Morphological Classification of Extragalactic Radio Sources Using Gradient Boosting Methods
CoRR(2023)
摘要
The field of radio astronomy is witnessing a boom in the amount of data produced per day due to newly commissioned radio telescopes. One of the most crucial problems in this field is the automatic classification of extragalactic radio sources based on their morphologies. Most recent contributions in the field of morphological classification of extragalactic radio sources have proposed classifiers based on convolutional neural networks. Alternatively, this work proposes gradient boosting machine learning methods accompanied by principal component analysis as data-efficient alternatives to convolutional neural networks. Recent findings have shown the efficacy of gradient boosting methods in outperforming deep learning methods for classification problems with tabular data. The gradient boosting methods considered in this work are based on the XGBoost, LightGBM, and CatBoost implementations. This work also studies the effect of dataset size on classifier performance. A three-class classification problem is considered in this work based on the three main Fanaroff-Riley classes: class 0, class I, and class II, using radio sources from the Best-Heckman sample. All three proposed gradient boosting methods outperformed a state-of-the-art convolutional neural networks-based classifier using less than a quarter of the number of images, with CatBoost having the highest accuracy. This was mainly due to the superior accuracy of gradient boosting methods in classifying Fanaroff-Riley class II sources, with 3--4\% higher recall.
更多查看译文
关键词
extragalactic radio sources,gradient boosting methods,classification
AI 理解论文
溯源树
样例
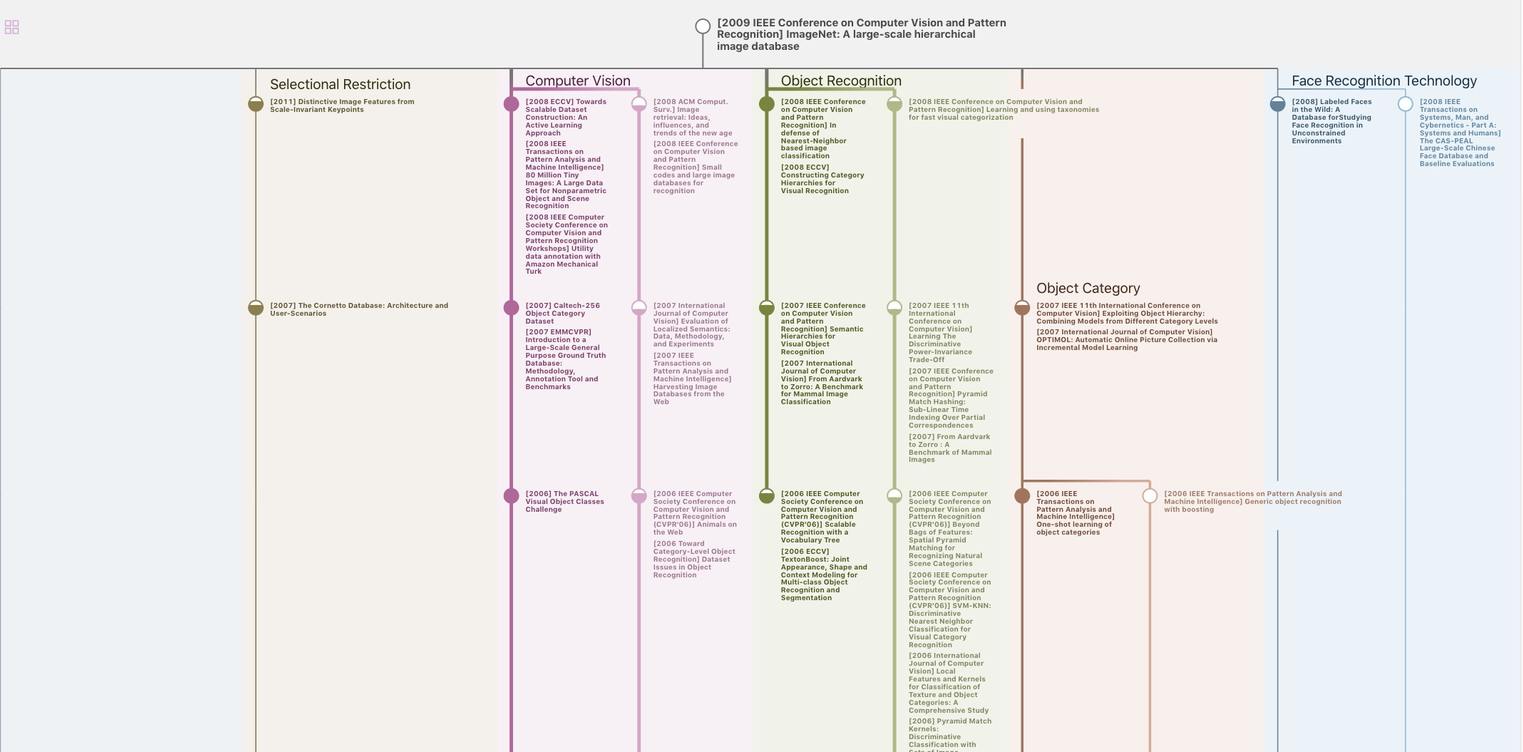
生成溯源树,研究论文发展脉络
Chat Paper
正在生成论文摘要