Stronger correlation of music features with brain signals predicts increased levels of enjoyment
2023 IEEE Applied Sensing Conference (APSCON)(2023)
摘要
Music recommendation systems struggle with predicting the aesthetic responses of listeners based solely on acoustic characteristics, which are dependent on the listener’s perception. This research correlates acoustic music features with brain responses to report the neural aesthetic hypothesis that the intensity of an aesthetic experience can be decoded based on the degree of correlation to brain responses. We employ hybrid encoding-decoding model (Canonical Correlation Analysis) to identify music features that maximally covary with brain responses. EEG signals of 20 participants are analyzed while they listen to 12 songs and mark their enjoyment on a scale of 1 to 5. Firstly, 18 acoustic features are extracted from music signals and transformed into the first principal component (PC1). In addition, two other features used for analysis are root mean square (RMS) and Spectral Flux (Flux). The first principal canonical component (CC1) with PC1 determines significant (p<0.05) evidence of correlating with brain responses that increasing correlation reflects increased enjoyment. We consider each participant’s average CC1 values and enjoyment rating over all 12 songs, followed by plotting a correlation graph to decode the relationship. We observe a significant (p<0.05) positive linear correlation with increasing CC1 scores of PC1 features against increased enjoyment rating. PC1 shows the maximum Pearson correlation (r = 0.48, p = 0.03). In addition, we segregate the brain responses based on low (1,2) and high (3,4) enjoyment ratings and find that higher CC1 values correspond to brain responses of high enjoyment and low values to low enjoyment in all three features. Our experiments reveal that Canonical correlation reflects music-induced pleasure and can be employed in EEG-enabled headphones to decode the user experience, leading to better recommendations.
更多查看译文
关键词
EEG,Aesthetic,Music,CCA
AI 理解论文
溯源树
样例
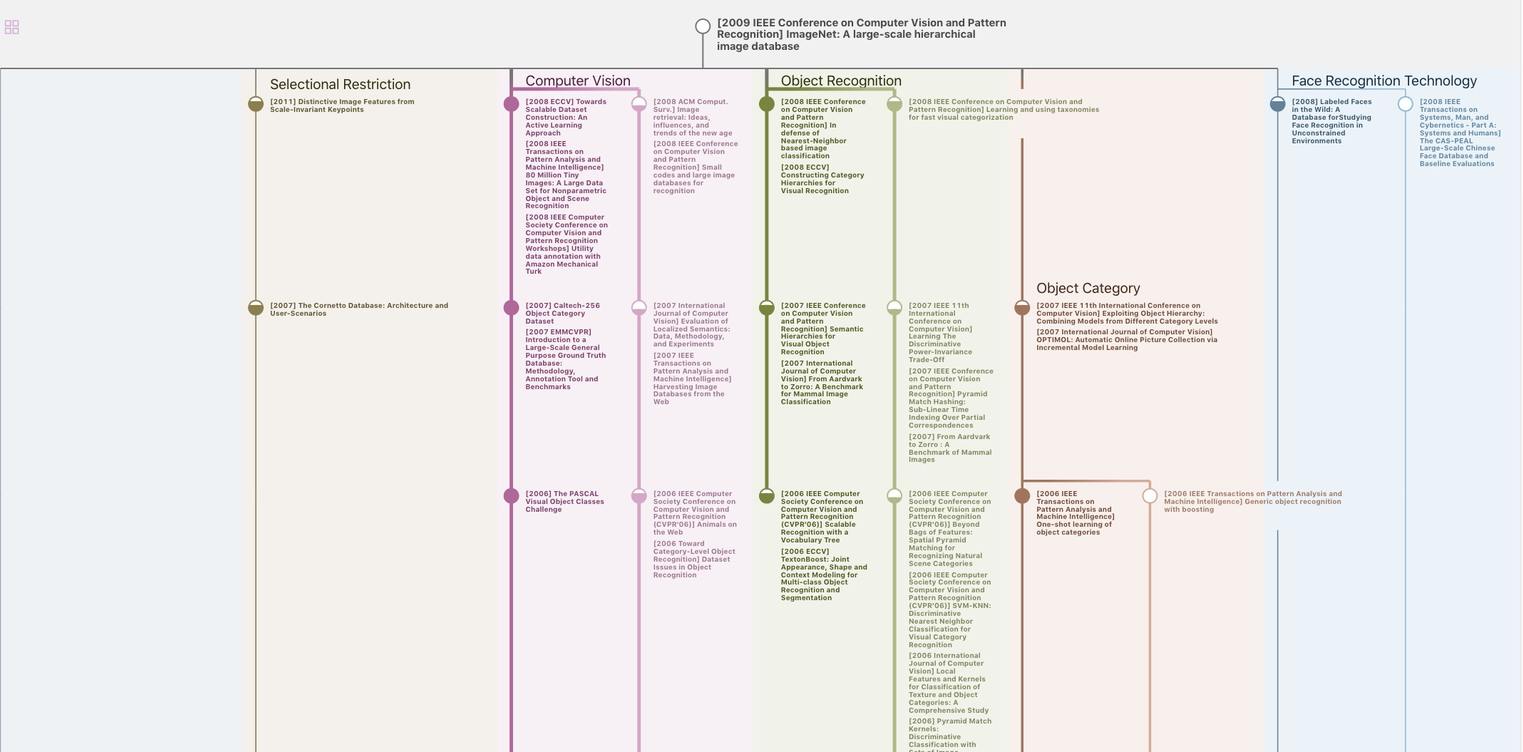
生成溯源树,研究论文发展脉络
Chat Paper
正在生成论文摘要