Towards Query-limited Adversarial Attacks on Graph Neural Networks
2022 IEEE 34th International Conference on Tools with Artificial Intelligence (ICTAI)(2022)
摘要
Graph Neural Network (GNN) is a graph representation learning approach for graph-structured data, which has witnessed a remarkable progress in the past few years. As a counterpart, the robustness of such a model has also received considerable attention. Previous studies show that the performance of a well-trained GNN can be faded by black-box adversarial examples significantly. In practice, the attacker can only query the target model with very limited counts, yet the existing methods require hundreds of thousand queries to extend attacks, leading the attacker to be exposed easily. To perform a step forward in addressing this issue, in this paper, we propose a novel attack methods, namely Graph Query-limited Attack (GQA), in which we generate adversarial examples on the surrogate model to fool the target model. Specifically, in GQA, we use contrastive learning to fit the feature extraction layers of the surrogate model in a query-free manner, which can reduce the need of queries. Furthermore, in order to utilize query results sufficiently, we obtain a series of queries with rich information by changing the input iteratively, and storing them in a buffer for recycling usage. Experiments show that GQA can decrease the accuracy of the target model by 4.8%, with only 1% edges modified and 100 queries performed.
更多查看译文
关键词
Adversarial Attack,Graph Neural Network,Graph Representation Learning
AI 理解论文
溯源树
样例
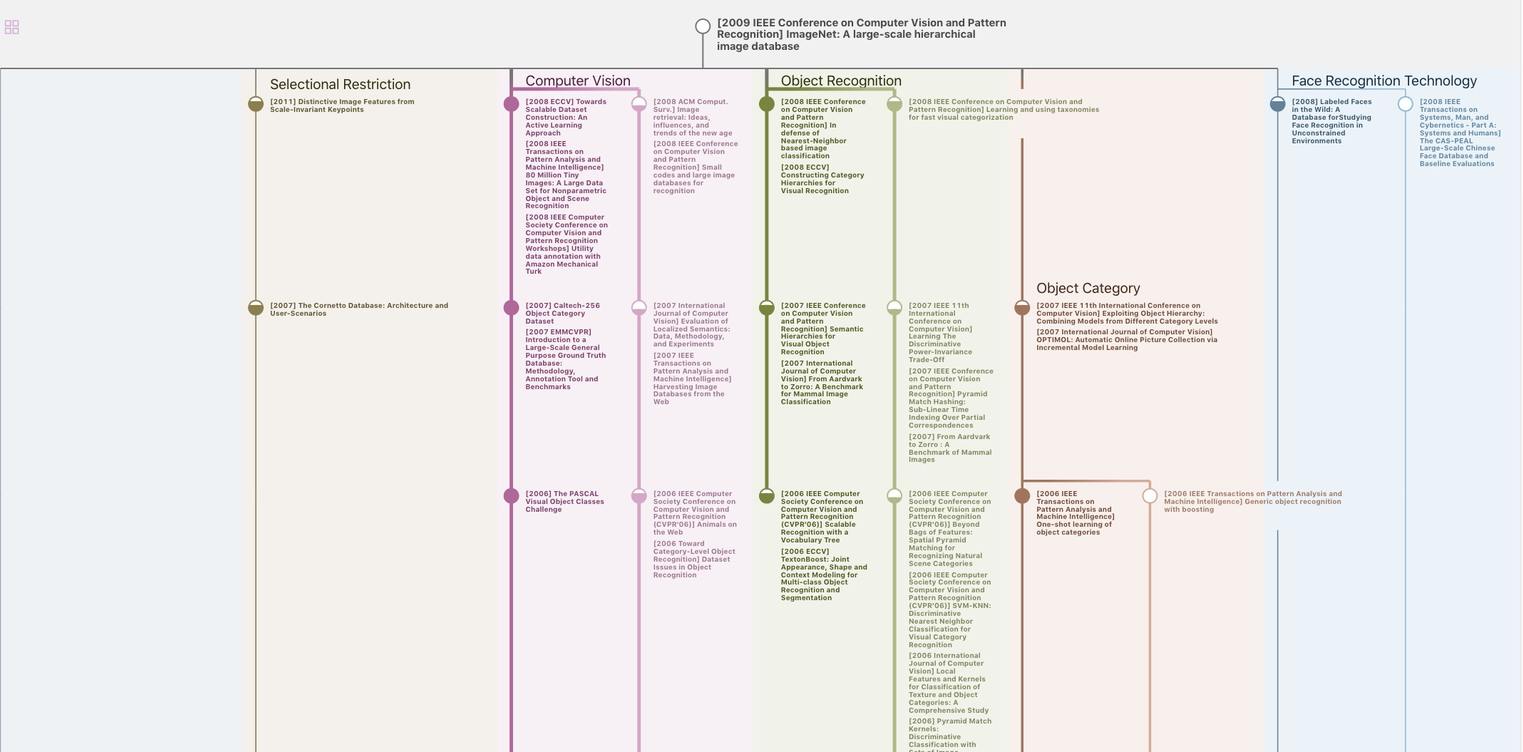
生成溯源树,研究论文发展脉络
Chat Paper
正在生成论文摘要