Multi-level uncertainty aware learning for semi-supervised dental panoramic caries segmentation
Neurocomputing(2023)
Abstract
Dental caries segmentation based on oral panoramic medical images is a demanding medical task. However, it is challenging to determine imaging diagnosis results as the actual pathological changes under certain conditions, especially in the suspected pathological locations that distinguish the artifacts from the lesions. This paper proposes introducing the semi-supervised learning framework to redistribute the collected cases to set up exact lesion areas and divide suspected areas with uncertainties into unlabeled data instead of reducing dataset labeling costs. Consistent regularization learning allows us to exploit the feature of ambiguous lesions to optimize decision boundaries. Unfortunately, the labels generated by the general SSL method for fuzzy regions are volatile, especially encountering no specific morphological rules and various scales that fluctuate more seriously, making a devastating impact on the regularization training relying on steady prediction. For such a challenge, we consider places that always form stable predictions under various disorders are often highly deterministic. Therefore, we propose introducing multi-level disturbances, including noise, iterative, and multi-scale disturbances. We simultaneously present in-depth supervision training on the multi-layer decoder to form stable and consistent multi-scale intermediate predictions, which significantly enrich the number of samples gathered in the following integration process. Then, Monte Carlo sampling is applied to integrate multi-level prediction results to assemble a more robust uncertainty mask map, which gradually clarifies the inter-class feature representation of actual lesions and artifacts. The extended experiment shows that we have successfully and effectively improved the performance of the caries segmentation task by using such uncertain lesion sample features. The dataset is now public, and the code is available at https://github.com/Zzz512/MLUA. (c) 2023 Elsevier B.V. All rights reserved.
MoreTranslated text
Key words
Medical image,Uncertainty perception,Dental lesion segmentation,Multi-level integration
AI Read Science
Must-Reading Tree
Example
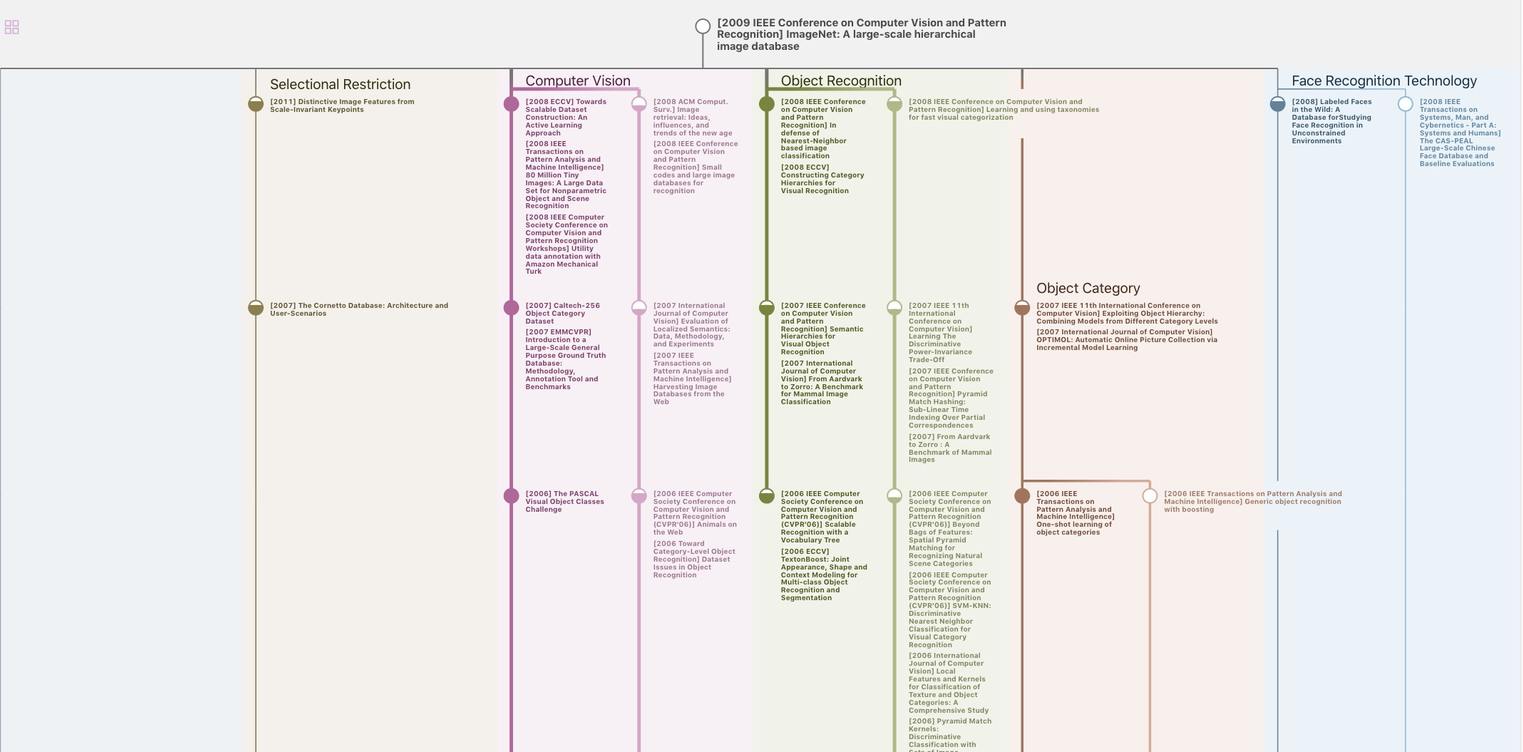
Generate MRT to find the research sequence of this paper
Chat Paper
Summary is being generated by the instructions you defined