Semantic segmentation based on Deep learning for the detection of Cyanobacterial Harmful Algal Blooms (CyanoHABs) using synthetic images
Applied Soft Computing(2023)
摘要
Cyanobacterial Harmful Algal Blooms (CyanoHABs) in lakes and reservoirs have increased substantially in recent decades due to different environmental factors. Its early detection is a crucial issue to minimize health effects, particularly in potential drinking and recreational water bodies. The use of Autonomous Surface Vehicles (ASVs) equipped with machine vision systems (cameras) onboard, represents a useful alternative at this time. In this regard, we propose an image Semantic Segmentation approach based on Deep Learning with Convolutional Neural Networks (CNNs) for the early detection of CyanoHABs considering an ASV perspective. The use of these models is justified by the fact that with their convolutional architecture, it is possible to capture both, spectral and textural information considering the context of a pixel and its neighbors. To train these models it is necessary to have data, but the acquisition of real images is a difficult task, due to the capricious appearance of the algae on water surfaces sporadically and intermittently over time and after long periods of time, requiring even years and the permanent installation of the image capture system. This justifies the generation of synthetic data so that sufficiently trained models are required to detect CyanoHABs patches when they emerge on the water surface. The data generation for training and the use of the semantic segmentation models to capture contextual information determine the need for the proposal, as well as its novelty and contribution.
更多查看译文
关键词
cyanobacterial harmful algal blooms,semantic segmentation,deep learning,cyanohabs,detection
AI 理解论文
溯源树
样例
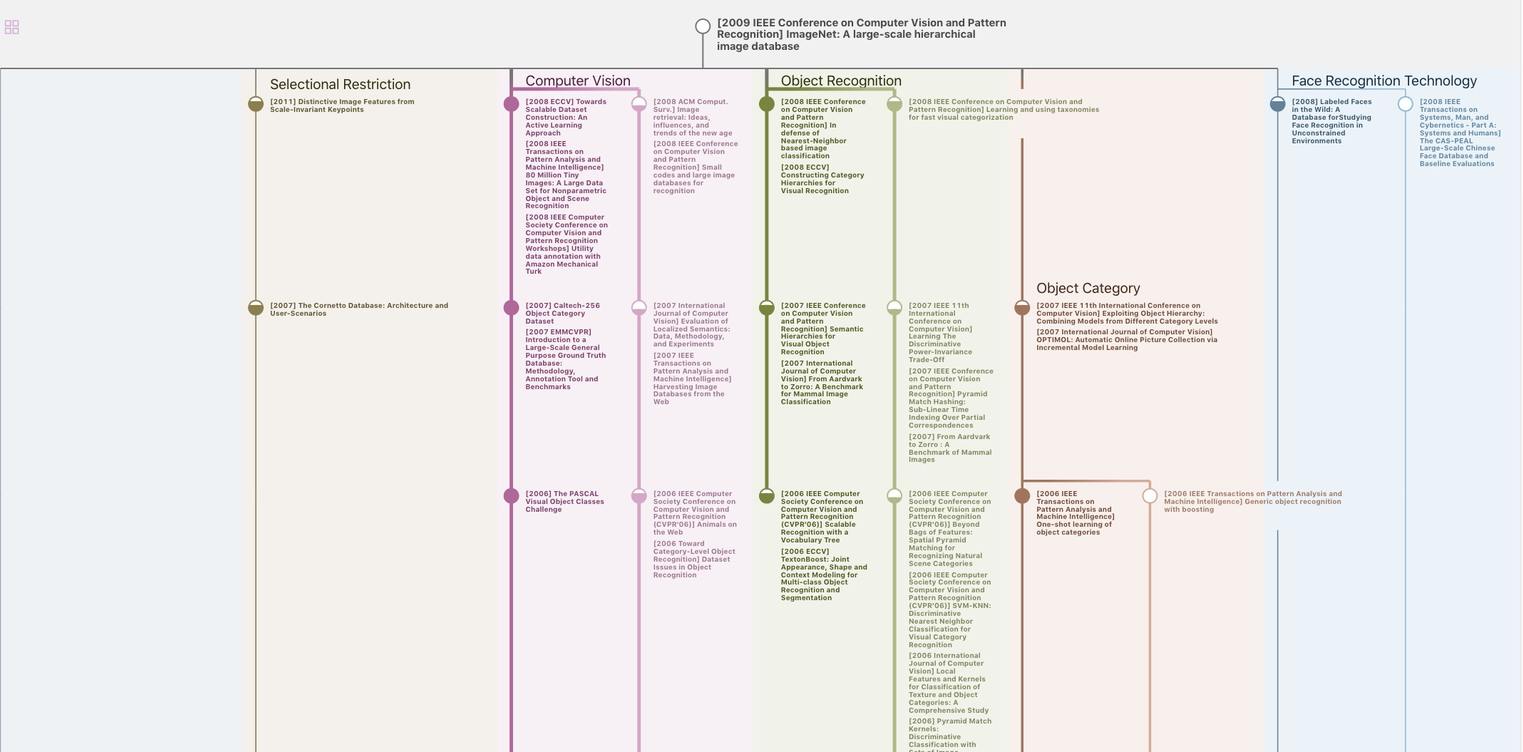
生成溯源树,研究论文发展脉络
Chat Paper
正在生成论文摘要