Enhancing Wind Power Forecast Precision via Multi-head Attention Transformer: An Investigation on Single-step and Multi-step Forecasting
2023 INTERNATIONAL JOINT CONFERENCE ON NEURAL NETWORKS, IJCNN(2023)
摘要
The main objective of this study is to propose an enhanced wind power forecasting (EWPF) transformer model for handling power grid operations and boosting power market competition. It helps reliable large-scale integration of wind power relies in large part on accurate wind power forecasting (WPF). The proposed model is evaluated for single-step and multi-step WPF, and compared with gated recurrent unit (GRU) and long short-term memory (LSTM) models on a wind power dataset. The results of the study indicate that the proposed EWPF transformer model outperforms conventional recurrent neural network (RNN) models in terms of time-series forecasting accuracy. In particular, the results reveal a minimum performance improvement of 5% and a maximum of 20% compared to LSTM and GRU. These results indicate that the EWPF transformer model provides a promising alternative for wind power forecasting and has the potential to significantly improve the precision of WPF. The findings of this study have implications for energy producers and researchers in the field of WPF.
更多查看译文
关键词
Wind Power Forecasting,LSTM,GRU,Transformer
AI 理解论文
溯源树
样例
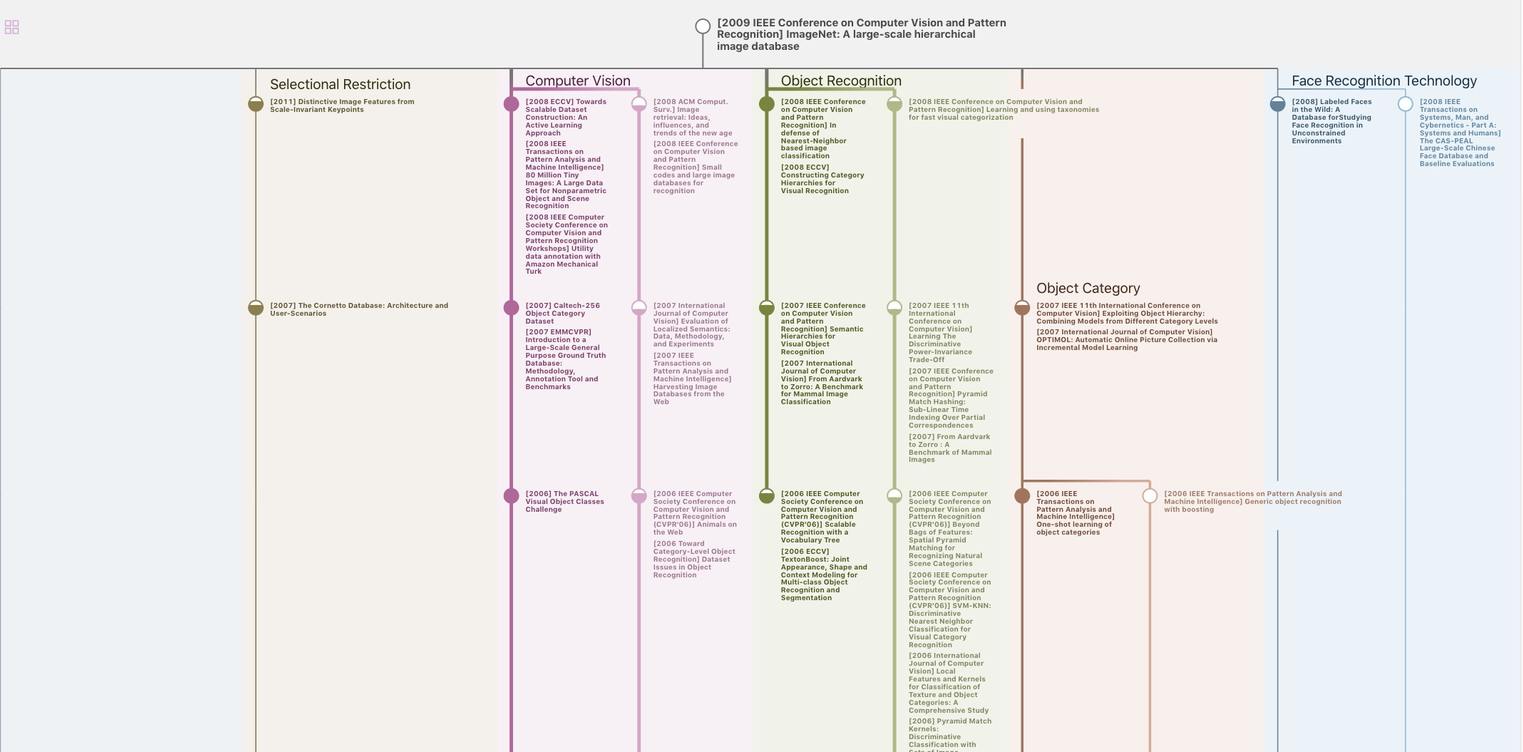
生成溯源树,研究论文发展脉络
Chat Paper
正在生成论文摘要