An Improved Framework for Reliable Cardiovascular Disease Prediction Using Hybrid Ensemble Learning
2023 International Conference on Electrical, Computer and Communication Engineering (ECCE)(2023)
摘要
Cardiovascular diseases (CVDs), which include heart disorders, are the most prevalent and significant causes of death worldwide, including Bangladesh. Blood artery problems, rhythm issues, chest pain, heart attacks, strokes, and erratic blood pressure are a few of these. In Bangladesh, cardiovascular disease is the main factor in both male and female fatalities. More than 80% of CVD deaths are caused by heart disease and strokes, which are the predominant causes. To be able to examine the effectiveness of the various models, this research article explains the underlying methods as Support vector machines (SVM), K-Nearest Neighbors (KNN), Logistic Regression (LR), Random Forest (RF), Decision Tree (DT), and XGBoost (XGB), wherein Random Forest perform better when their hyperparameters are tuned (RandomizedSearchCV). There suggested ensemble technique such as Bagging, Voting, Stacking. Additionally, it is suggested that a hybrid strategy using Bagging and stacking ensemble approaches can boost the predictability of cardiovascular disease. For this analysis of patient performance, we used a dataset from Kaggle that comprises of 70,000 unique data values. According to the experiment's findings, the proposed model had the best disease prediction accuracy, coming in at 84.03%.
更多查看译文
关键词
Cardiovascular disease,Hybrid ensemble Approach,Hyperparameter Tuning
AI 理解论文
溯源树
样例
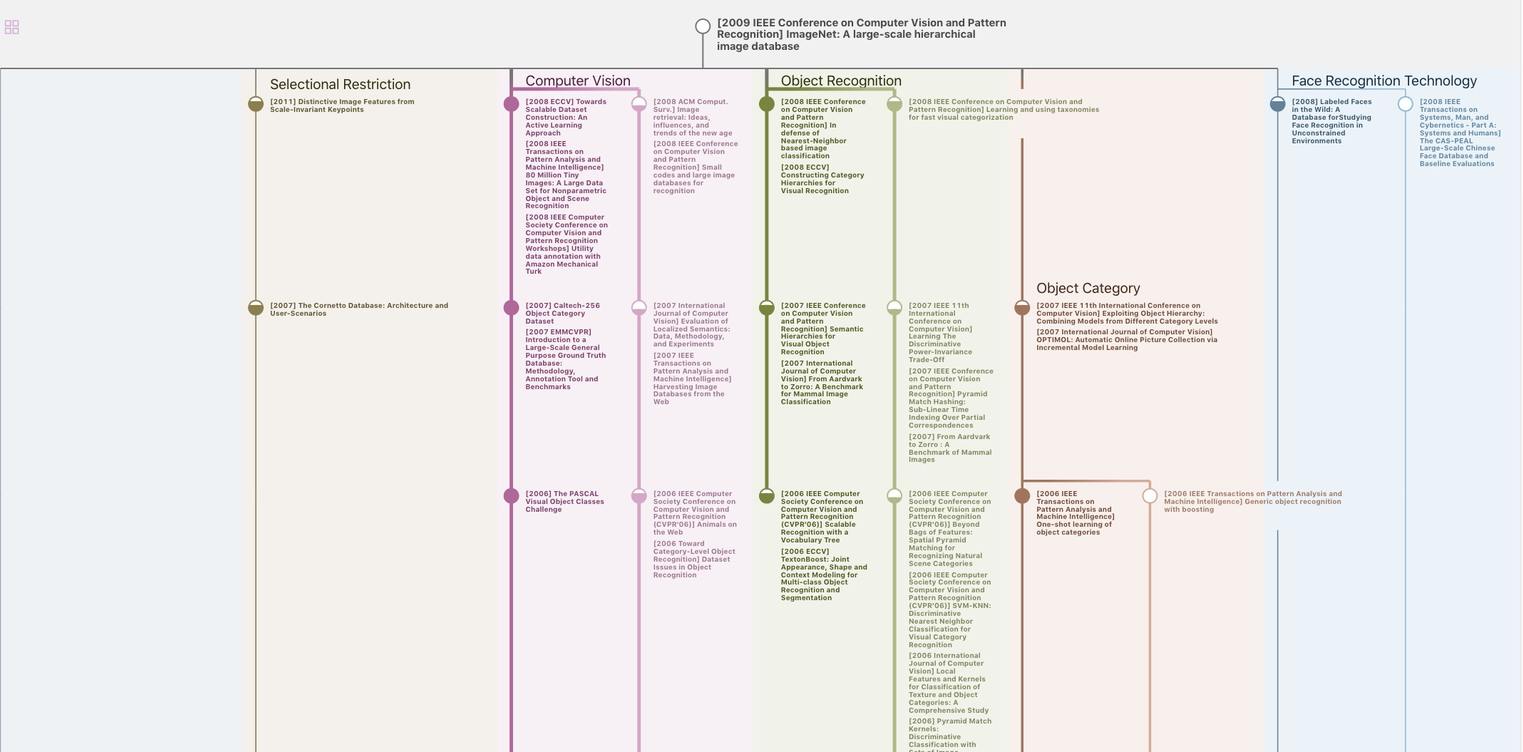
生成溯源树,研究论文发展脉络
Chat Paper
正在生成论文摘要