Disentangling Neuron Representations with Concept Vectors
CoRR(2023)
摘要
Mechanistic interpretability aims to understand how models store representations by breaking down neural networks into interpretable units. However, the occurrence of polysemantic neurons, or neurons that respond to multiple unrelated features, makes interpreting individual neurons challenging. This has led to the search for meaningful vectors, known as concept vectors, in activation space instead of individual neurons. The main contribution of this paper is a method to disentangle polysemantic neurons into concept vectors encapsulating distinct features. Our method can search for fine-grained concepts according to the user's desired level of concept separation. The analysis shows that polysemantic neurons can be disentangled into directions consisting of linear combinations of neurons. Our evaluations show that the concept vectors found encode coherent, human-understandable features.
更多查看译文
关键词
representations,concept
AI 理解论文
溯源树
样例
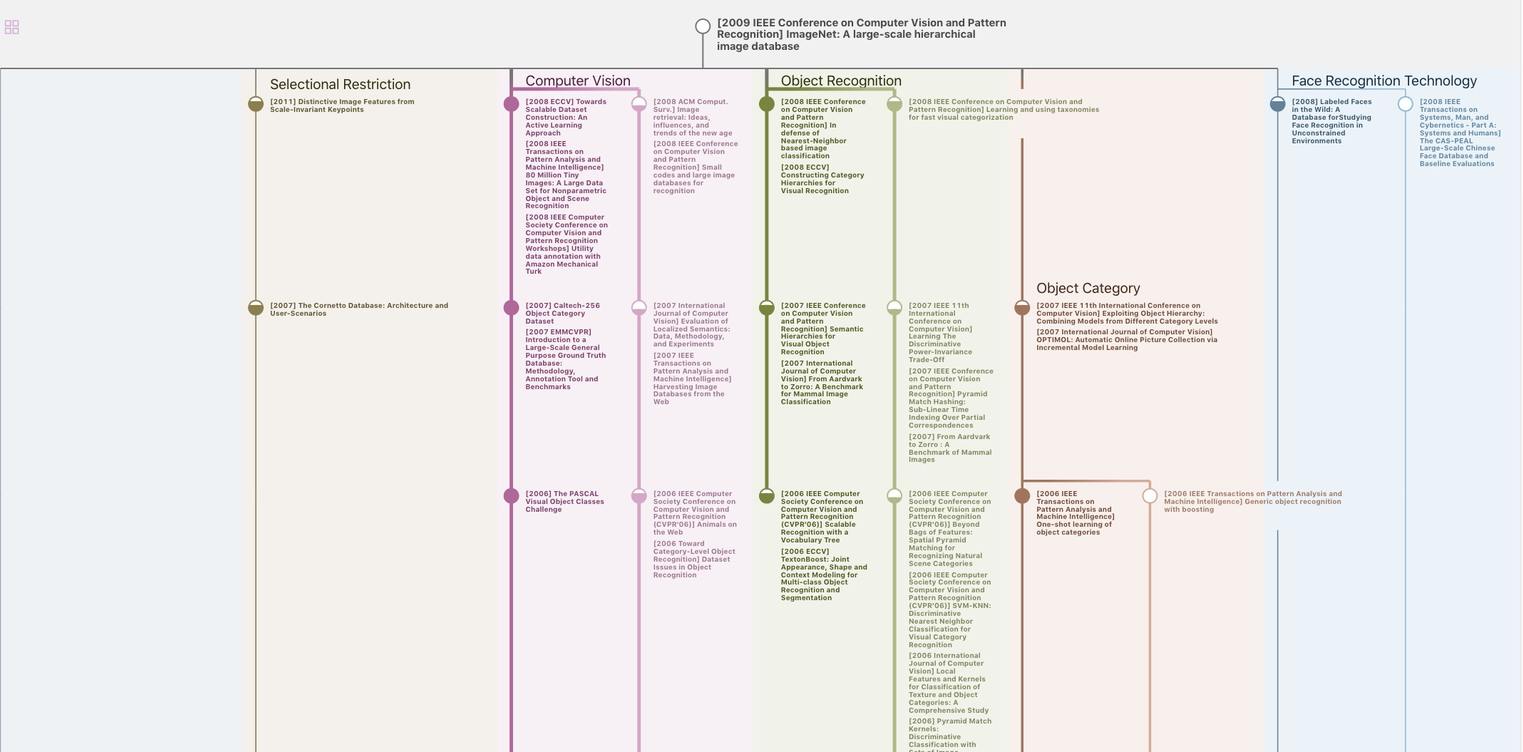
生成溯源树,研究论文发展脉络
Chat Paper
正在生成论文摘要