Enhancing Neural Aspect Term Extraction Using Part-Of-Speech and Syntactic Dependency Features
2022 IEEE 34th International Conference on Tools with Artificial Intelligence (ICTAI)(2022)
摘要
We tackle Aspect Term Extraction (ATE), an important natural language processing task that automatically identifies words of domain-specific aspects. Recently, a variety of sophisticated neural models and learning strategies have been explored for enhancing ATE, and significant improvements have been obtained. We intend to strengthen the neural models by involving features of Part-Of-Speech (POS) and syntactic dependency into the encoding process. It is motivated by the empirical findings that features of linguistic structure help to refine the understanding of semantics. Accordingly, we propose a stepwise encoding approach, where POS and syntactic dependency are successively leveraged step by step, including 1) joint encoding over both word and POS sequences using a pretrained language model; 2) BiGRU-based representational refinement conditioned on semantics-aware POS information and POS-aware semantic information; 3) representational augmentation by convolutional encoding of dependency graph. We conduct experiments on the four benchmark datasets of Semantic Evaluation (SemEval) for ATE. Experimental results show that our method obtains substantial improvements on all the considered datasets, and the performance (
$F1$
-score) reaches 87.69%, 89.76%, 77.94% and 83.96% for L-14, R-14, R-15 and R-16, respectively. All the models and source codes in the experiments will be made publicly available to support reproducible research.
更多查看译文
关键词
aspect term extraction,natural language processing,syntactic feature,sequentiality
AI 理解论文
溯源树
样例
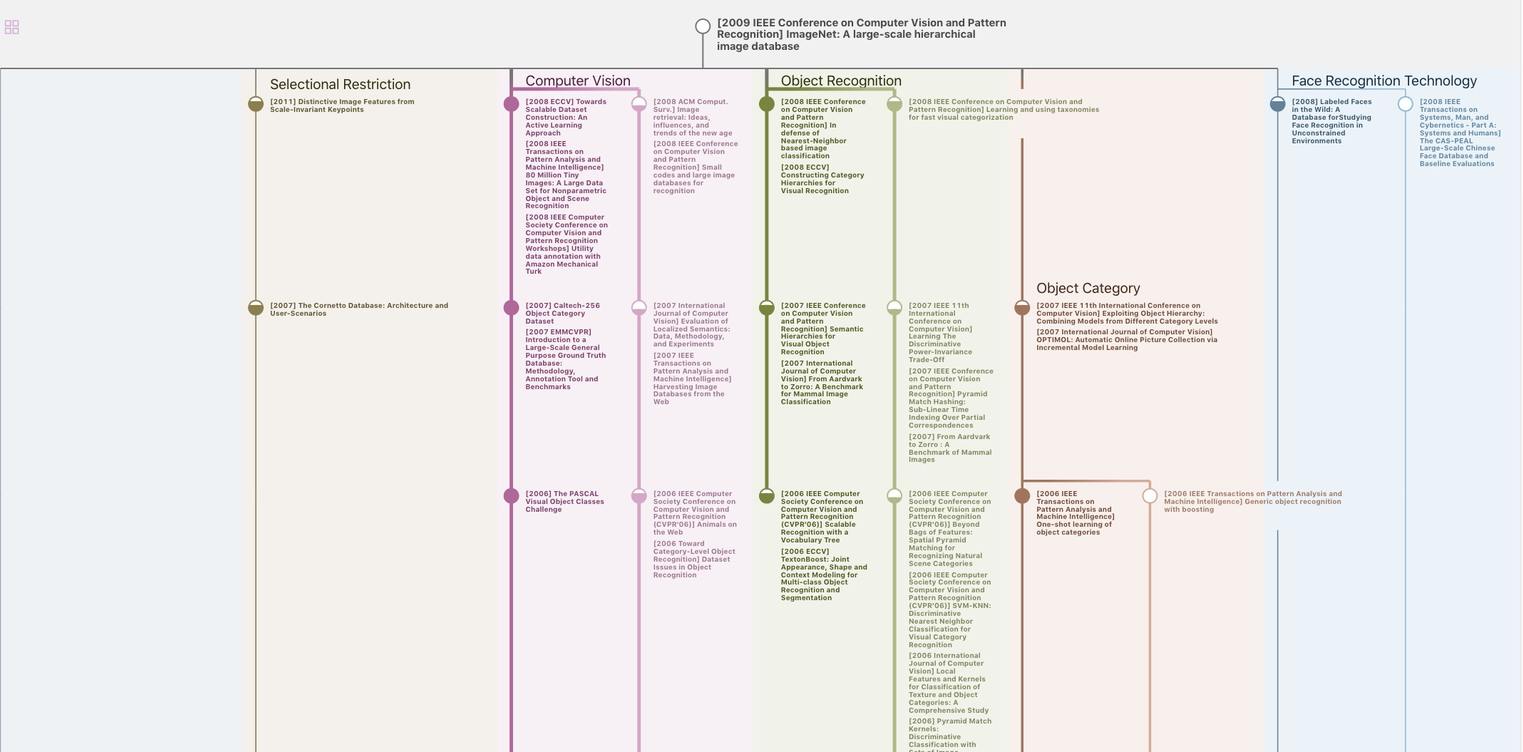
生成溯源树,研究论文发展脉络
Chat Paper
正在生成论文摘要