Machine learning for predicting fatigue properties of additively manufactured materials
CHINESE JOURNAL OF AERONAUTICS(2024)
Abstract
Fatigue properties of materials by Additive Manufacturing (AM) depend on many factors such as AM processing parameter, microstructure, residual stress, surface roughness, porosities, post -treatments, etc. Their evaluation inevitably requires these factors combined as many as possible, thus resulting in low efficiency and high cost. In recent years, their assessment by leveraging the power of Machine Learning (ML) has gained increasing attentions. A comprehensive overview on the state-of-the-art progress of applying ML strategies to predict fatigue properties of AM materials, as well as their dependence on AM processing and post -processing parameters such as laser power, scanning speed, layer height, hatch distance, built direction, post -heat temperature, etc., were presented. A few attempts in employing Feedforward Neural Network (FNN), Convolutional Neural Network (CNN), Adaptive Network -Based Fuzzy Inference System (ANFIS), Support Vector Machine (SVM) and Random Forest (RF) to predict fatigue life and RF to predict fatigue crack growth rate are summarized. The ML models for predicting AM materials' fatigue properties are found intrinsically similar to the commonly used ones, but are modified to involve AM features. Finally, an outlook for challenges (i.e., small dataset, multifarious features, overfitting, low interpretability, and unable extension from AM material data to structure life) and potential solutions for the ML prediction of AM materials' fatigue properties is provided. (c) 2023 Production and hosting by Elsevier Ltd. on behalf of Chinese Society of Aeronautics and Astronautics. This is an open access article under the CC BY -NC -ND license.
MoreTranslated text
Key words
Additive manufacturing,Machine learning,Fatigue life,Fatigue crack growth rate,Prediction
AI Read Science
Must-Reading Tree
Example
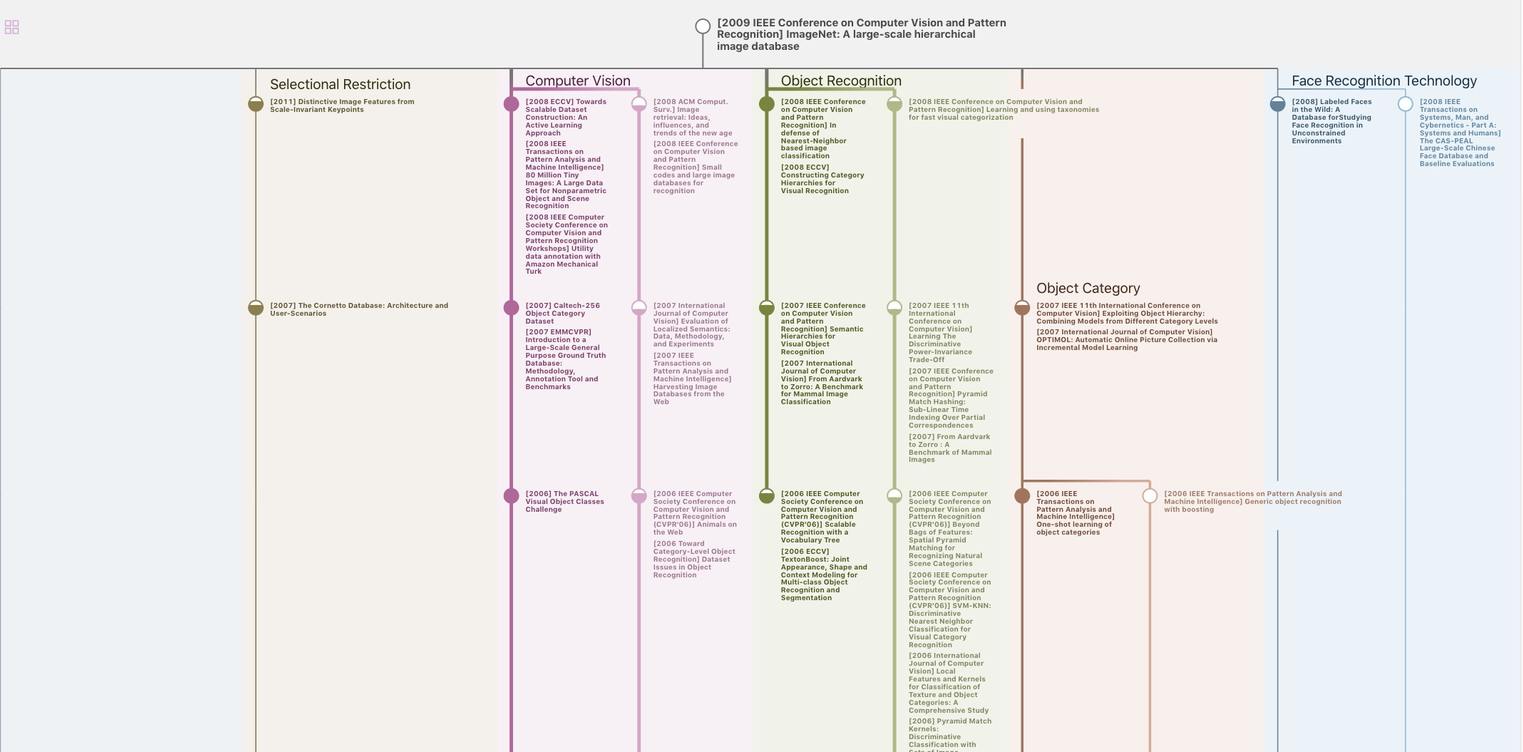
Generate MRT to find the research sequence of this paper
Chat Paper
Summary is being generated by the instructions you defined