Walking Your LiDOG: A Journey Through Multiple Domains for LiDAR Semantic Segmentation
2023 IEEE/CVF International Conference on Computer Vision (ICCV)(2023)
Abstract
The ability to deploy robots that can operate safely in diverse environments is crucial for developing embodied intelligent agents. As a community, we have made tremendous progress in within-domain LiDAR semantic segmentation. However, do these methods generalize across domains? To answer this question, we design the first experimental setup for studying domain generalization (DG) for LiDAR semantic segmentation (DG-LSS). Our results confirm a significant gap between methods, evaluated in a cross-domain setting: for example, a model trained on the source dataset (SemanticKITTI) obtains $26.53$ mIoU on the target data, compared to $48.49$ mIoU obtained by the model trained on the target domain (nuScenes). To tackle this gap, we propose the first method specifically designed for DG-LSS, which obtains $34.88$ mIoU on the target domain, outperforming all baselines. Our method augments a sparse-convolutional encoder-decoder 3D segmentation network with an additional, dense 2D convolutional decoder that learns to classify a birds-eye view of the point cloud. This simple auxiliary task encourages the 3D network to learn features that are robust to sensor placement shifts and resolution, and are transferable across domains. With this work, we aim to inspire the community to develop and evaluate future models in such cross-domain conditions.
MoreTranslated text
Key words
lidar,semantic segmentation,lidog,journey
AI Read Science
Must-Reading Tree
Example
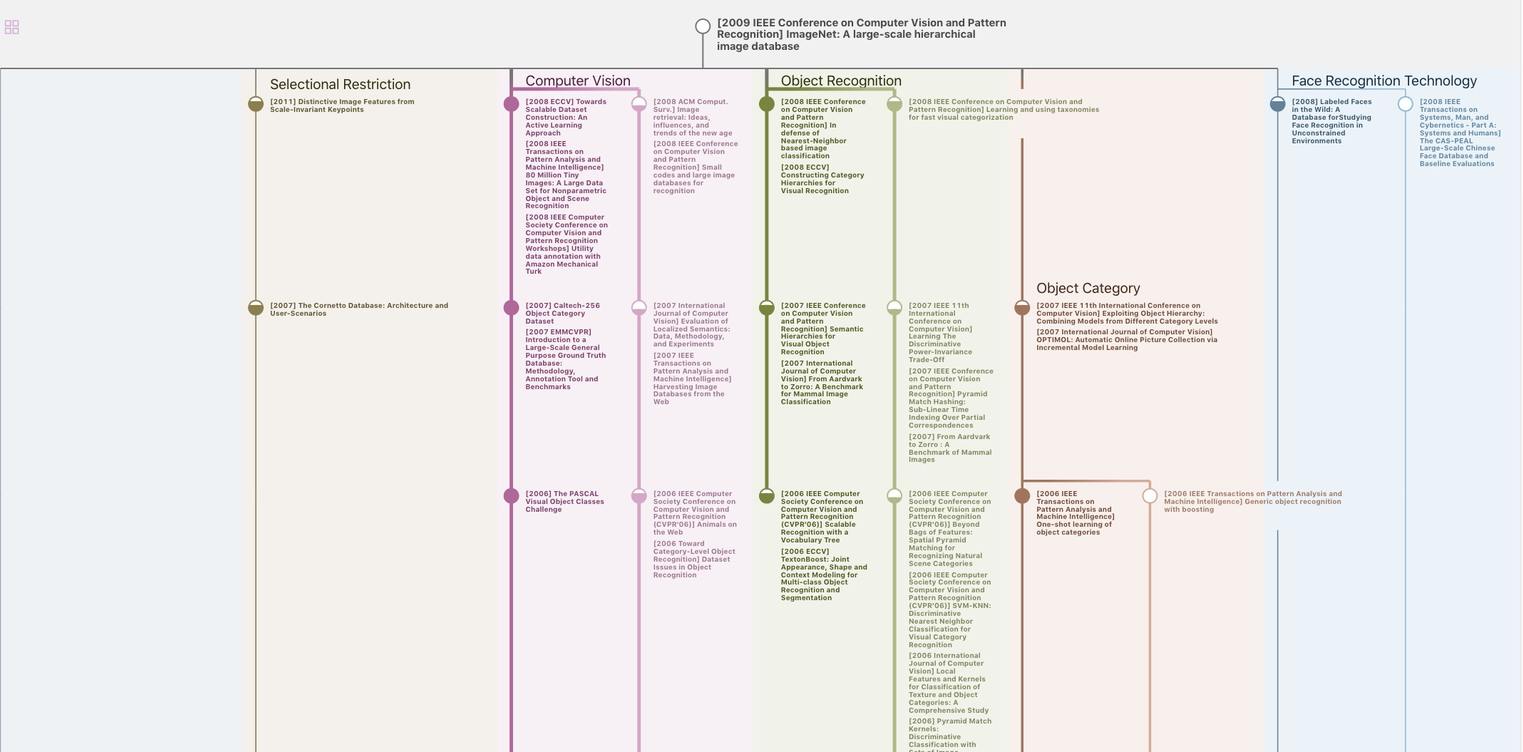
Generate MRT to find the research sequence of this paper
Chat Paper
Summary is being generated by the instructions you defined