A Spatial Calibration Method for Robust Cooperative Perception
IEEE ROBOTICS AND AUTOMATION LETTERS(2024)
摘要
Cooperative perception is a promising technique for intelligent and connected vehicles through vehicle-to-everything (V2X) cooperation, provided that accurate pose information and relative pose transforms are available. Nevertheless, obtaining precise positioning information often entails high costs associated with navigation systems. Hence, it is required to calibrate relative pose information for multi-agent cooperative perception. This letter proposes a simple but effective object association approach named context-based matching (CBM), which identifies inter-agent object correspondences using intra-agent geometrical context. In detail, this method constructs contexts using the relative position of the detected bounding boxes, followed by local context matching and global consensus maximization. The optimal relative pose transform is estimated based on the matched correspondences, followed by cooperative perception fusion. Extensive experiments are conducted on both the simulated and real-world datasets. Even with larger inter-agent localization errors, high object association precision and decimeter-level relative pose calibration accuracy are achieved among the cooperating agents.
更多查看译文
关键词
Calibration,Location awareness,Feature extraction,Transforms,Rotation measurement,Vectors,Robustness,Distributed robot systems,object detection,pose errors,robustness
AI 理解论文
溯源树
样例
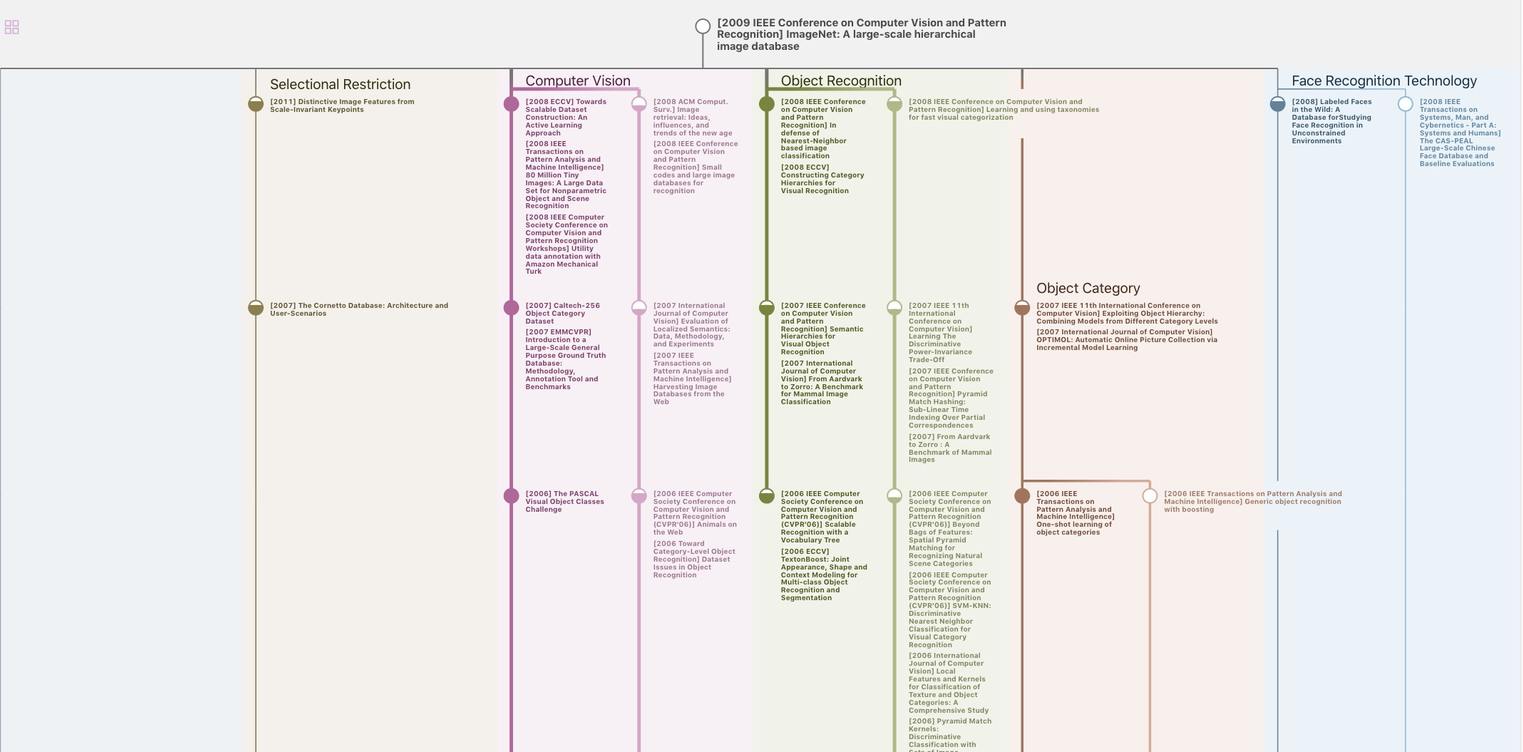
生成溯源树,研究论文发展脉络
Chat Paper
正在生成论文摘要