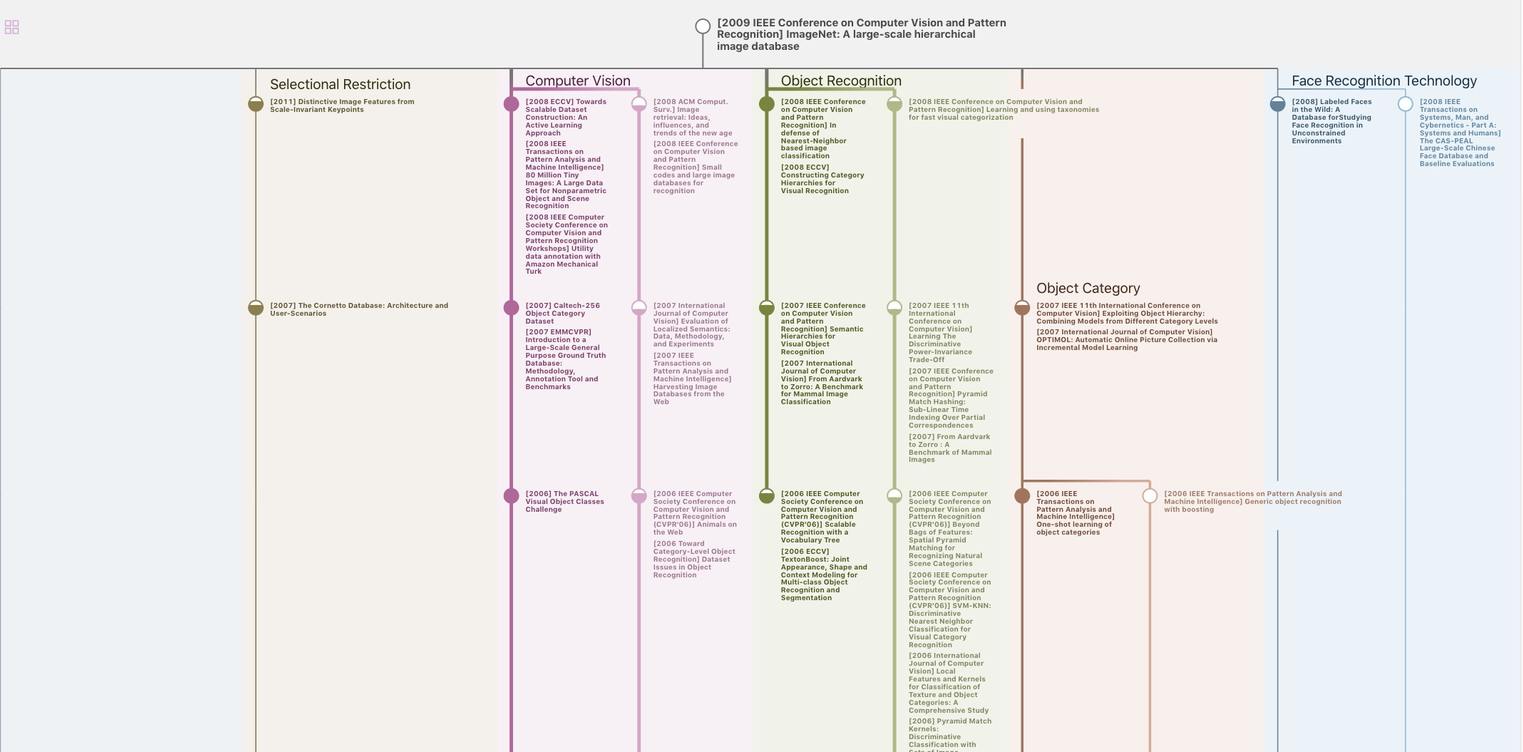
生成溯源树,研究论文发展脉络
Chat Paper
正在生成论文摘要