Biomechanics Digital Twin: Markerless Joint Acceleration Prediction Using Machine Learning and Computer Vision
2023 Future of Educational Innovation-Workshop Series Data in Action(2023)
摘要
For athletes, coaches, or rehabilitation patients, the systems currently used to perform biomechanical studies and the dependence on technical experts for interpreting analyses and results can limit organizational, logistical, and economic resources. In this project, a Recurrent Neural Network model was created to predict human joint accelerations through the automatic digitalization of human body movement using video and acceleration sensors. The project aimed to prevent injuries and fractures in athletes and the elderly population because there is a lack of tools that predicts the risk of these traumas as a preventive method. Acceleration data was collected using Matlab mobile installed in cell phones attached to the arms and legs of volunteers doing physical tasks (walking, running, jumping). Experiments were video recorded, and machine learning models were trained using acceleration and video using Python libraries. After model evaluation, we observed that the selected model could predict the best on the XY axes and the worst on the Z axis, probably due to predicting a three-dimensional feature with a two-dimensional input. A biomechanical Digital Twin was created by combining the information from wearable devices, computer vision, and machine learning algorithms. This tool was able to estimate human joint accelerations (up to an extent) during movements with more refinement; it can help to evaluate movement performance within exercises or tasks and aid in injury/fracture risk prediction.
更多查看译文
关键词
biometrics,machine learning,biomechanics,educational innovation,higher education
AI 理解论文
溯源树
样例
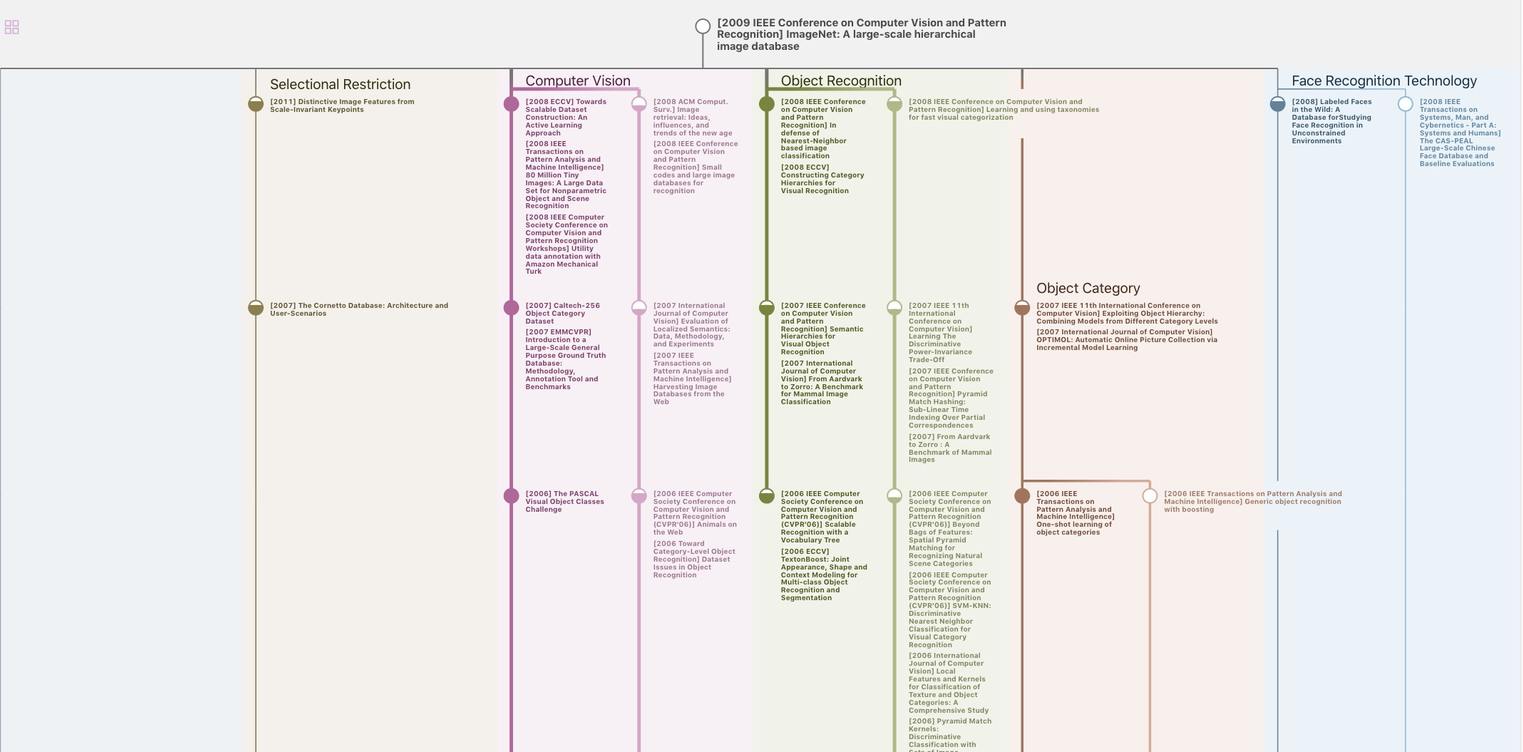
生成溯源树,研究论文发展脉络
Chat Paper
正在生成论文摘要