SAILER: Structure-aware Pre-trained Language Model for Legal Case Retrieval
PROCEEDINGS OF THE 46TH INTERNATIONAL ACM SIGIR CONFERENCE ON RESEARCH AND DEVELOPMENT IN INFORMATION RETRIEVAL, SIGIR 2023(2023)
摘要
Legal case retrieval, which aims to find relevant cases for a query case, plays a core role in the intelligent legal system. Despite the success that pre-training has achieved in ad-hoc retrieval tasks, effective pre-training strategies for legal case retrieval remain to be explored. Compared with general documents, legal case documents are typically long text sequences with intrinsic logical structures. However, most existing language models have difficulty understanding the long-distance dependencies between different structures. Moreover, in contrast to the general retrieval, the relevance in the legal domain is sensitive to key legal elements. Even subtle differences in key legal elements can significantly affect the judgement of relevance. However, existing pre-trained language models designed for general purposes have not been equipped to handle legal elements. To address these issues, in this paper, we propose SAILER, a new Structure-Aware pre-traIned language model for LEgal case Retrieval. It is highlighted in the following three aspects: (1) SAILER fully utilizes the structural information contained in legal case documents and pays more attention to key legal elements, similar to how legal experts browse legal case documents. (2) SAILER employs an asymmetric encoder-decoder architecture to integrate several different pre-training objectives. In this way, rich semantic information across tasks is encoded into dense vectors. (3) SAILER has powerful discriminative ability, even without any legal annotation data. It can distinguish legal cases with different charges accurately. Extensive experiments over publicly available legal benchmarks demonstrate that our approach can significantly outperform previous state-of-the-art methods in legal case retrieval.
更多查看译文
关键词
Legal Case Retrieval,Dense Retrieval,Pre-training,Structure-aware
AI 理解论文
溯源树
样例
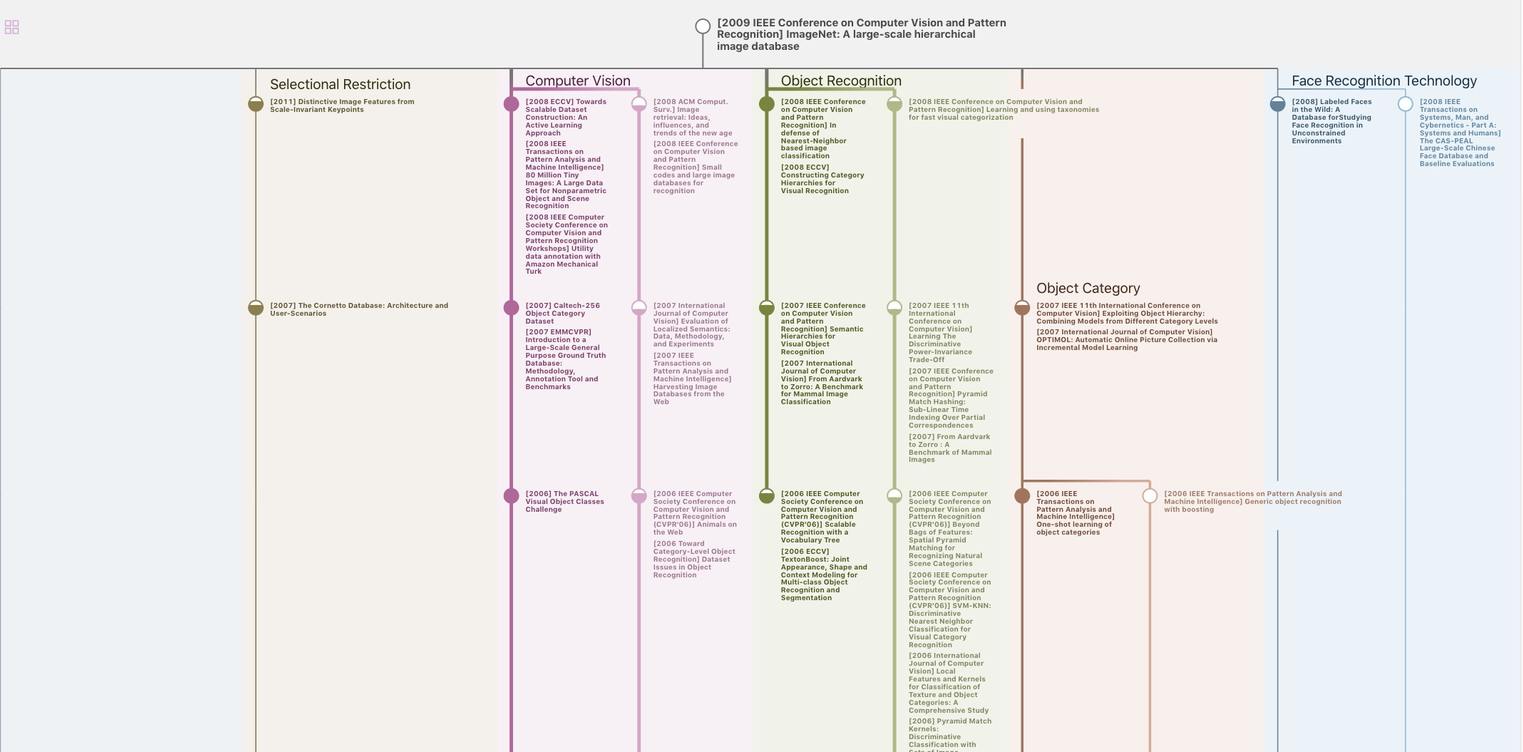
生成溯源树,研究论文发展脉络
Chat Paper
正在生成论文摘要