Kernel methods are competitive for operator learning
JOURNAL OF COMPUTATIONAL PHYSICS(2024)
摘要
We present a general kernel-based framework for learning operators between Banach spaces along with a priori error analysis and comprehensive numerical comparisons with popular neural net (NN) approaches such as Deep Operator Networks (DeepONet) [46] and Fourier Neural Operator (FNO) [45]. We consider the setting where the input/output spaces of target operator g(dagger) : v -> nu are reproducing kernel Hilbert spaces (RKHS), the data comes in the form of partial observations phi(u(t)), phi(v(t)) of input/output functions v(t) = G(dagger)(u(t)) (t = 1,...,N), and the measurement operators phi : V -> R-n and phi: nu -> R-n are linear. Writing psi : R-n -> V and chi : R-n -> nu for the optimal recovery maps associated with phi and phi, we approximate G(dagger) with (G) over bar = chi circle(f) over bar circle phi where (f) over bar is an optimal recovery approximation of f(dagger) := phi circle G dagger circle psi : R-n -> R-m. We show that, even when using vanilla kernels (e.g., linear or Matern), our approach is competitive in terms of cost-accuracy trade-off and either matches or beats the performance of NN methods on a majority of benchmarks. Additionally, our framework offers several advantages inherited from kernel methods: simplicity, interpretability, convergence guarantees, a priori error estimates, and Bayesian uncertainty quantification. As such, it can serve as a natural benchmark for operator learning.
更多查看译文
关键词
Operator learning,Optimal recovery,Kernel methods,Gaussian processes,Functional regression,Partial differential equations
AI 理解论文
溯源树
样例
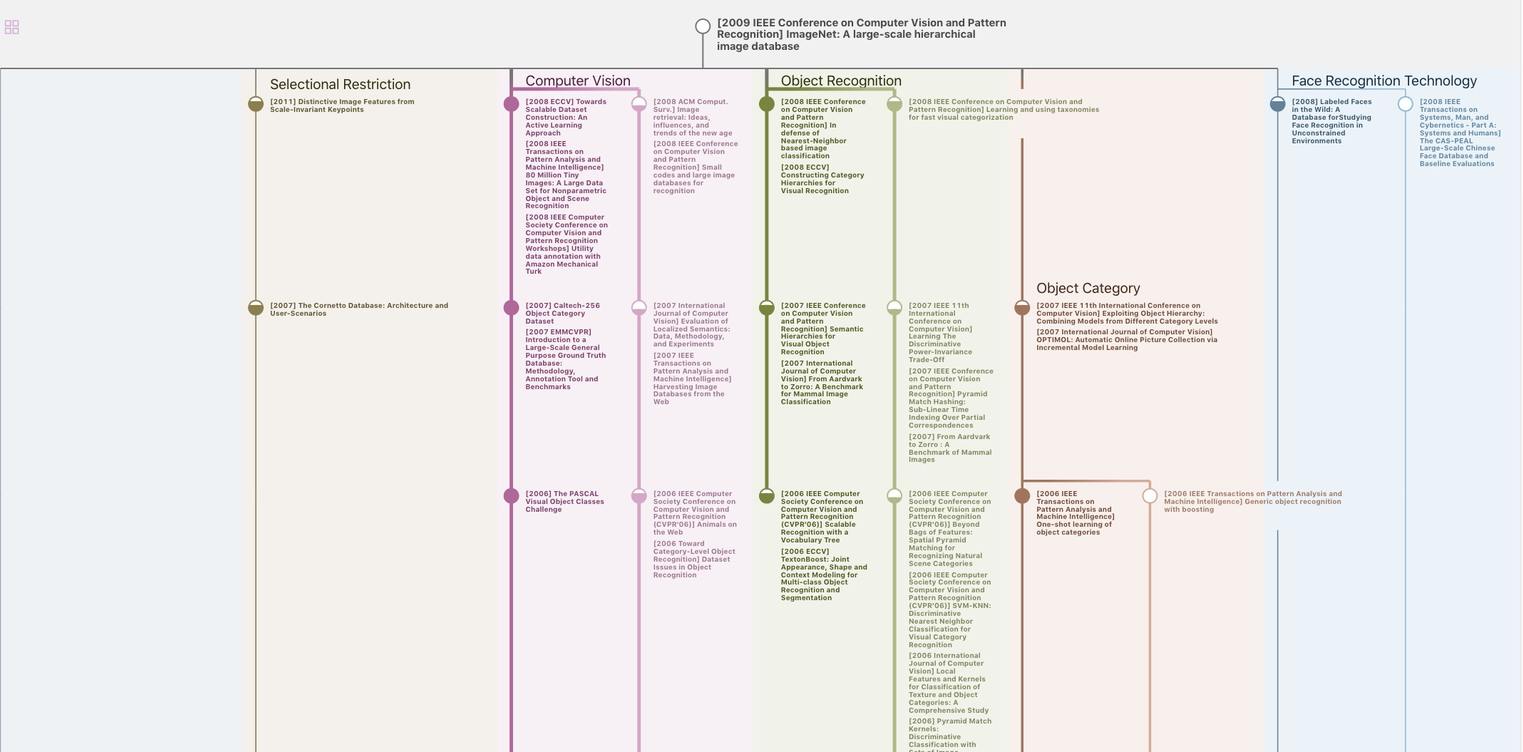
生成溯源树,研究论文发展脉络
Chat Paper
正在生成论文摘要