When attention is not enough to unveil a text’s author profile: Enhancing a transformer with a wide branch
Neural Computing and Applications(2023)
摘要
Author profiling (AP) is a highly relevant natural language processing (NLP) problem; it deals with predicting features of authors such as gender, age and personality traits. It is done by analyzing texts written by the authors themselves; take for instance documents such as books, articles, and more recently posts in social media platforms. In the present study, we focus in the latter, which is an scenario with a number of applications in marketing, security, health and others. Surprisingly, given the achievements of deep learning (DL) strategies on other NLP tasks, for AP DL architectures regularly underperform, left behind by classical machine learning (ML) approaches. In this study we show how a deep learning architecture based on transformers offers competitive results by exploiting a joint-intermediate fusion strategy called the Wide & Deep Transformer (WD-T). Our methodology implements a fusion of contextualized word vector representations and handcrafted features, by using a self-attention mechanism and a novel encoding technique that incorporates stylistic , topic , and personal information from authors. This allows for the creation of more accurate, fine-grained predictions. Our approach attained competitive performance against top-quartile results from the 2017–2019 editions at the Plagiarism analysis, Authorship identification, and Near-duplicate detection forum (PAN) in English and Spanish languages for gender and language variety predictions, and the Kaggle Myers–Briggs-type indicator (MBTI) dataset for personality forecasting . Our proposal consistently surpasses all other deep learning methods in PAN collections by as much as 2.4%, and up to 3.4% in the MBTI dataset. These results suggest that this DL strategy effectively addresses and improves upon the limitations of previous techniques and paves the way for new avenues of inquiry.
更多查看译文
关键词
Author profiling,Attention mechanisms,Deep neural networks,Deep learning fusion strategies,Domain-specific features,Wide and deep networks
AI 理解论文
溯源树
样例
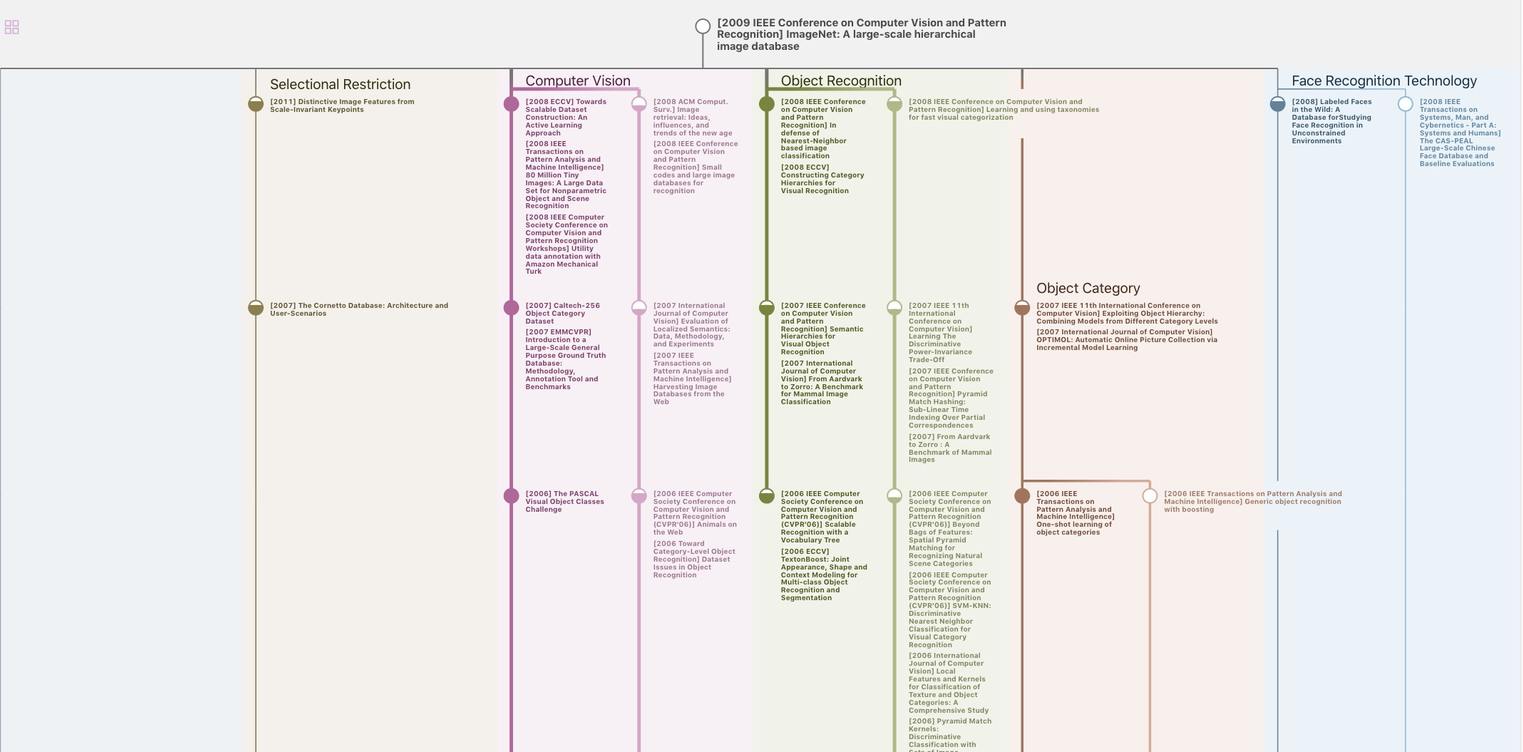
生成溯源树,研究论文发展脉络
Chat Paper
正在生成论文摘要