Quantifying and Interpreting the Prediction Accuracy of Models for the Time of a Cardiovascular Event-Moving Beyond C Statistic A Review
JAMA CARDIOLOGY(2023)
摘要
IMPORTANCE For personalized or stratified medicine, it is critical to establish a reliable and efficient prediction model for a clinical outcome of interest. The goal is to develop a parsimonious model with fewer predictors for broad future application without compromising predictability. A general approach is to construct various empirical models via individual patients' specific baseline characteristics/biomarkers and then evaluate their relative merits. When the outcome of interest is the timing of a cardiovascular event, a commonly used metric to assess the adequacy of the fitted models is based on C statistics. These measures quantify a model's ability to separate those who develop events earlier from those who develop them later or not at all (discrimination), but they do not measure how closely model estimates match observed outcomes (prediction accuracy). Metrics that provide clinically interpretable measures to quantify prediction accuracy are needed. OBSERVATIONS C statistics measure the concordance between the risk scores derived from the model and the observed event time observations. However, C statistics do not quantify the model prediction accuracy. The integrated Brier Score, which calculates the mean squared distance between the empirical cumulative event-free curve and its individual patient's counterparts, estimates the prediction accuracy, but it is not clinically intuitive. A simple alternative measure is the average distance between the observed and predicted event times over the entire study population. This metric directly quantifies the model prediction accuracy and has often been used to evaluate the goodness of fit of the assumed models in settings other than survival data. This time-scale measure is easier to interpret than the C statistics or the Brier score.CONCLUSIONS AND RELEVANCE This article enhances our understanding of the model selection/evaluation process with respect to prediction accuracy. A simple, intuitive measure for quantifying such accuracy beyond C statistics can improve the reliability and efficiency of the selected model for personalized and stratified medicine.
更多查看译文
AI 理解论文
溯源树
样例
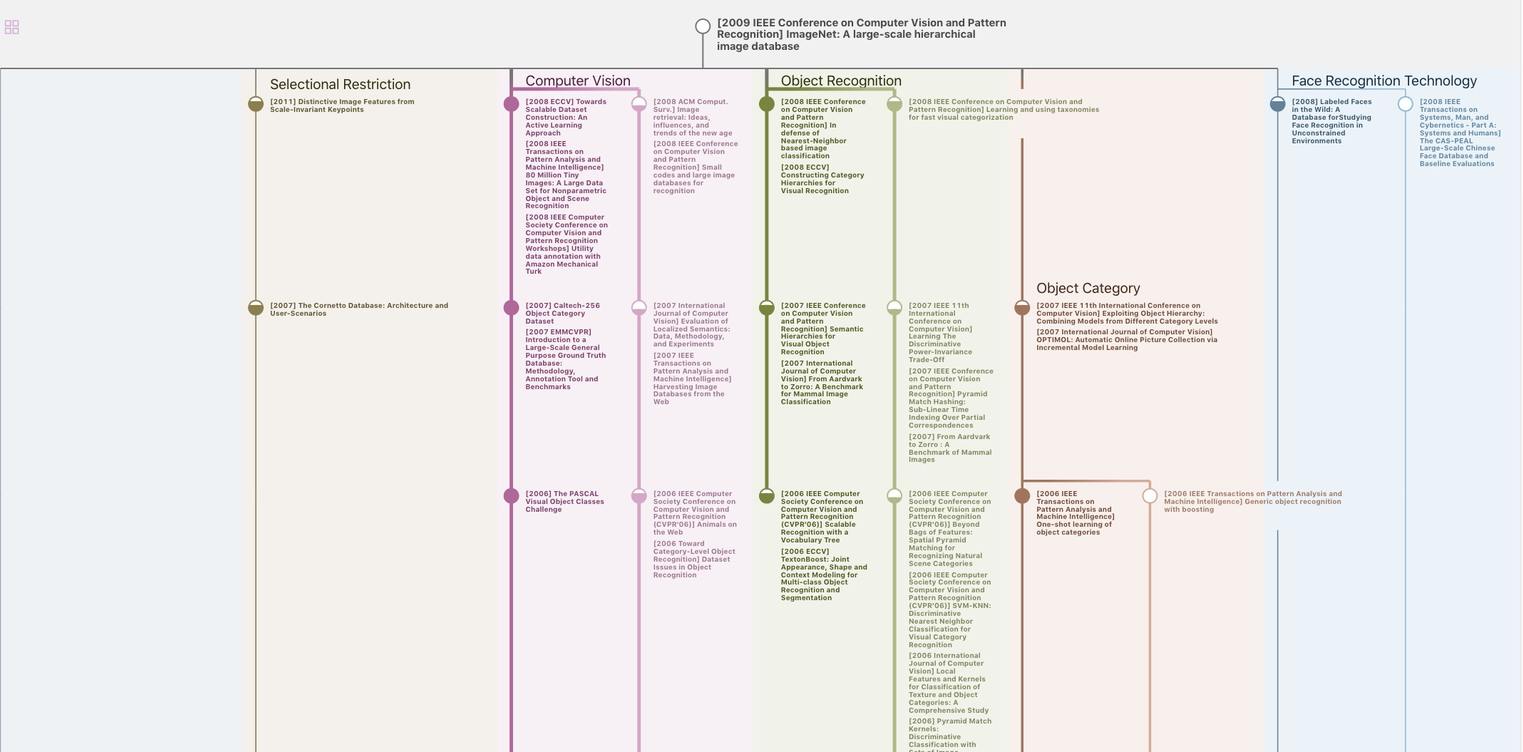
生成溯源树,研究论文发展脉络
Chat Paper
正在生成论文摘要