A data transfer method based on one dimensional convolutional neural network for cross-building load prediction
Energy(2023)
摘要
Load prediction is one of the basic tasks in energy system operation and management. With the development of data mining and artificial intelligence, data driven-based prediction models have been widely used. However, the accuracy of prediction model depends on large amounts of high-quality training data, that is difficult to obtain in practice, especially for new buildings. In order to solve the problem of data shortage, a building load data transfer model based on one dimensional convolutional neural network is proposed in this paper. The data transfer model can narrow the special features gap between the source domain and target domain, so that the source building data after transferring can be used to complete the prediction task on the target building. To verify the effectiveness of this strategy, the proposed model is applied in four scenes to transfer source building data to target building and then the prediction models are built using transferred data. The results show that the mean absolute percentage errors are reduced by 7.52%, 4.96%, 6.59% and 2.34%, respectively, compared with the model trained on the limited data in the four scenes. This work can provide guidance for the effective use of existing data resources.
更多查看译文
关键词
Building load, Prediction model, Transfer learning, Convolutional neural network
AI 理解论文
溯源树
样例
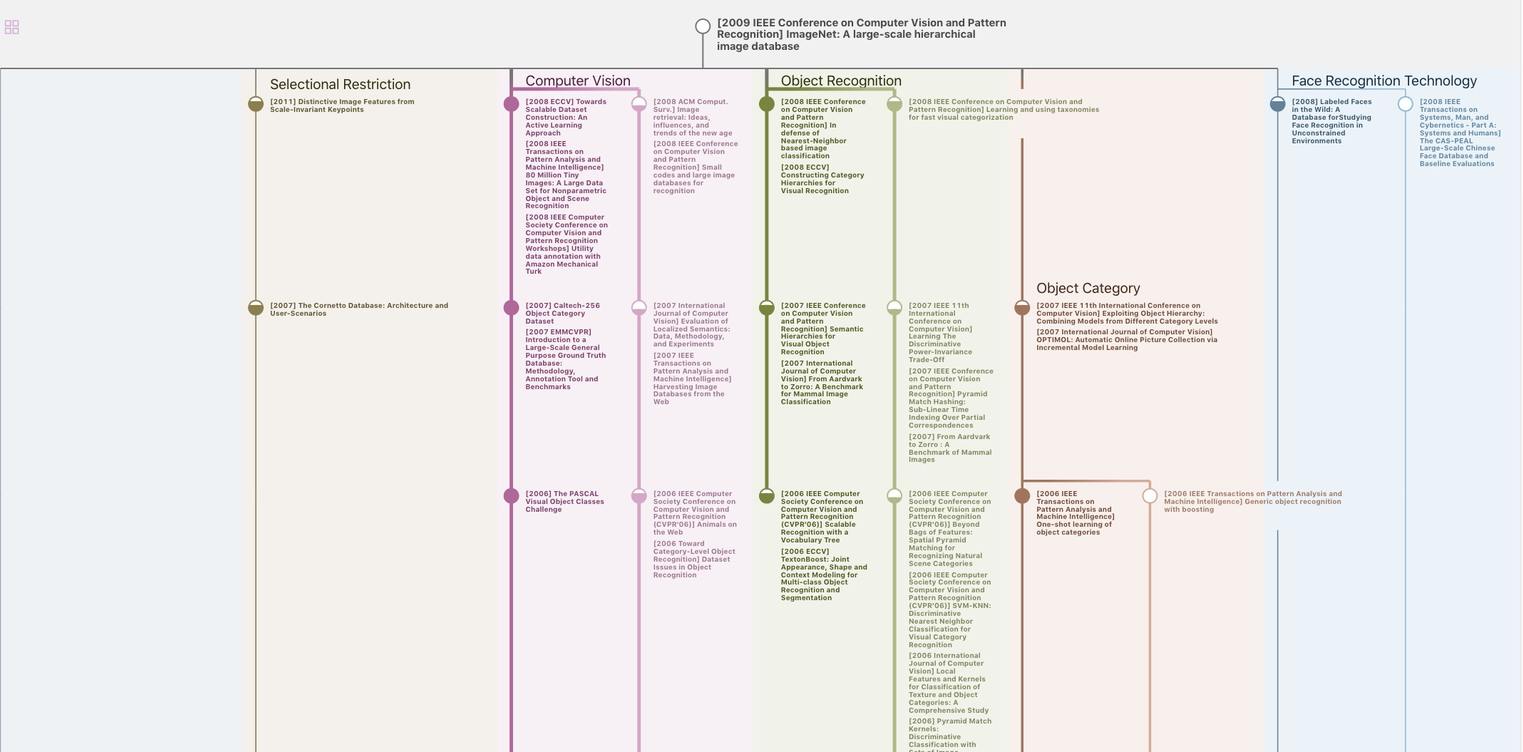
生成溯源树,研究论文发展脉络
Chat Paper
正在生成论文摘要