Regret-Minimizing Double Oracle for Extensive-Form Games
CoRR(2023)
摘要
By incorporating regret minimization, double oracle methods have demonstrated rapid convergence to Nash Equilibrium (NE) in normal-form games and extensive-form games, through algorithms such as online double oracle (ODO) and extensive-form double oracle (XDO), respectively. In this study, we further examine the theoretical convergence rate and sample complexity of such regret minimization-based double oracle methods, utilizing a unified framework called Regret-Minimizing Double Oracle. Based on this framework, we extend ODO to extensive-form games and determine its sample complexity. Moreover, we demonstrate that the sample complexity of XDO can be exponential in the number of information sets $|S|$, owing to the exponentially decaying stopping threshold of restricted games. To solve this problem, we propose the Periodic Double Oracle (PDO) method, which has the lowest sample complexity among all existing double oracle methods, being only polynomial in $|S|$. Empirical evaluations on multiple poker and board games show that PDO achieves significantly faster convergence than previous double oracle algorithms and reaches a competitive level with state-of-the-art regret minimization methods.
更多查看译文
关键词
games,regret-minimizing,extensive-form
AI 理解论文
溯源树
样例
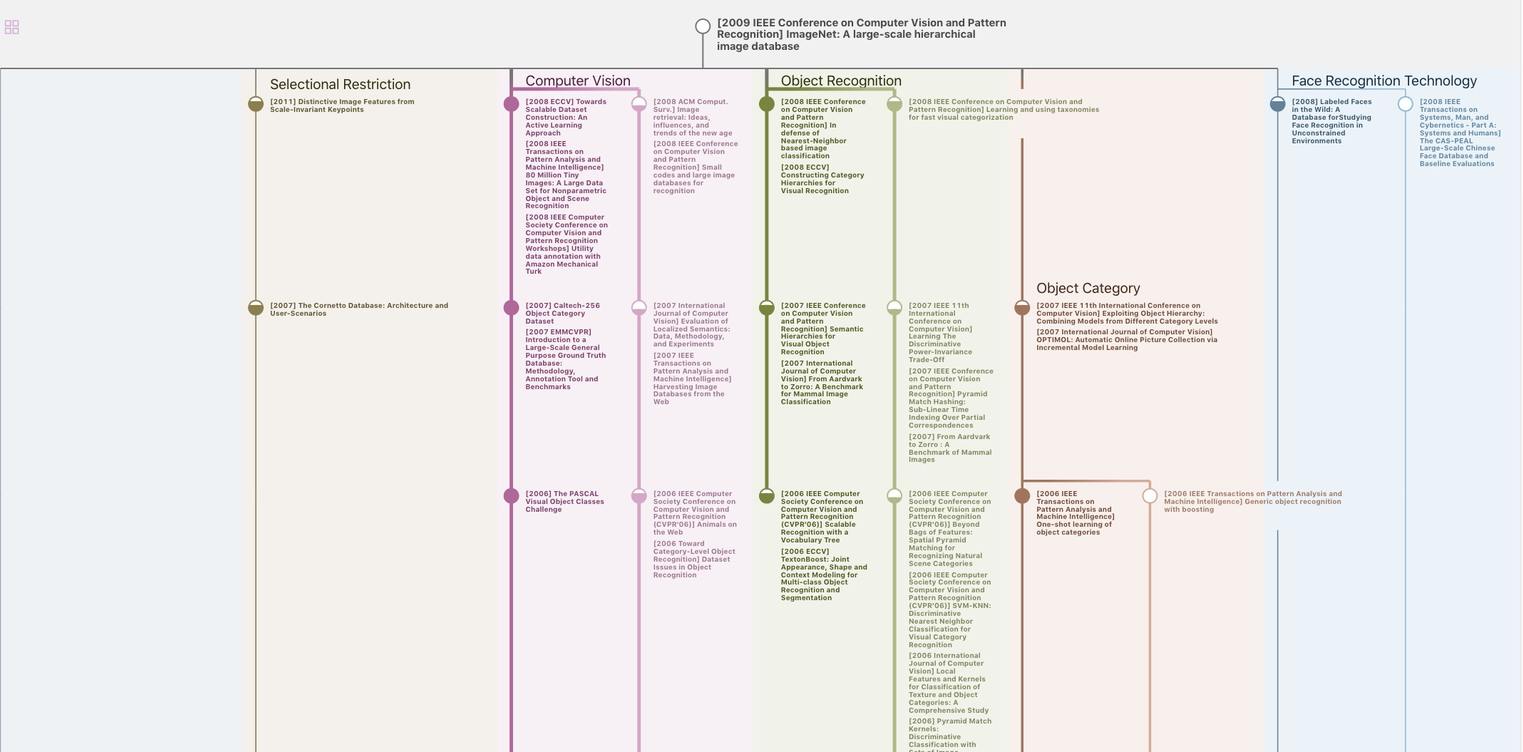
生成溯源树,研究论文发展脉络
Chat Paper
正在生成论文摘要