Comma Selection Outperforms Plus Selection on OneMax with Randomly Planted Optima
CoRR(2023)
摘要
It is an ongoing debate whether and how comma selection in evolutionary algorithms helps to escape local optima. We propose a new benchmark function to investigate the benefits of comma selection: OneMax with randomly planted local optima, generated by frozen noise. We show that comma selection (the (1,lambda) EA) is faster than plus selection (the (1 +lambda) EA) on this benchmark, in a fixed-target scenario, and for offspring population sizes lambda for which both algorithms behave differently. For certain parameters, the (1,lambda) EA finds the target in T(.. ln..) evaluations, with high probability (w.h.p.), while the (1 +lambda) EA w.h.p. requires almost Theta((n ln n)(2)) evaluations. We further show that the advantage of comma selection is not arbitrarily large: w.h.p. comma selection outperforms plus selection at most by a factor of O (n ln n) for most reasonable parameter choices. We develop novel methods for analysing frozen noise and give powerful and general fixed-target results with tail bounds that are of independent interest.
更多查看译文
关键词
selection
AI 理解论文
溯源树
样例
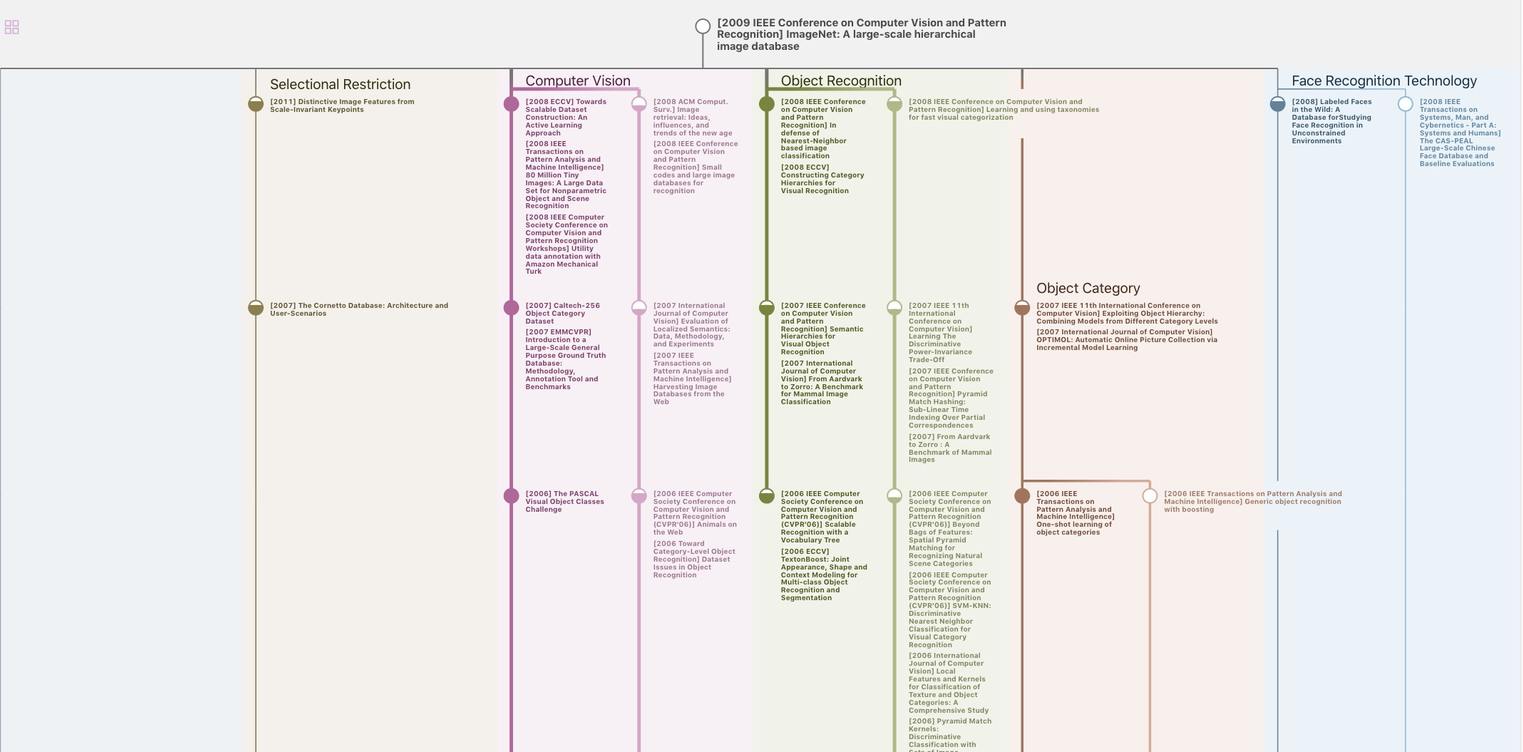
生成溯源树,研究论文发展脉络
Chat Paper
正在生成论文摘要