Evaluating ChatGPT's Information Extraction Capabilities: An Assessment of Performance, Explainability, Calibration, and Faithfulness
CoRR(2023)
摘要
The capability of Large Language Models (LLMs) like ChatGPT to comprehend user intent and provide reasonable responses has made them extremely popular lately. In this paper, we focus on assessing the overall ability of ChatGPT using 7 fine-grained information extraction (IE) tasks. Specially, we present the systematically analysis by measuring ChatGPT's performance, explainability, calibration, and faithfulness, and resulting in 15 keys from either the ChatGPT or domain experts. Our findings reveal that ChatGPT's performance in Standard-IE setting is poor, but it surprisingly exhibits excellent performance in the OpenIE setting, as evidenced by human evaluation. In addition, our research indicates that ChatGPT provides high-quality and trustworthy explanations for its decisions. However, there is an issue of ChatGPT being overconfident in its predictions, which resulting in low calibration. Furthermore, ChatGPT demonstrates a high level of faithfulness to the original text in the majority of cases. We manually annotate and release the test sets of 7 fine-grained IE tasks contains 14 datasets to further promote the research. The datasets and code are available at https://github.com/pkuserc/ChatGPT_for_IE.
更多查看译文
关键词
information extraction capabilities,chatgpt
AI 理解论文
溯源树
样例
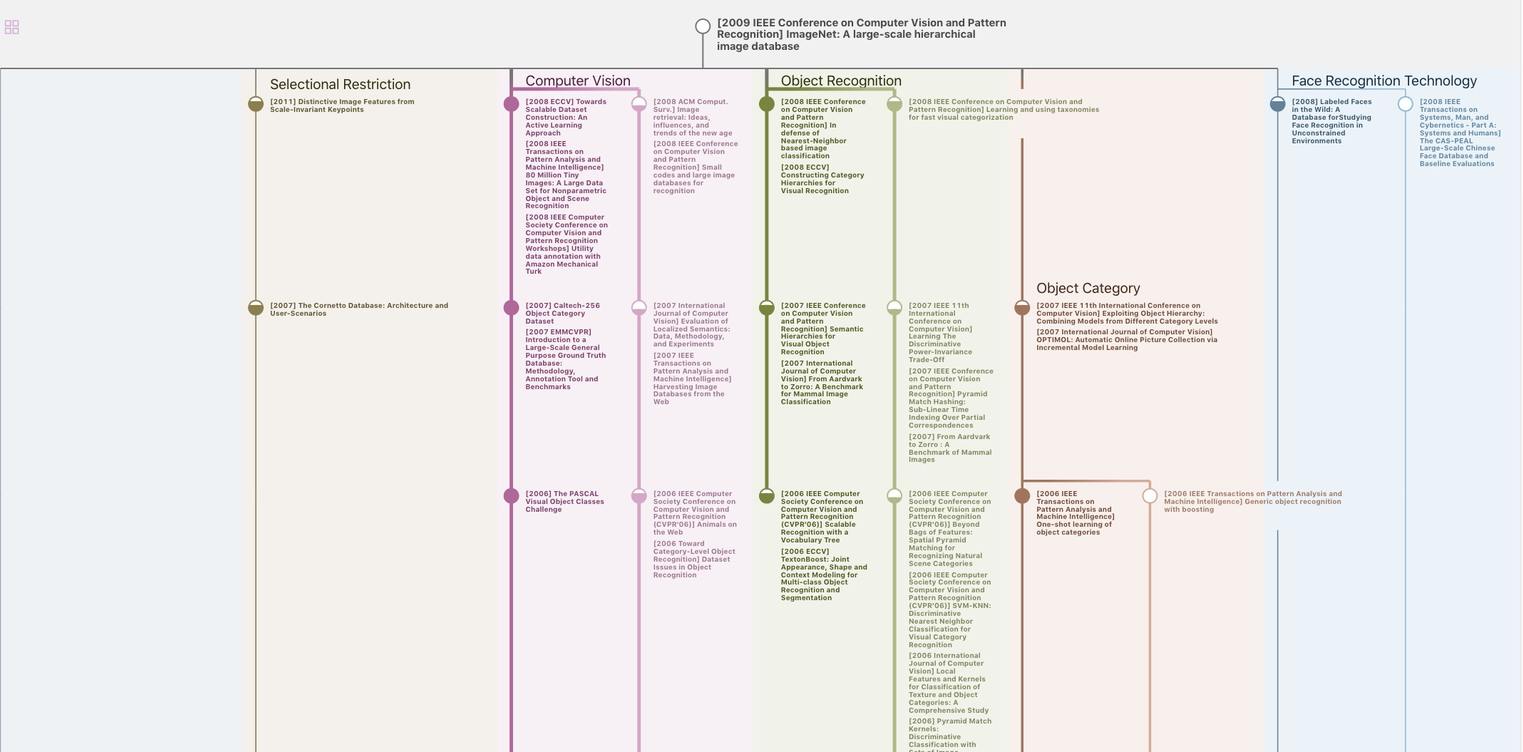
生成溯源树,研究论文发展脉络
Chat Paper
正在生成论文摘要