Contrastive Language, Action, and State Pre-training for Robot Learning
CoRR(2023)
摘要
In this paper, we introduce a method for unifying language, action, and state information in a shared embedding space to facilitate a range of downstream tasks in robot learning. Our method, Contrastive Language, Action, and State Pre-training (CLASP), extends the CLIP formulation by incorporating distributional learning, capturing the inherent complexities and one-to-many relationships in behaviour-text alignment. By employing distributional outputs for both text and behaviour encoders, our model effectively associates diverse textual commands with a single behaviour and vice-versa. We demonstrate the utility of our method for the following downstream tasks: zero-shot text-behaviour retrieval, captioning unseen robot behaviours, and learning a behaviour prior for language-conditioned reinforcement learning. Our distributional encoders exhibit superior retrieval and captioning performance on unseen datasets, and the ability to generate meaningful exploratory behaviours from textual commands, capturing the intricate relationships between language, action, and state. This work represents an initial step towards developing a unified pre-trained model for robotics, with the potential to generalise to a broad range of downstream tasks.
更多查看译文
关键词
contrastive language,learning,action,pre-training
AI 理解论文
溯源树
样例
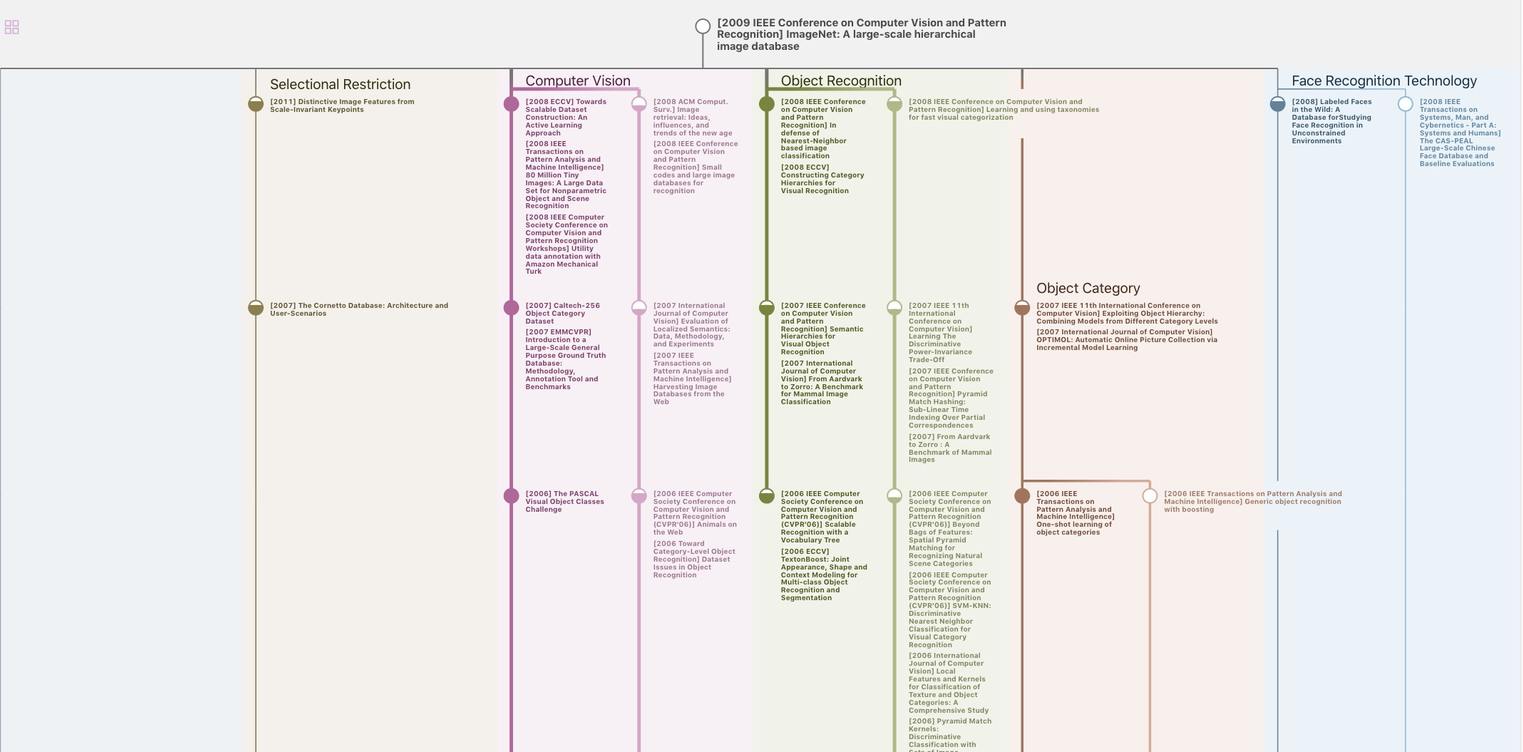
生成溯源树,研究论文发展脉络
Chat Paper
正在生成论文摘要