Robust, randomized preconditioning for kernel ridge regression
CoRR(2023)
摘要
This paper introduces two randomized preconditioning techniques for robustly solving kernel ridge regression (KRR) problems with a medium to large number of data points ($10^4 \leq N \leq 10^7$). The first method, RPCholesky preconditioning, is capable of accurately solving the full-data KRR problem in $O(N^2)$ arithmetic operations, assuming sufficiently rapid polynomial decay of the kernel matrix eigenvalues. The second method, KRILL preconditioning, offers an accurate solution to a restricted version of the KRR problem involving $k \ll N$ selected data centers at a cost of $O((N + k^2) k \log k)$ operations. The proposed methods solve a broad range of KRR problems and overcome the failure modes of previous KRR preconditioners, making them ideal for practical applications.
更多查看译文
关键词
kernel ridge regression,randomized
AI 理解论文
溯源树
样例
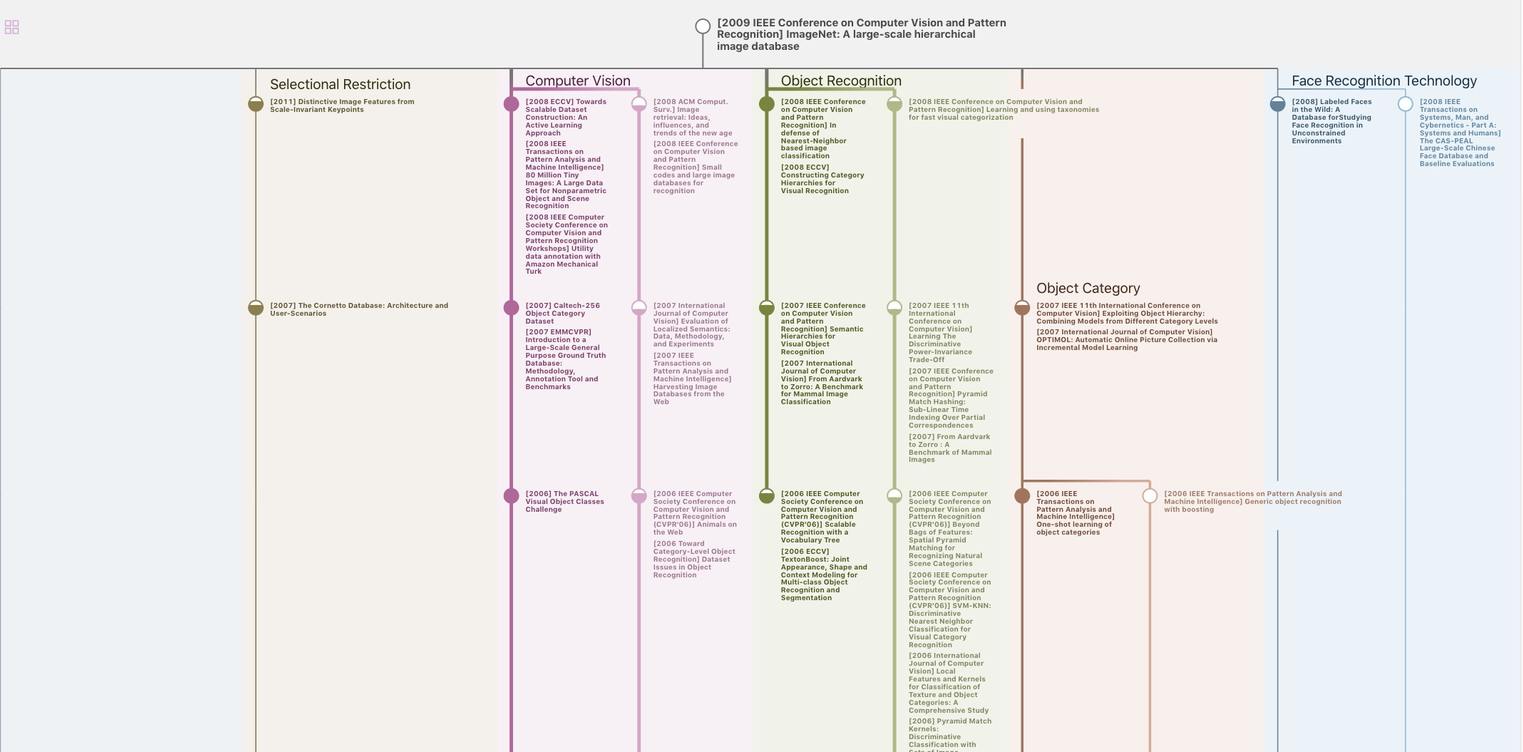
生成溯源树,研究论文发展脉络
Chat Paper
正在生成论文摘要