A Futures Quantitative Trading Strategy Based on a Deep Reinforcement Learning Algorithm
2023 IEEE 8th International Conference on Big Data Analytics (ICBDA)(2023)
摘要
Deep reinforcement learning (DRL) is a type of machine learning algorithm that has gained a lot of attention for its application in the financial field. Based on the proximal policy optimization algorithm (PPO) in deep reinforcement learning, this paper designs a trading strategy for the Chinese futures market, and realizes the end-to-end decision-making process from futures data to trading actions. Afterwards, using domestic rebar futures data, multiple historical data were selected for backtesting, and compared with traditional trading strategies. The results show that in the 12 selected test periods, 83.3% of the test periods are profitable, which is better than 33.3% of mean reversion (MR) and 25% of trend following (TF). It shows that the strategy proposed by us shows good adaptability when the futures market rises or falls compared with traditional methods, and can reduce losses through trading even when the market price changes significantly, thus increasing the return on investment.
更多查看译文
关键词
machine learning,Proximal Policy Optimization algorithm,deep reinforcement learning,financial trading
AI 理解论文
溯源树
样例
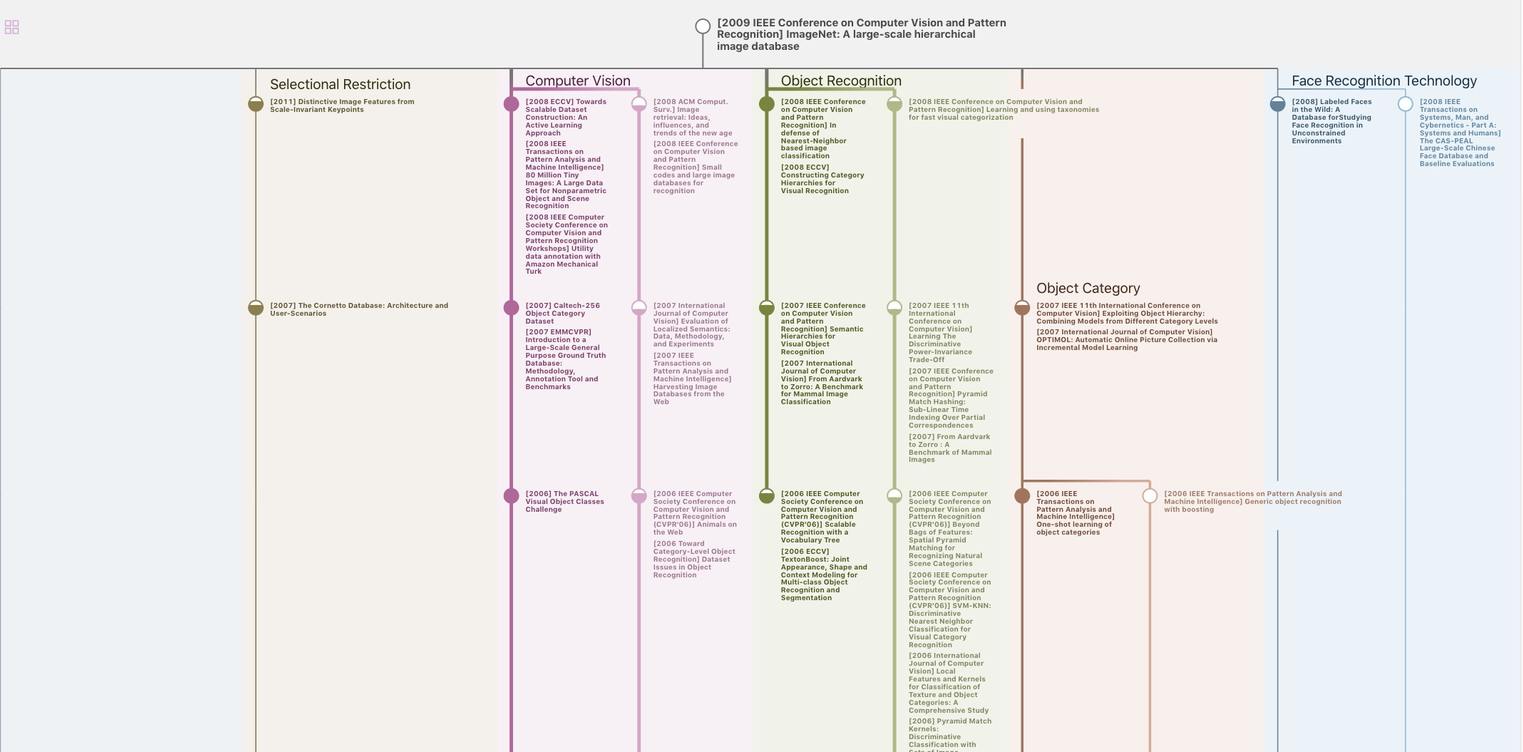
生成溯源树,研究论文发展脉络
Chat Paper
正在生成论文摘要