Transfer Learning for Regression through Adaptive Gaussian Process
2022 IEEE 34th International Conference on Tools with Artificial Intelligence (ICTAI)(2022)
摘要
Extracting knowledge from closely-related domains to solve a new problem has become an advanced methodology in machine learning, and is called transfer learning. Conspicuous among existing regression methods are those built around Gaussian process (GP) because they consider variance in the predicted values, although they also require that the similarity between the source domain and the target domain needs to fall within a certain range. To overcome this limitation in GP methods and improve transfer learning performance, this study proposes an adaptive Gaussian process (AGP) for regression. The AGP method broadens the range of acceptable similarity in current GP methods by developing a new transfer kernel. The results of experiments with transfer regression problems on both synthetic and real-world datasets indicate that this AGP method signiticantly improves prediction accuracy.
更多查看译文
关键词
transfer learning,Gaussian process,regression
AI 理解论文
溯源树
样例
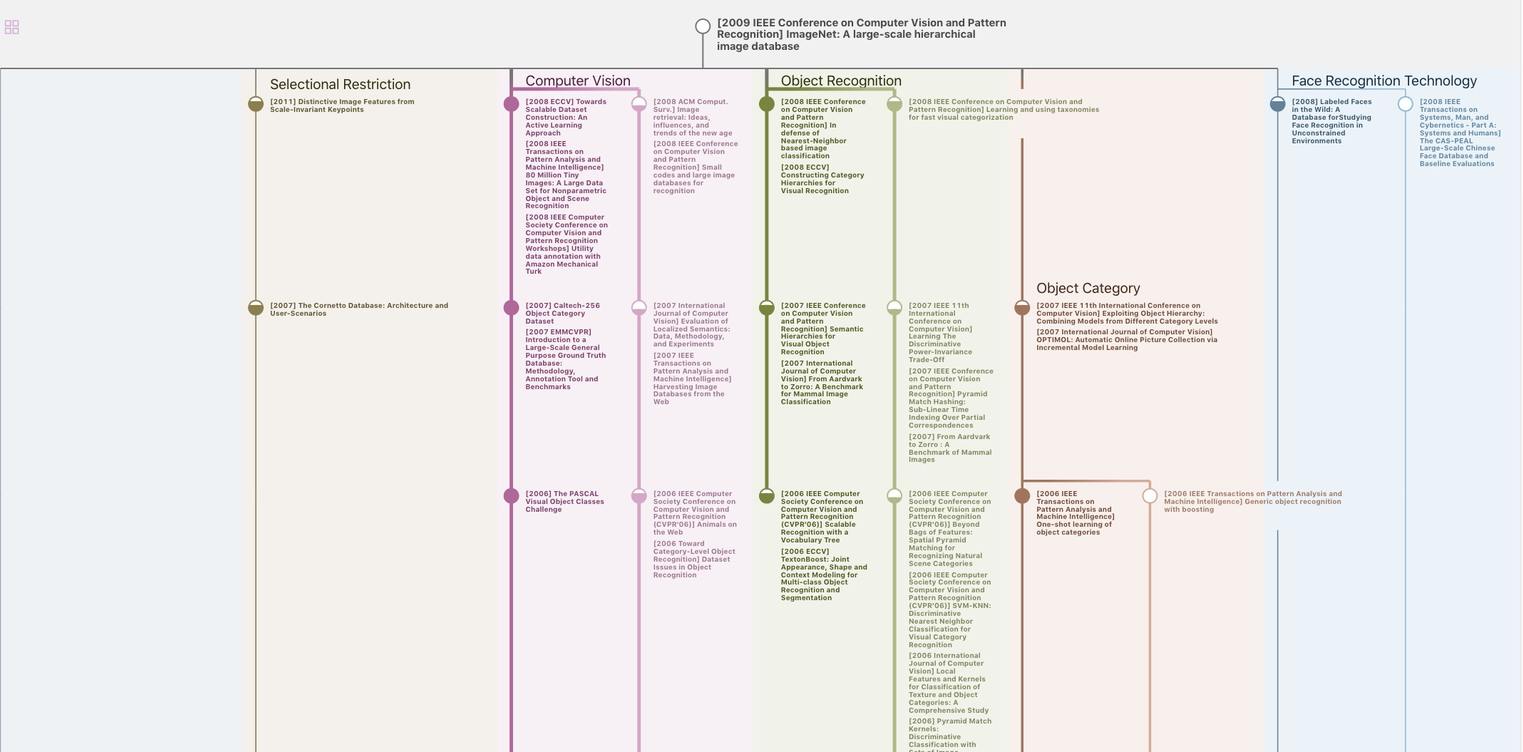
生成溯源树,研究论文发展脉络
Chat Paper
正在生成论文摘要