A 3D structure mapping-based efficient topology optimization framework
Journal of Mechanical Design(2023)
摘要
Abstract It is well known that the computational cost of classic topology optimization (TO) methods increase rapidly with the size of the design problem because of the high-dimensional numerical simulation required at each iteration. Recently, the technical route of replacing the TO process with artificial neural network (ANN) models has gained popularity. These ANN models, once trained, can rapidly produce an optimized design solution for a given design specification. However, the complex mapping relationship between design specifications and corresponding optimized structures presents challenges in the construction of neural networks with good generalizability. In this paper, we propose a new design framework that uses deep learning techniques to accelerate the TO process. Specifically, we present an efficient topology optimization (ETO) framework in which structure update at each iteration is conducted on a coarse scale and a structure mapping neural network (SMapNN) is constructed to map the updated coarse structure to its corresponding fine structure. As such, fine-scale numerical simulations are replaced by coarse-scale simulations, thereby greatly reducing the computational cost. In addition, fragmentation and padding strategies are used to improve the trainability and adaptability of SMapNN, leading to a better generalizability. The efficiency and accuracy of the proposed ETO framework are verified using both benchmark and complex design tasks. It has been shown that with the SMapNN, TO designs of millions of elements can be completed within a few minutes on a personal computer.
更多查看译文
关键词
topology optimization, convolutional neural network, machine learning, artificial intelligence, computer-aided design, conceptual design, design optimization, simulation-based design
AI 理解论文
溯源树
样例
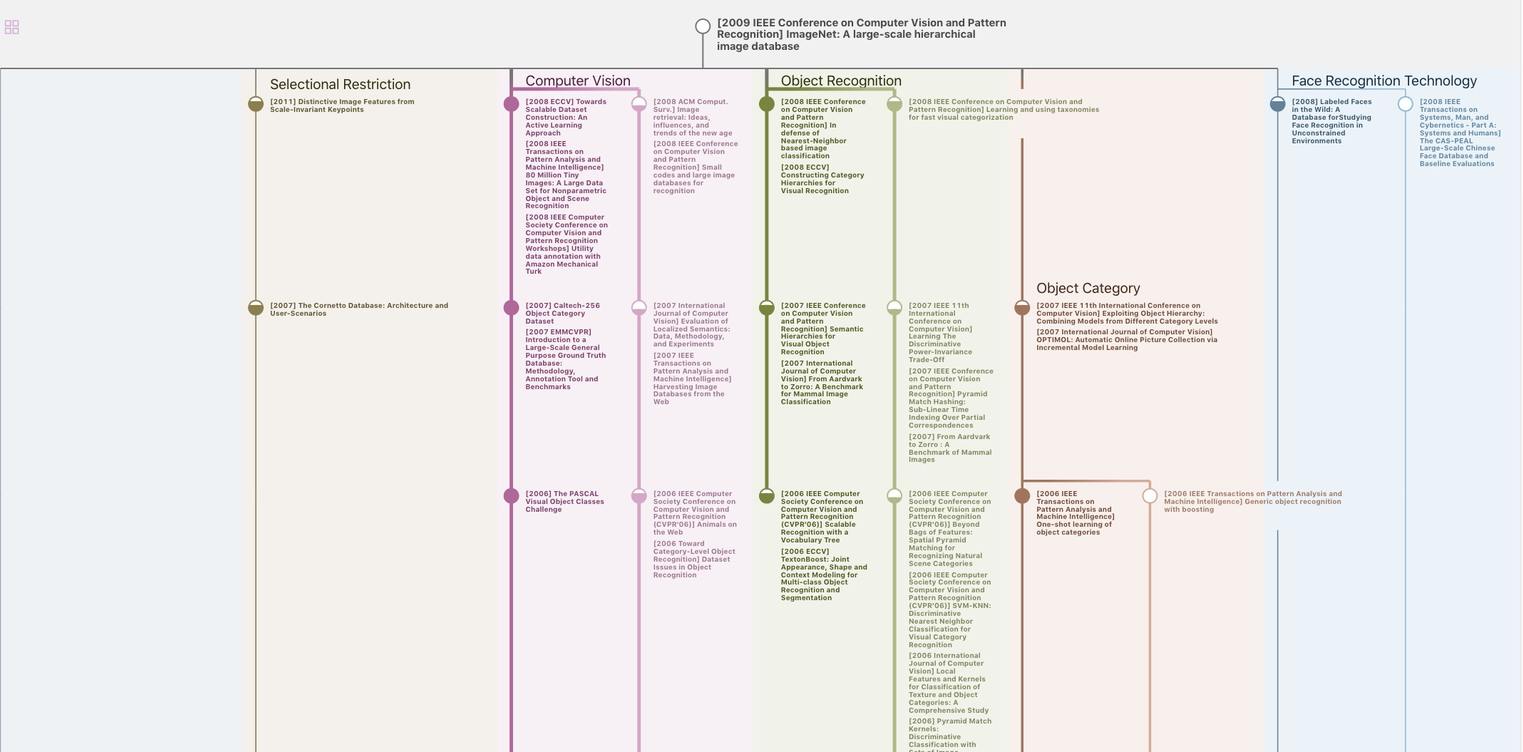
生成溯源树,研究论文发展脉络
Chat Paper
正在生成论文摘要