Skill-Oriented Hierarchical Structure for Deep Knowledge Tracing
2022 IEEE 34th International Conference on Tools with Artificial Intelligence (ICTAI)(2022)
摘要
Knowledge tracing (KT) which aims to trace stu-dents' knowledge state is an effective technique in intelligent tutoring systems. Although most KT models have exploited the question side information, plentiful hierarchical information between skills hasn't been well extracted for making more accurate predictions. In this paper, a novel model called Skill-oriented Hierarchical structure for Deep Knowledge Tracing (SHDKT) is proposed to discover the relations between questions, which are implicit in the hierarchical skill structure. SHDKT comprises three modules. First, The skill concurrency graph (SCG) is constructed by incorporating students' response infor-mation into the question-skill bipartite graph, which contains both sequence and co-occurrence relations between skills. Second, a hierarchical skill representation module (HSRM) is proposed to exploit the hierarchical information of skills based on the SCG. Finally, a question representation module (QRM) is presented by learning explicit and implicit interactions of question side infor-mation. Hence we can predict the student response accurately through question representation. Extensive experiments on the KT datasets validate the effectiveness of our model.
更多查看译文
关键词
Intelligent tutoring system,Knowledge tracing,Graph Neural Network,Hierarchical Structure Modeling
AI 理解论文
溯源树
样例
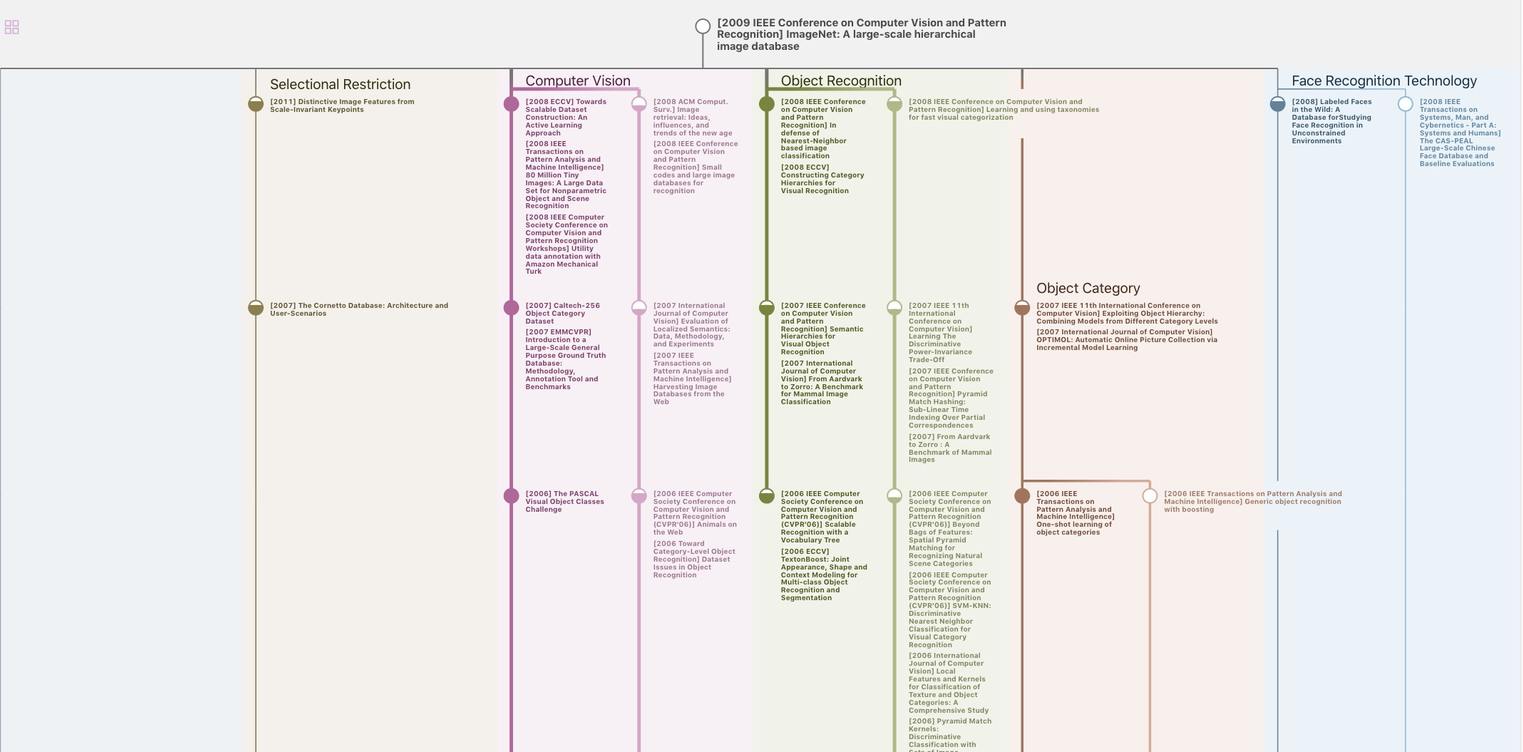
生成溯源树,研究论文发展脉络
Chat Paper
正在生成论文摘要