KaryoNet: Chromosome Recognition With End-to-End Combinatorial Optimization Network
IEEE transactions on medical imaging(2023)
摘要
Chromosome recognition is a critical way to diagnose various hematological malignancies and genetic diseases, which is however a repetitive and time-consuming process in karyotyping. To explore the relative relation between chromosomes, in this work, we start from a global perspective and learn the contextual interactions and class distribution features between chromosomes within a karyotype. We propose an end-to-end differentiable combinatorial optimization method, KaryoNet, which captures long-range interactions between chromosomes with the proposed Masked Feature Interaction Module (MFIM) and conducts label assignment in a flexible and differentiable way with Deep Assignment Module (DAM). Specially, a Feature Matching Sub-Network is built to predict the mask array for attention computation in MFIM. Lastly, Type and Polarity Prediction Head can predict chromosome type and polarity simultaneously. Extensive experiments on R-band and G-band two clinical datasets demonstrate the merits of the proposed method. For normal karyotypes, the proposed KaryoNet achieves the accuracy of 98.41% on R-band chromosome and 99.58% on G-band chromosome. Owing to the extracted internal relation and class distribution features, KaryoNet can also achieve state-of-the-art performances on karyotypes of patients with different types of numerical abnormalities. The proposed method has been applied to assist clinical karyotype diagnosis. Our code is available at: https://github.com/xiabc612/KaryoNet.
更多查看译文
关键词
Chromosome recognition,label assignment,transformer,attention mask
AI 理解论文
溯源树
样例
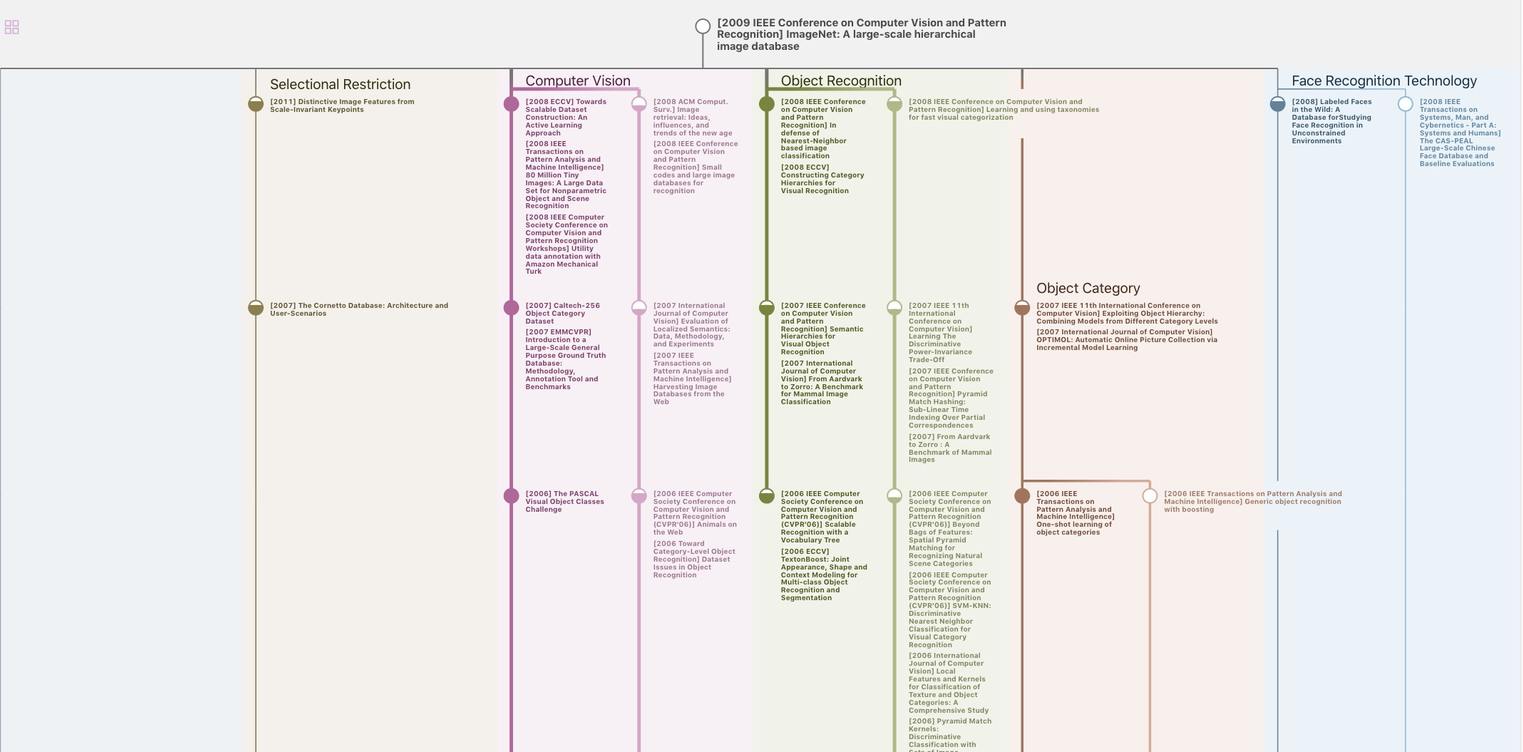
生成溯源树,研究论文发展脉络
Chat Paper
正在生成论文摘要