Quantile partially linear additive model for data with dropouts and an application to modeling cognitive decline
Statistics in medicine(2023)
摘要
The National Alzheimer's Coordinating Center Uniform Data Set includes test results from a battery of cognitive exams. Motivated by the need to model the cognitive ability of low-performing patients we create a composite score from ten tests and propose to model this score using a partially linear quantile regression model for longitudinal studies with non-ignorable dropouts. Quantile regression allows for modeling non-central tendencies. The partially linear model accommodates nonlinear relationships between some of the covariates and cognitive ability. The data set includes patients that leave the study prior to the conclusion. Ignoring such dropouts will result in biased estimates if the probability of dropout depends on the response. To handle this challenge, we propose a weighted quantile regression estimator where the weights are inversely proportional to the estimated probability a subject remains in the study. We prove that this weighted estimator is a consistent and efficient estimator of both linear and nonlinear effects.
更多查看译文
关键词
longitudinal data,missing data,quantile regression,semiparametric
AI 理解论文
溯源树
样例
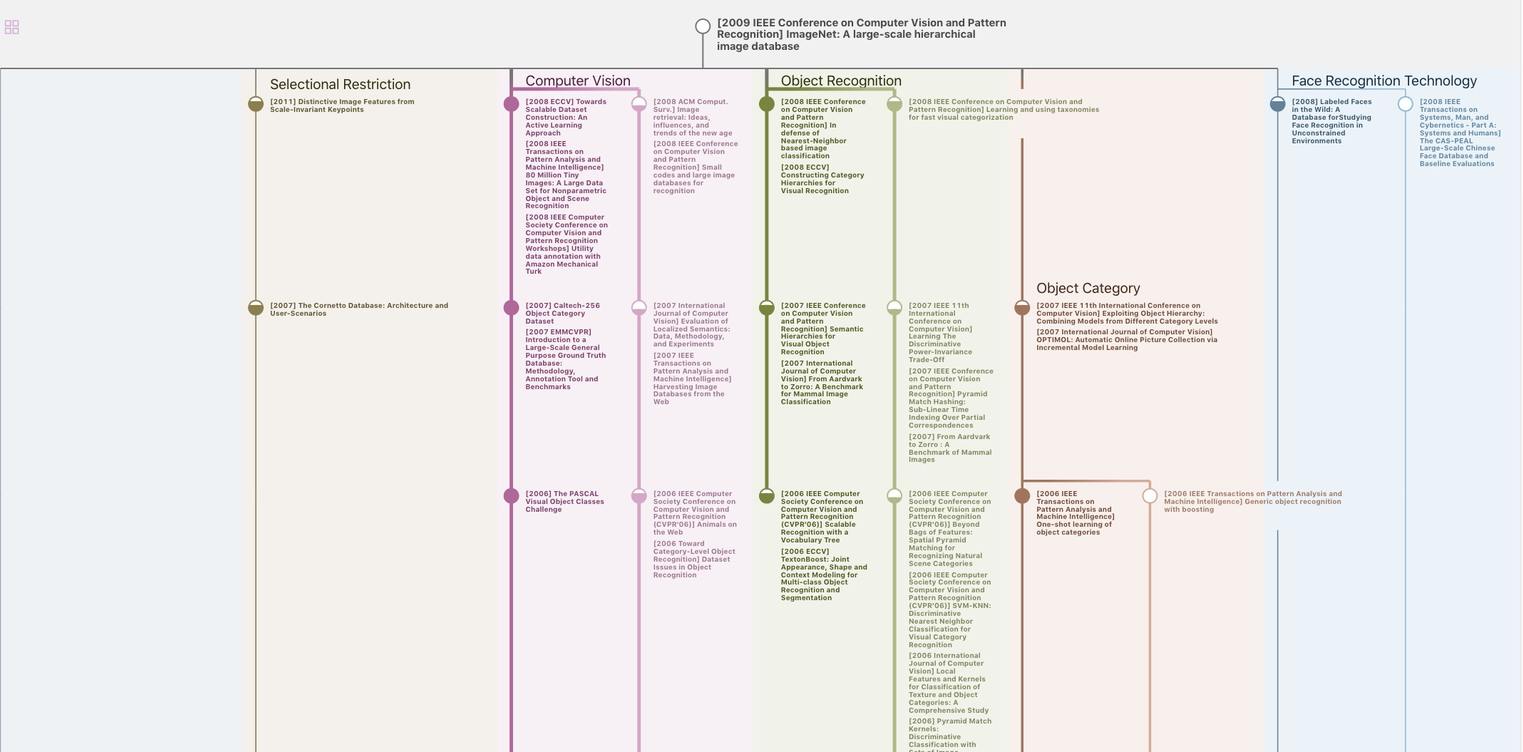
生成溯源树,研究论文发展脉络
Chat Paper
正在生成论文摘要