Towards Interpretable Feature Representation for Domain Adaptation Problem
2022 IEEE 34th International Conference on Tools with Artificial Intelligence (ICTAI)(2022)
摘要
Deep convolutional neural networks (CNNs) have witnessed a great progress in visual recognition over the past years. However, deep CNN models are still suffering from the domain adaptation problem. Most of the existing methods try to resolve this issue by creating more useful samples in the source domain for network training so that the well-trained CNN models can well adapt to more possible variations in the target domain. However, such methods are different with human visual mechanism. Human eyes can effectively recognize images with large variations that were never seen before, as long as human eyes are very familiar with partial contents of the input images. We simulate the visual mechanism of human eyes and make feature responses diverse as far as possible. We proposed a novel angular diversity loss, which contains a pair of angular Spatial Activation Diversity (A-SAD) losses by borrowing the idea of the angular losses. Besides concerning the recognition accuracy, we also focus on understanding deep CNNs. Recent works further pushed the interpretability into the training stage of the CNN models. This helps CNN models learn more meaningful feature representations. Extensive experiment on MNIST dataset and its six variation dataset show the effectiveness of the proposed A-SAD loss.
更多查看译文
关键词
Interpretable features,diversity loss,angular Softmax loss,regularization
AI 理解论文
溯源树
样例
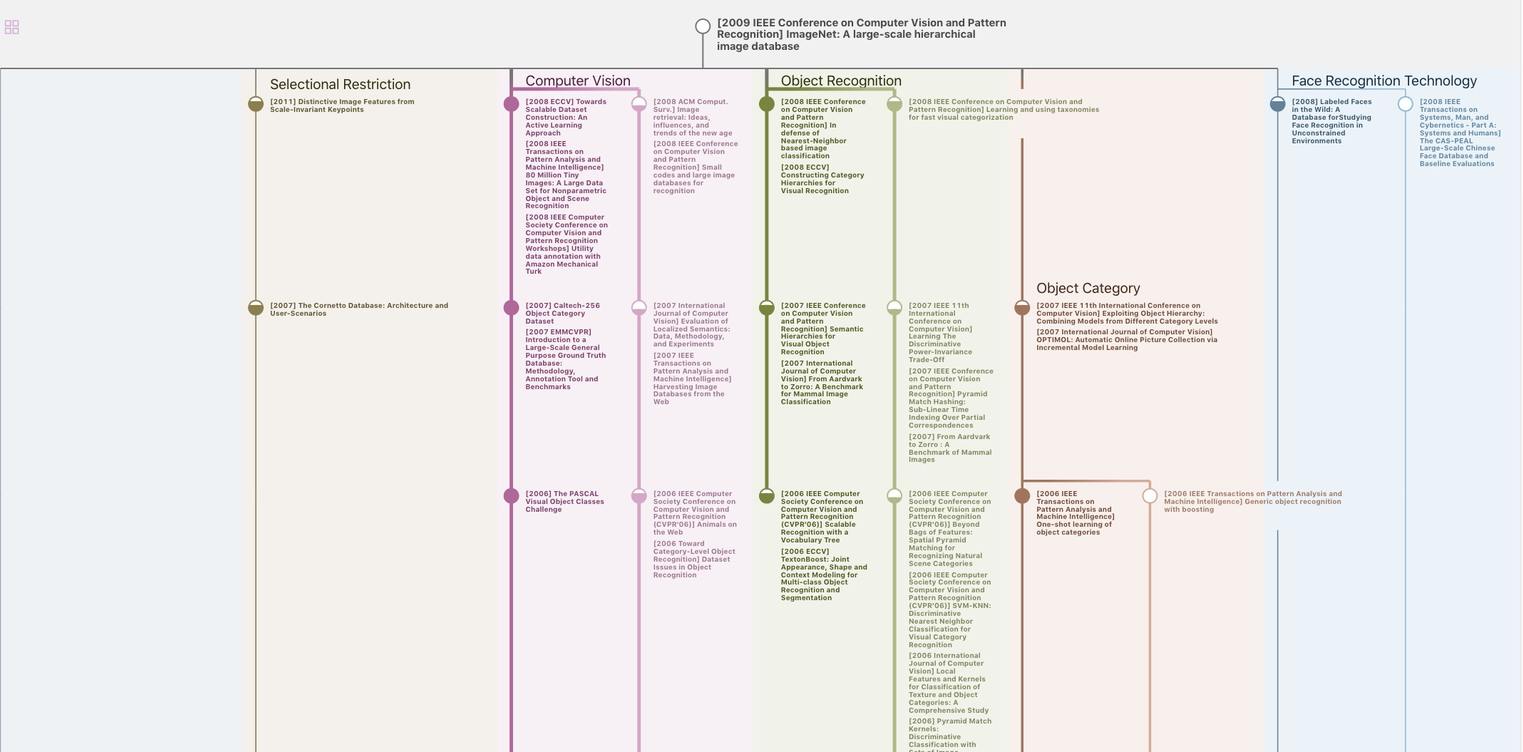
生成溯源树,研究论文发展脉络
Chat Paper
正在生成论文摘要