Advancing underwater acoustic target recognition via adaptive data pruning and smoothness-inducing regularization
CoRR(2023)
摘要
Underwater acoustic recognition for ship-radiated signals has high practical application value due to the ability to recognize non-line-of-sight targets. However, due to the difficulty of data acquisition, the collected signals are scarce in quantity and mainly composed of mechanical periodic noise. According to the experiments, we observe that the repeatability of periodic signals leads to a double-descent phenomenon, which indicates a significant local bias toward repeated samples. To address this issue, we propose a strategy based on cross-entropy to prune excessively similar segments in training data. Furthermore, to compensate for the reduction of training data, we generate noisy samples and apply smoothness-inducing regularization based on KL divergence to mitigate overfitting. Experiments show that our proposed data pruning and regularization strategy can bring stable benefits and our framework significantly outperforms the state-of-the-art in low-resource scenarios.
更多查看译文
关键词
underwater acoustic target recognition,adaptive data
AI 理解论文
溯源树
样例
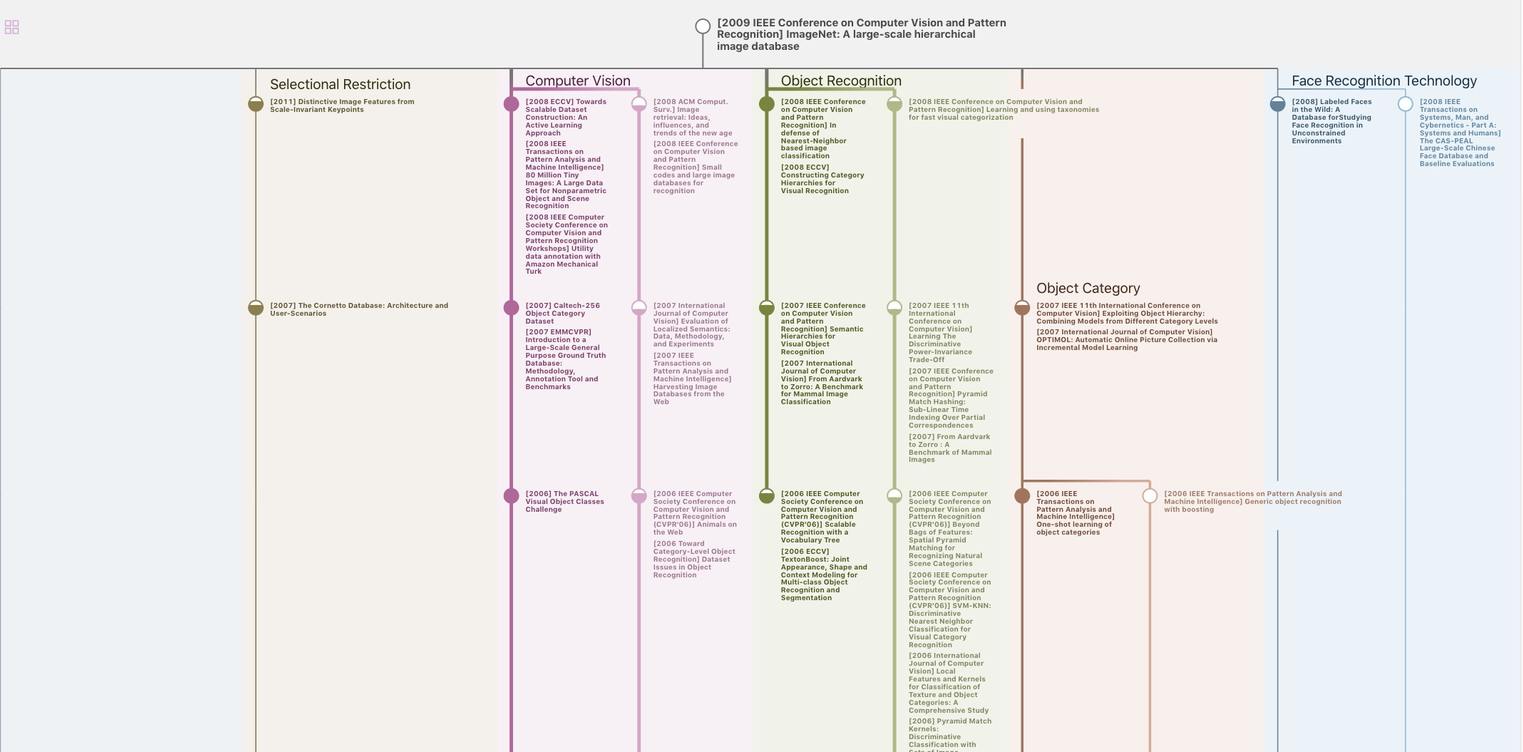
生成溯源树,研究论文发展脉络
Chat Paper
正在生成论文摘要