Optimizations of Autoencoders for Analysis and Classification of Microscopic In Situ Hybridization Images
CoRR(2023)
摘要
Currently, analysis of microscopic In Situ Hybridization images is done manually by experts. Precise evaluation and classification of such microscopic images can ease experts' work and reveal further insights about the data. In this work, we propose a deep-learning framework to detect and classify areas of microscopic images with similar levels of gene expression. The data we analyze requires an unsupervised learning model for which we employ a type of Artificial Neural Network - Deep Learning Autoencoders. The model's performance is optimized by balancing the latent layers' length and complexity and fine-tuning hyperparameters. The results are validated by adapting the mean-squared error (MSE) metric, and comparison to expert's evaluation.
更多查看译文
关键词
autoencoders,hybridization,images,classification
AI 理解论文
溯源树
样例
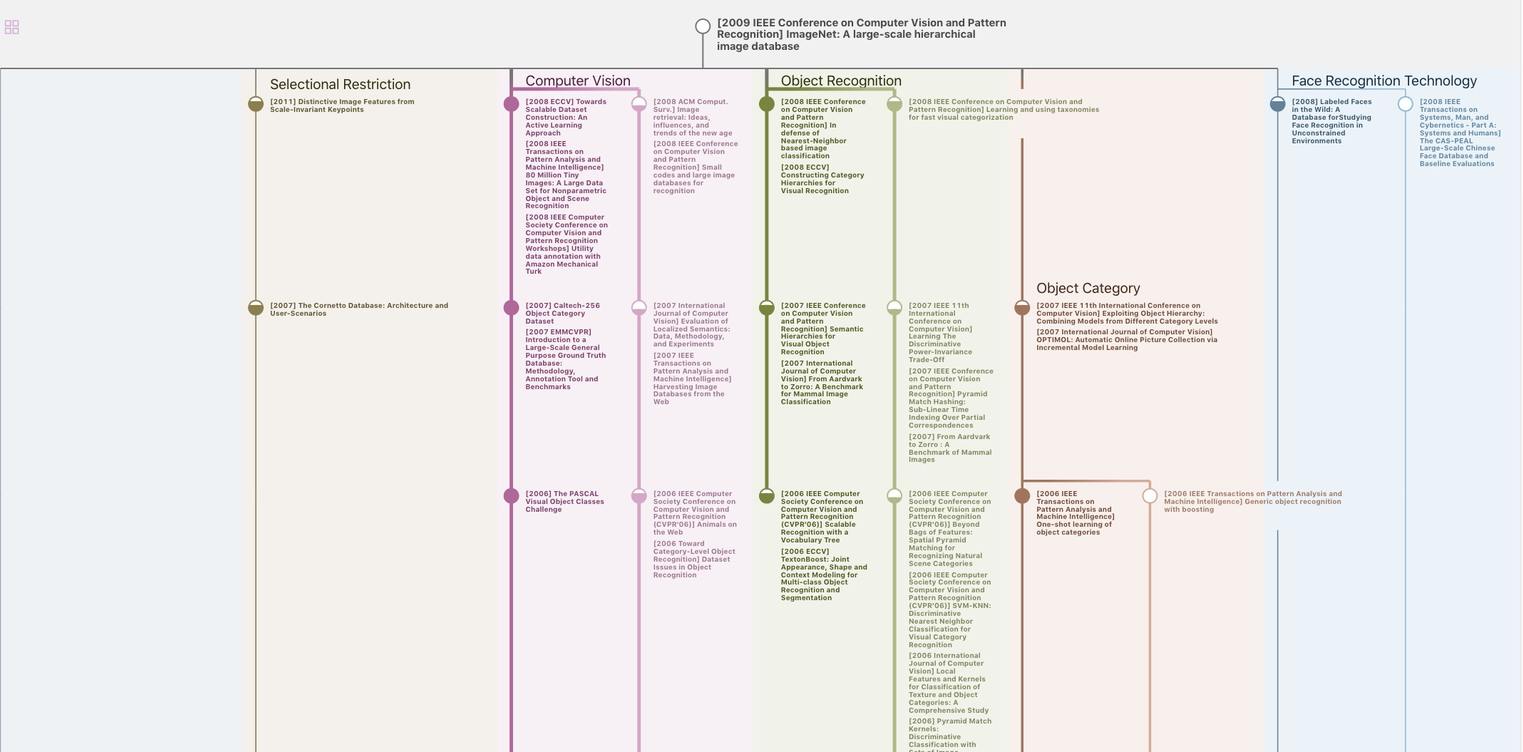
生成溯源树,研究论文发展脉络
Chat Paper
正在生成论文摘要