Rema-Net: An efficient multi-attention convolutional neural network for rapid skin lesion segmentation.
Computers in biology and medicine(2023)
摘要
For clinical treatment, the accurate segmentation of lesions from dermoscopic images is extremely valuable. Convolutional neural networks (such as U-Net and its numerous variants) have become the main methods for skin lesion segmentation in recent years. However, because these methods frequently have a large number of parameters and complicated algorithm structures, which results in high hardware requirements and long training time, it is difficult to effectively use them for fast training and segmentation tasks. For this reason, we proposed an efficient multi-attention convolutional neural network (Rema-Net) for rapid skin lesion segmentation. The down-sampling module of the network only uses a convolutional layer and a pooling layer, with spatial attention added to improve useful features. We also designed skip-connections between the down-sampling and up-sampling parts of the network, and used reverse attention operation on the skip-connections to strengthen segmentation performance of the network. We conducted extensive experiments on five publicly available datasets to validate the effectiveness of our method, including the ISIC-2016, ISIC-2017, ISIC-2018, PH2, and HAM10000 datasets. The results show that the proposed method reduced the number of parameters by nearly 40% when compared with U-Net. Furthermore, the segmentation metrics are significantly better than some previous methods, and the predictions are closer to the real lesion.
更多查看译文
关键词
Convolutional neural network,Skin lesion segmentation,Spatial attention,Reverse attention
AI 理解论文
溯源树
样例
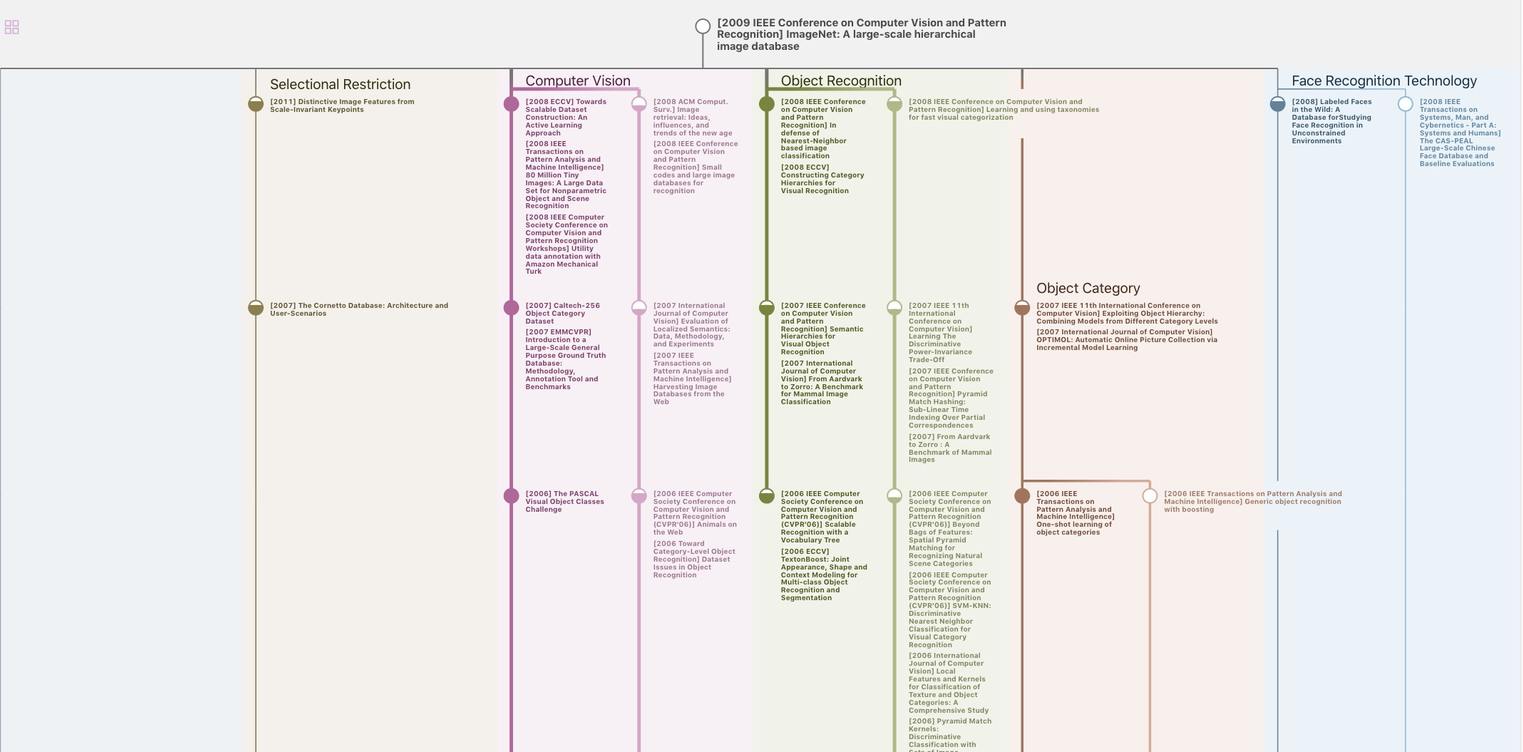
生成溯源树,研究论文发展脉络
Chat Paper
正在生成论文摘要