DQS3D: Densely-matched Quantization-aware Semi-supervised 3D Detection
2023 IEEE/CVF International Conference on Computer Vision (ICCV)(2023)
摘要
In this paper, we study the problem of semi-supervised 3D object detection, which is of great importance considering the high annotation cost for cluttered 3D indoor scenes. We resort to the robust and principled framework of selfteaching, which has triggered notable progress for semisupervised learning recently. While this paradigm is natural for image-level or pixel-level prediction, adapting it to the detection problem is challenged by the issue of proposal matching. Prior methods are based upon two-stage pipelines, matching heuristically selected proposals generated in the first stage and resulting in spatially sparse training signals. In contrast, we propose the first semisupervised 3D detection algorithm that works in the singlestage manner and allows spatially dense training signals. A fundamental issue of this new design is the quantization error caused by point-to-voxel discretization, which inevitably leads to misalignment between two transformed views in the voxel domain. To this end, we derive and implement closed-form rules that compensate this misalignment onthe-fly. Our results are significant, e.g., promoting ScanNet mAP@0.5 from 35.2% to 48.5% using 20% annotation. Codes and data will be publicly available.
更多查看译文
关键词
dqs3d,detection,densely-matched,quantization-aware,semi-supervised
AI 理解论文
溯源树
样例
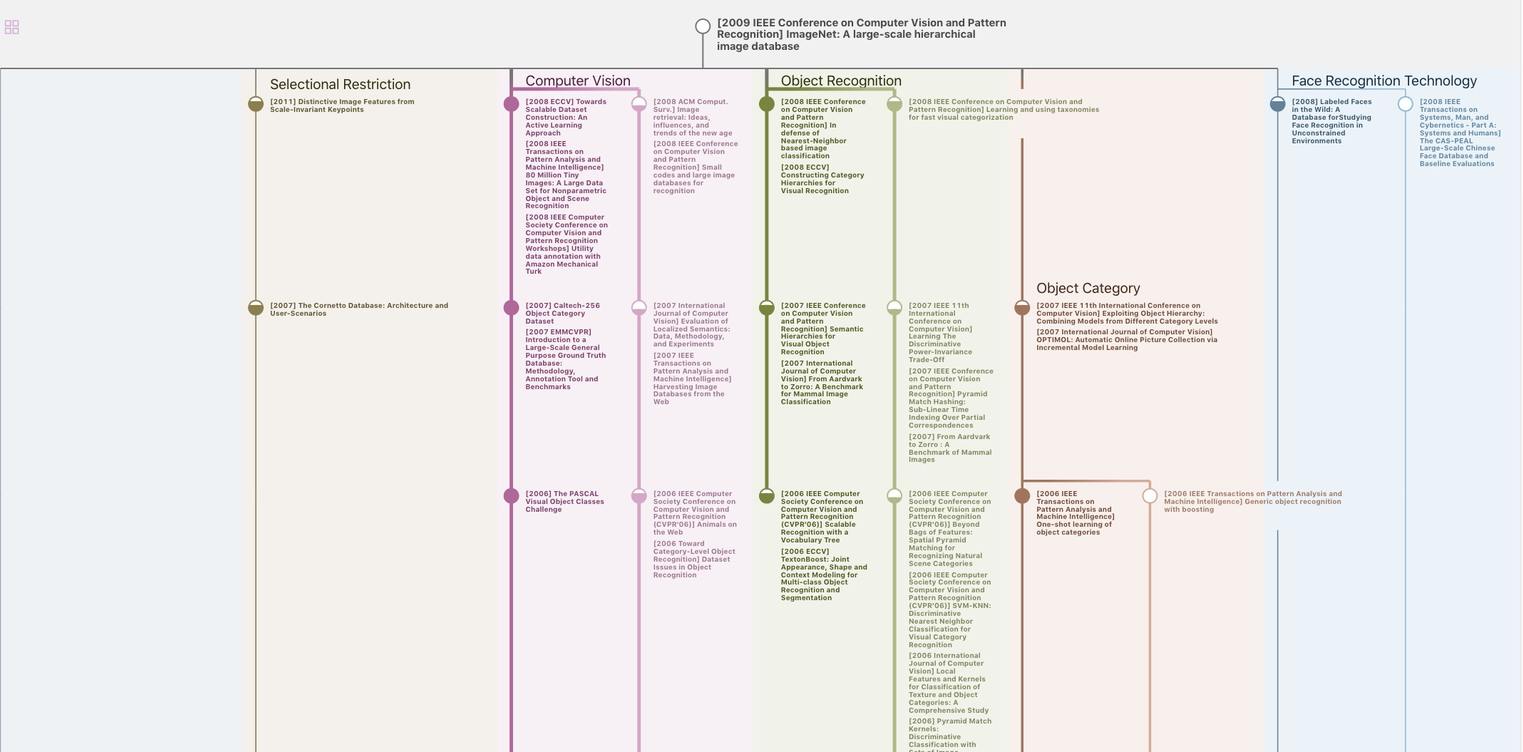
生成溯源树,研究论文发展脉络
Chat Paper
正在生成论文摘要