Parallel Bootstrap-Based On-Policy Deep Reinforcement Learning for Continuous Fluid Flow Control Applications
CoRR(2023)
摘要
The coupling of deep reinforcement learning to numerical flow control problems has recently received considerable attention, leading to groundbreaking results and opening new perspectives for the domain. Due to the usually high computational cost of fluid dynamics solvers, the use of parallel environments during the learning process represents an essential ingredient to attain efficient control in a reasonable time. Yet, most of the deep reinforcement learning literature for flow control relies on on-policy algorithms, for which the massively parallel transition collection may break theoretical assumptions and lead to suboptimal control models. To overcome this issue, we propose a parallelism pattern relying on partial-trajectory buffers terminated by a return bootstrapping step, allowing a flexible use of parallel environments while preserving the on-policiness of the updates. This approach is illustrated on a CPU-intensive continuous flow control problem from the literature.
更多查看译文
关键词
deep reinforcement learning,flow control,proximal policy optimization,parallel environments,bootstrapping
AI 理解论文
溯源树
样例
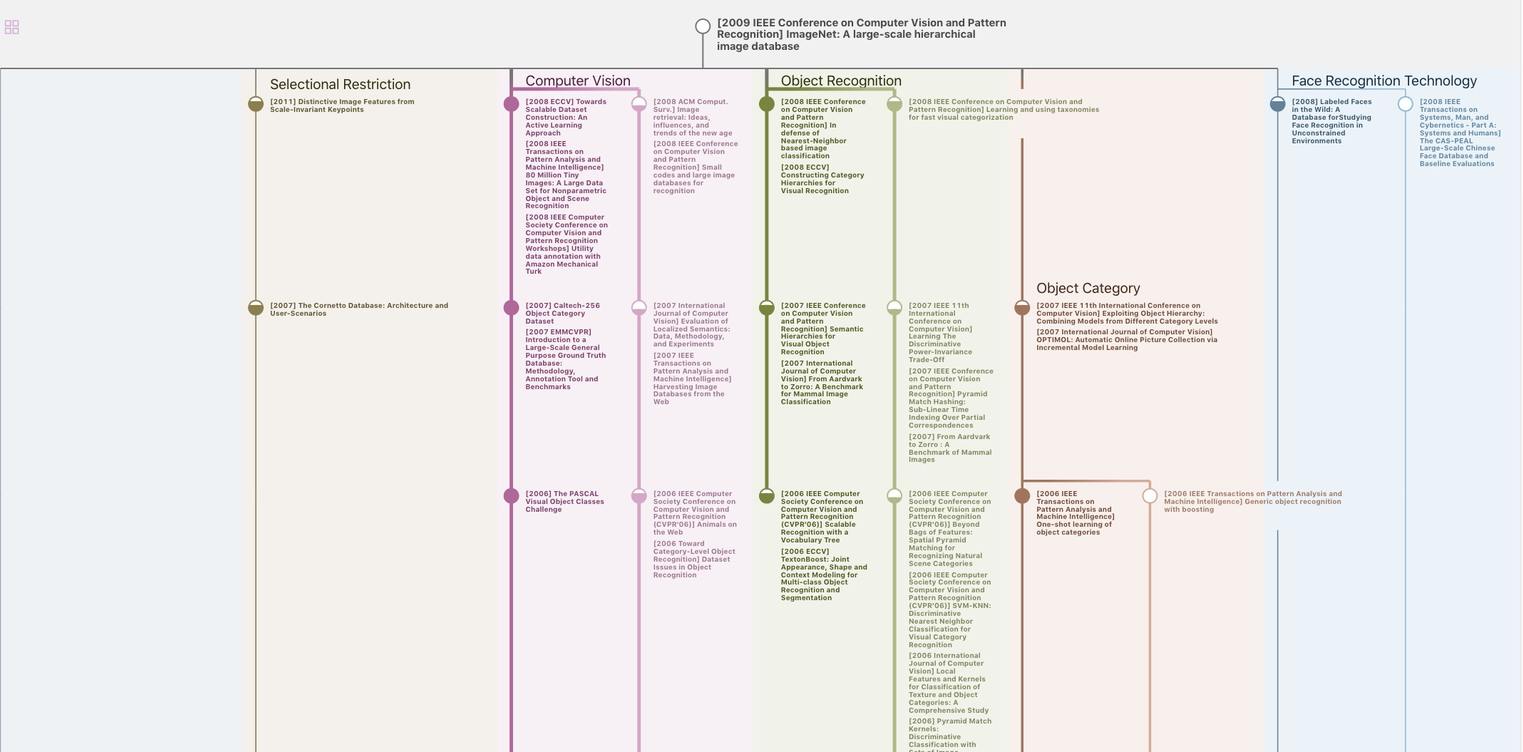
生成溯源树,研究论文发展脉络
Chat Paper
正在生成论文摘要