Homophily-Enhanced Self-Supervision for Graph Structure Learning: Insights and Directions.
IEEE transactions on neural networks and learning systems(2023)
摘要
Graph neural networks (GNNs) have recently achieved remarkable success on a variety of graph-related tasks, while such success relies heavily on a given graph structure that may not always be available in real-world applications. To address this problem, graph structure learning (GSL) is emerging as a promising research topic where task-specific graph structure and GNN parameters are jointly learned in an end-to-end unified framework. Despite their great progress, existing approaches mostly focus on the design of similarity metrics or graph construction, but directly default to adopting downstream objectives as supervision, which lacks deep insight into the power of supervision signals. More importantly, these approaches struggle to explain how GSL helps GNNs, and when and why this help fails. In this article, we conduct a systematic experimental evaluation to reveal that GSL and GNNs enjoy consistent optimization goals in terms of improving the graph homophily. Furthermore, we demonstrate theoretically and experimentally that task-specific downstream supervision may be insufficient to support the learning of both graph structure and GNN parameters, especially when the labeled data are extremely limited. Therefore, as a complement to downstream supervision, we propose homophily-enhanced self-supervision for GSL (HES-GSL), a method that provides more supervision for learning an underlying graph structure. A comprehensive experimental study demonstrates that HES-GSL scales well to various datasets and outperforms other leading methods. Our code will be available in https://github.com/LirongWu/Homophily-Enhanced-Self-supervision.
更多查看译文
关键词
Deep learning,graph neural networks (GNNs),graph structure learning (GSL),self-supervised learning
AI 理解论文
溯源树
样例
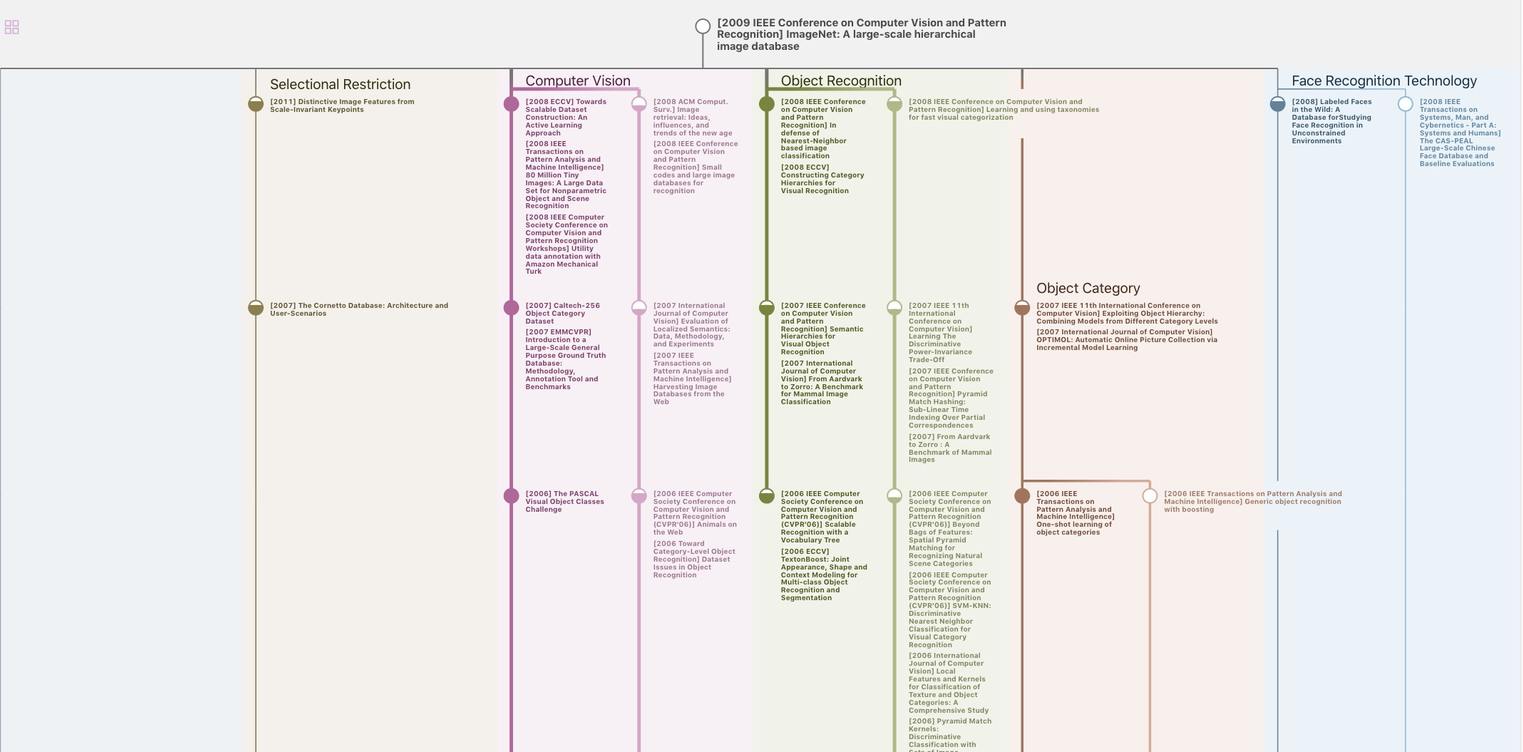
生成溯源树,研究论文发展脉络
Chat Paper
正在生成论文摘要