Readiness for Artificial Intelligence in Biobanking.
Biopreservation and biobanking(2023)
摘要
Biopreservation and BiobankingVol. 21, No. 2 EditorialFree AccessReadiness for Artificial Intelligence in BiobankingGregory H. Grossman and Marianne K. HendersonGregory H. GrossmanAdvancing Sight Network, Birmingham, Alabama, USA.Search for more papers by this author and Marianne K. HendersonAddress correspondence to: Marianne K. Henderson, MS, CPC, National Cancer Institute, NIH, 9609 Medical Center Drive, 7E442, Rockville, MD 20850, USA E-mail Address: [email protected]National Cancer Institute, NIH, Bethesda, Maryland, USA.Search for more papers by this authorPublished Online:17 Apr 2023https://doi.org/10.1089/bio.2023.29121.editorialAboutSectionsPDF/EPUB Permissions & CitationsPermissionsDownload CitationsTrack CitationsAdd to favorites Back To Publication ShareShare onFacebookTwitterLinked InRedditEmail The recent launch of the language and text processing artificial intelligence (AI) platform ChatGPT from OpenAI has markedly raised interest in AI and signaled a new era of machine and deep learning. AI can be defined as an automated software system that can ingest, compile, and analyze data and domain knowledge to present informed, context-specific outputs.As contributors to the information management (data, systems, and networks) section of the International Society for Biological and Environmental Repositories (ISBER) Best Practices 5th edition, we have been following the advances and implementation of AI in health care, research, and biobanking with great anticipation. We also see the same excitement from the ISBER membership, as illustrated by the active discussions on the ISBER Open Forum.There are many different types and categories of deep and machine learning AI platforms based on their engineering and function. Although AI was described in computer science as early as the 1950s and the earliest NIH AI in Medicine conference was held in 1975,1 it is only recently that some of the technological limitations of its use have been overcome, including data availability, to allow it to be more widely applied. We routinely encounter AI-driven programs in our daily lives, although we may not be fully aware of their presence over the past few years.Examples range from chatbots to voice assistants such as Amazon's Alexa or Apple's Siri, to screening-based algorithms, such as those used in mammography, that detect patterns that may signal abnormalities to be more closely examined. Imaging algorithms benefit from learning through continued use, to refine performance. Recently, AI has become integrated more widely into medical and scientific practices. An AI program to screen for diabetic retinopathy by interpreting clinical images was successfully modeled in India and the United States,2 with screening implemented in a country-wide health system in Thailand.3Furthermore and importantly, some approved AI screening programs have matured enough that they are autonomous, meaning that human oversight of clinical diagnosis is not required.4 These examples highlight AI's unique ability to decipher hidden patterns in data, build predictive models, and deliver actionable recommendations.Much of our field anticipates that the inevitable implementation of AI in biobanking will have profound and positive impacts, with the overall outcome of increasing specimen/data utilization, greater repository sustainability, and increased speed of scientific discovery. AI has the potential to impact all areas of biobanking, from recruiting donors, improving biobank operations, developing educational and training materials for new biobankers, increasing sample use, improving data annotation, and faster deposition of ethically-protected data. Industries across the globe are looking toward AI to help optimize operations, and biobanking will be no different.As examples, we can imagine using natural language processors to help our biobanks and their resources to market more broadly to the scientific community; or interpreting and converting free text comments or voice notes into standard categorical data fields that can facilitate data interoperability. AI in biobanking could create the draft text for consenting, standard operating procedures or operational documentation, write analytical code for samples and data, and write scripts for data deposition. We can also see its impact on accelerating the availability and analysis of large data sets, such as in the case of whole genome sequencing.Although the beneficial aspects and opportunities hold much promise, integrating AI within the biobanking continuum poses challenges and risks. What looks to be the most salient risk of implementing AI in biobanking is not the malicious actions of a self-aware superintelligence portrayed in dystopian science fiction, but something more closely aligned to an inherent human trait—bias. Data bias in AI parallels the traditional computer science concept of “garbage in, garbage out,” in which inaccurate, erroneous, or absent input leads to false or “garbage” output data (including actionable reports or decisions). It is here that we can make the most impact at this time so that biobanking is “ready” for AI applications.The continued push for incorporating and utilizing principles such as Open Science, FAIR (Findability, Accessibility, Interoperability, and Reuse), or DwC (Darwin Core), data standards such as SPREC (Standard PREanalytical Code), and guidelines such as ISO 8000 (data quality), will help to ensure robust, high-quality, and ethical data.5–8 There is also a greater need for repositories to plan for the interoperability of their data and systems to be able to integrate with AI applications in the cloud, across networks, or on virtual platforms. Another form of bias that presents a challenge is algorithmic bias.AI algorithmic bias tends to highly reflect human bias, whereby the system delivers erroneous conclusions or predictions, without showing ambiguity or confidence limits in its delivery, which can be ethically problematic and ultimately damaging to groups of people or sensitive species. This threat underscores the need for AI programmers to develop and incorporate robust ethical guidelines/guardrails to protect from harm to environments, species, and populations. The unintended harm to populations and society was called out by an Open Letter to AI developers to take a pause in their training and release of more powerful AI systems than GPT-4.9Our biobanking subject matter expert role will be needed to influence the development of these guardrails as AI methods and algorithms evolve. Two additional pillars that must be forefront in AI development include the reliability that outputs provided can be trusted and that security measures from hacking and breaches of personal or sensitive data are implemented.This most exciting and challenging era of biobanking awaits our community. We need to educate ourselves on the possible uses and impacts of deep learning, machine learning, and natural language processing applications through engagements with AI experts. Through these engagements and the formation of biobanking workgroups, we will contribute to ethically-sound AI tools and practices for biobanking and data sharing. Perhaps the most exciting aspect of AI in biobanking is that this nascent technology will have emergent and exponential benefits, accelerating our impact on the discovery and implementation of new knowledge to protect the health of our populations and the environment.To kick off further discussions, we are developing a webinar global cast on AI's impact on biobanking as part of ISBER's Webinar Planning Task Force. We look forward to continuing this dialogue, through the active engagement of members of the biobanking community and the implementation of AI in biobanking in the very near future.References1. Kaul V, Enslin S, Gross SA. History of artificial intelligence in medicine. Gastrointest Endosc 2020;92(4):807–812; doi: 10.1016/j.gie.2020.06.040 Crossref, Medline, Google Scholar2. Gulshan V, Peng L, Coram M, et al. Development and validation of a deep learning algorithm for detection of diabetic retinopathy in retinal fundus photographs. JAMA 2016;316(22):2402–2410; doi: 10.1001/jama.2016.17216 Crossref, Medline, Google Scholar3. Ruamviboonsuk P, Tiwari R, Sayres R, et al. Real-time diabetic retinopathy screening by deep learning in a multisite national screening programme: A prospective interventional cohort study. Lancet Digit Health 2022;4(4):e235–e244; doi: 10.1016/S2589-7500(22)00017-6 Crossref, Medline, Google Scholar4. Abràmoff MD, Lavin PT, Birch M, et al. Pivotal trial of an autonomous AI-based diagnostic system for detection of diabetic retinopathy in primary care offices. NPJ Digital Med 2018;1:39; doi: 10.1038/s41746-018-0040-6 Crossref, Medline, Google Scholar5. Wilkinson M, Dumontier M, Aalbersberg I, et al. The FAIR Guiding Principles for scientific data management and stewardship. Sci Data 2016;3:160018; doi: 10.1038/sdata.2016.18 Crossref, Medline, Google Scholar6. Vicente-Saez R, Martinex-Fuentes C. Open Science now: A systematic literature review for an integrated definition. J Bus Res 2018;88:428–436; doi: 10.1016/j.jbusres.2017.12.043 Crossref, Google Scholar7. Biodiversity Information Standards (TDWG). Available from: www.tdwg.org, tdwg.org [Last accessed: March 22, 2023]. Google Scholar8. Lehmann S, Guadagni F, Moore H, et al. Standard preanalytical coding for biospecimens: Review and implementation of the Sample PREanalytical Code (SPREC). Biopreserv Biobank 2012;10(4):366–374; doi: 10.1089/bio.2012.0012 Link, Google Scholar9. 5 Future of Life Institute. Pause Giant AI Experiments: An Open Letter. Available from: https://futureoflife.org/open-letter/pause-giant-ai-experiments/ [Last accessed: April 7, 2023]. Google ScholarFiguresReferencesRelatedDetails Volume 21Issue 2Apr 2023 InformationCopyright 2023, Mary Ann Liebert, Inc., publishersTo cite this article:Gregory H. Grossman and Marianne K. Henderson.Readiness for Artificial Intelligence in Biobanking.Biopreservation and Biobanking.Apr 2023.119-120.http://doi.org/10.1089/bio.2023.29121.editorialPublished in Volume: 21 Issue 2: April 17, 2023PDF download
更多查看译文
关键词
biobanking,artificial intelligence,readiness
AI 理解论文
溯源树
样例
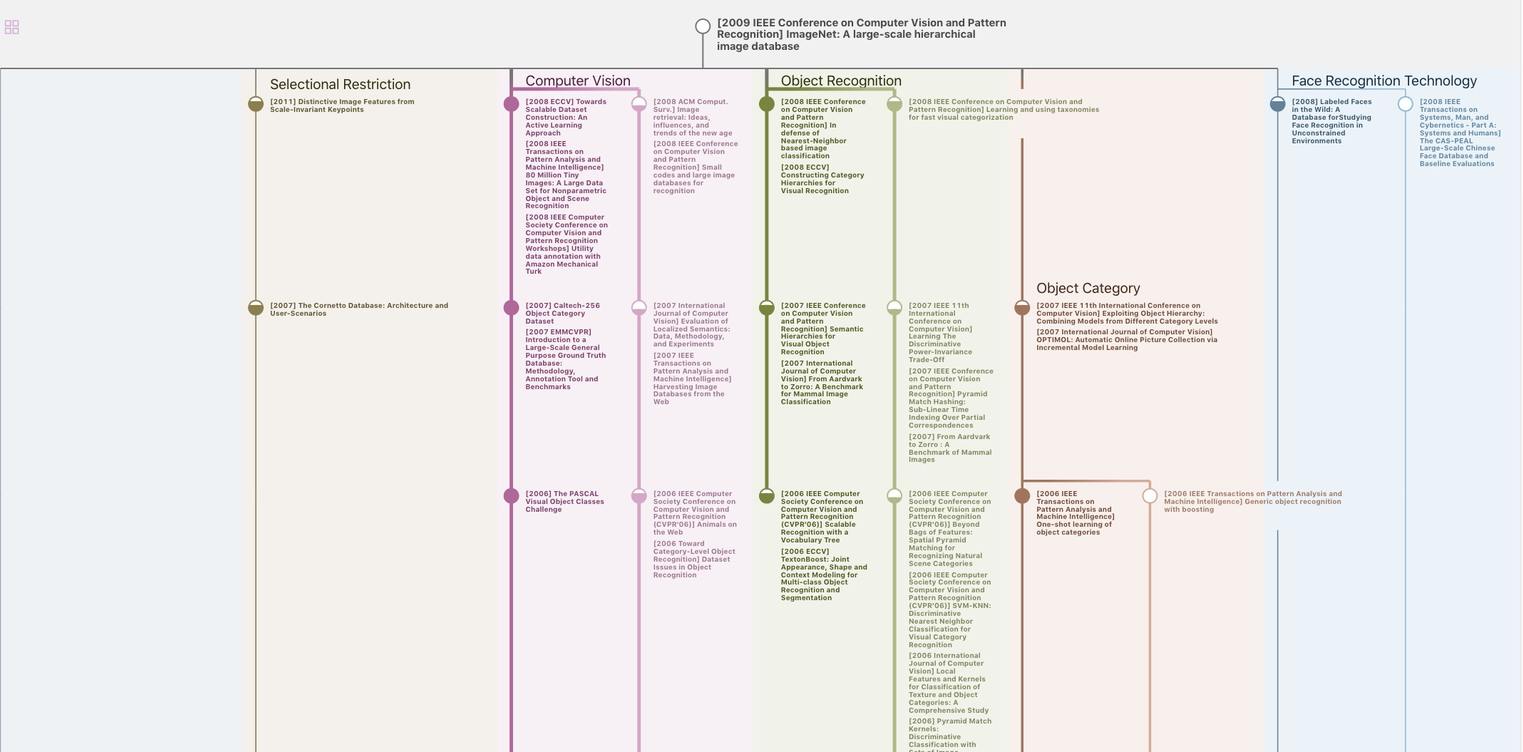
生成溯源树,研究论文发展脉络
Chat Paper
正在生成论文摘要